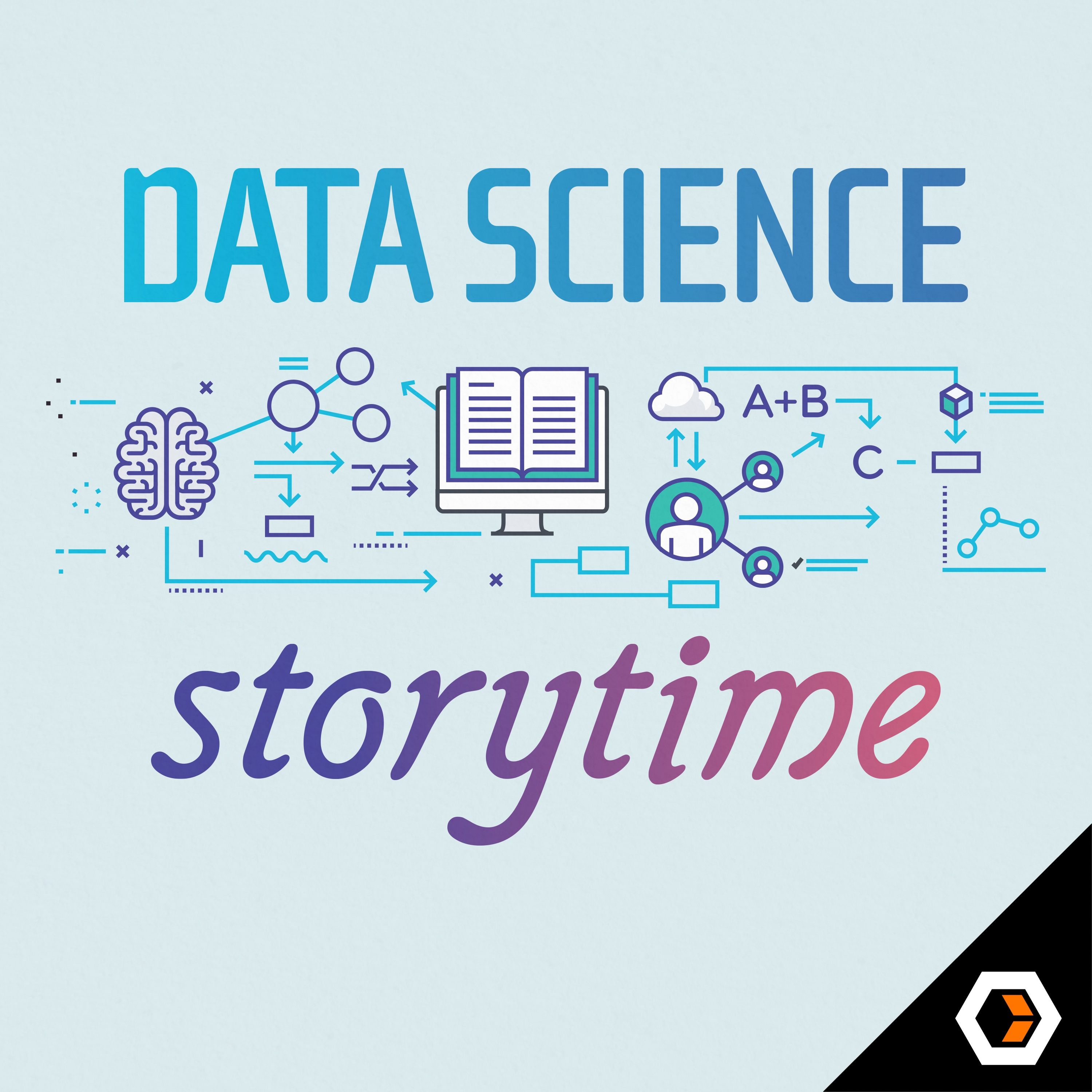
Ep. #3, The Future of Data
In the latest Data Science Storytime, Kyle and Kevin imagine what the future will look like where more companies take a cue from Google and Facebook and start counting & measuring everything. Hear the pair discuss why there is so much potential in companies like Tesla and why all the deep learning buzz might not be all it’s cracked up to be.
In the latest Data Science Storytime, Kyle and Kevin imagine what the future will look like where more companies take a cue from Google and Facebook and start counting & measuring everything. Hear the pair discuss why there is so much potential in companies like Tesla and why all the deep learning buzz might not be all it’s cracked up to be.
transcript
Kevin Wofsy: Okay, we're back.
Kyle Wild: And we're back.
Kevin: And we're back. I forget how we start these things.
Kyle: Well, we don't have it. There's no "we starting these things" yet. I don't think we have a cadence, or even know what the thing is. What are you going to name it and all that? Let's not go there, though.
Kevin: No, I know.
Kyle: And spend an hour on that.
Kevin: I know, I know. We already did. It's our best episode. It's our best episode, is coming up with a name that we might now abandon. I do like ours now.
We can just get a running start, and then whenever is the right time to start, we'll start. But I know that you just got back from New York where you were making an announcement with IBM about a partnership for Keen with the Watson project.
Kyle: Yep.
Kevin: And so it got me thinking about all the things in your mind about the future: where you see the future of Keen, where you see the future of analytics, why it's exciting. And since we weren't there in the room with you then, I'm just curious, when you picked your "10 years into the future," what do you imagine? What do you imagine for the company? What do you imagine for the landscape of analytics?
Kyle: First, to answer that question we have to dig a little bit into what analytics, what we mean by that. Even you asking the question to me, even those colleagues for years, we may mean different things.
Kevin: That's great. Okay, let's dig.
Kyle: Imagine a big Venn diagram. There's a huge circle on the outside called "science," right? This is like the scientific method. This is a rigorous intellectual practice for groups of people to find the truth, or at least systematically find things that aren't truth. That's a little pedantic.
Within that, there's a whole bunch of stuff like, how do you form hypothesis? It's pretty intuitive, pretty human. There's some interesting work in AI to try to get machines to get better at that, but I'm not betting on that stuff hitting in 10 years. I think human insight and intuition is going to be pretty important for forming hypotheses and designing experiments.
But how to run those experiments and collect the data as a result of those experiments and how to evaluate whether your hypothesis is true or false, is actually the hard part for a lot of companies, for a lot of universities.
If you go to elite university science labs today, you'll see people writing on notebooks. You'll see people filling out old spreadsheet software, not even Excel. And that stuff, they develop master of this weird archaic software. It's the kinds of computers they have, and they store that stuff on that machine. They run their analysis, they write their paper, and the data is gone. It's usually small data. It's usually super well-structured, and it usually disappears.
So that's the state of science, like the actual science, like let's say, conservatively, tens of billions, or maybe hundreds of billions of dollars of enterprise in the human pursuit of knowledge. That's the state of how it's done today.
Kevin: Small, disconnected, disposable data.
Kyle: Small, disconnected, disposable, and we keep the unstructured paper that somebody writes. You can sometimes get to the underlying data. Practices are getting better. It's been 10 years since I was in college, when I saw a lot of the stuff up close, but suffice it to say, it's still like that.
I think in the business world, usually the business world's ahead on tools, sometimes behind on vision compared to academia. In the business world, the tools are not that different. So anyway, that's science-science, like the scientific method.
Kevin: Okay.
Kyle: The data science piece, I talked about something that's kind of archaic. Let's talk about something that's really at the cutting edge. What kind of company do you think is on the cutting edge right now?
Kevin: What kind of company do I think is on the cutting edge?
Kyle: Yeah, what are some companies that are probably really good at this stuff?
Kevin: I would think Google would be pretty good at that stuff.
Kyle: Yeah, Google's pretty damn good at this stuff. Facebook's pretty good at this stuff. LinkedIn and Amazon. Since LinkedIn is part of Microsoft, we're talking about four of the Fortune top six companies, and they're really good at this stuff, and
the way they do this stuff is, all the data is collected at very large scale. It's never thrown away. It's super connectable.
At least within their enterprise, people can get to the data, and it's very, very large. So it's the opposite of all the things we just described. The reason that I'm doing what I'm doing, one of the reasons we exist as a company is, what about all the companies that aren't in the Fortune six? Right?
Kevin: Wait, we're not in the Fortune six?
Kyle: We're not in the Fortune six, certainly, and none of our customers are, yet. So what about everyone else, right? To make it more concrete, some of the things that these companies are really good at, when Facebook rolls out a new feature, they roll it out to a tiny, tiny sample of people, small enough that if the feature doesn't perform well, they can destroy it and nobody ever knows it existed.
It's small enough a sample that doesn't even get in the press, and they do this kind of stuff quietly and secretly all the time. And they collect everything about what you do on their product. They don't need focus groups. Focus groups are a summary of user activity. They actually just have all the user activity.
They don't need to focus the data. They can get all of it.
And they do this stuff constantly and in real time, and every feature that they want should have hypothesis around it. And they have success criteria, so everything they do is scientific. As a product design company, largely, that's what they do.
Obviously, on the ad side, they're very scientific about that stuff, too. The interesting thing to me is that a lot of companies, really sophisticated and cutting-edge companies, just haven't figured this stuff out. And I think it's because the tools suck. So, in 10 years, I'd like to see a world where maybe there are 20,000 companies whose capacity, in 10 years, is worth Facebook's today.
Kevin: Well, if the tools suck, why do Google and Facebook have so much better tools?
Kyle: They build it, and they have very smart people. One of their biggest advantages is that they're new companies, so they're not tied to stuff that they bought in 1995 that didn't exist in 1995. One of the reasons Facebook is significantly better at this than Google, is that Facebook didn't exist when Google built a bunch of this stuff.
The newness alone gives them an advantage.
If you're looking at a solution set or toolset as a business, you have to buy, you'd have to build it, or you'd have to partner with somebody that has it. Buying and partnering weren't options, so they built. Every company in their generation built. These companies just built really well, and I think it's one of the reasons they're still around.
Kevin: Okay, so why can't every company just do that?
Kyle: Well, to build, you'd have to have the talent. You have to be able to find data scientists, data engineers who actually know how to do this stuff, and those are currently the two hardest things to hire in the entire tech industry. So, the solution of building out a team of the kinds of people that built these systems at those companies will not work, because you can't actually find those people.
If I imagine that, 10 years from now, there are 20,000 companies who have the data science capacity that Facebook and Google have in 2016, they have now, I'm not saying that those 20,000 companies will have whatever the next Facebook has then, but for them to get there, they're not going to be 20,000 companies that go out and hire a bunch of badass data engineers to build this stuff. Because there is just not enough talent on the market.
The main thing I think about is, how do you take people who are in the rest of the market who're stuck way in the past, and how do you bring them into, not as far in the past, and then how do you bring them into the present?
Eventually if you can bring them into the present, if you can get yourself into a situation where a retail chain, a Safeway, has the kind of data science capacity of a Facebook, then maybe those businesses will continue to exist in 20 years. They won't be crushed.
Kevin: So, just to be clear, where does Keen fit into this landscape now? And before we get into the future, how do we fit in? What is our role right now?
Kyle: Well, right now, we help about 3,000 of those companies, closer and closer to 4,000 having some of the same kinds of tooling.
Kevin: How?
Kyle: They can record history, write hypotheses, testing, and experiments across that historical data, and they can represent it wherever they want to represent it.
What we do makes it so that one developer can do what used to take a team of data engineers and data scientists.
As one developer using an API, one developer can do all of the telephony for the Uber ecosystem, because they use Twilio's API.
Kevin: Okay.
Kyle: One developer can do what a data team used to do. I think there are enough developers in the world to make that work. Obviously I think that. It's a part of our thesis. We'll see if it's true. One of the things that's really illuminating to me was at our conference when Jeff Norris from NASA came and gave that talk.
Kevin: Yep, I remember that.
Kyle: Because, talk about a group that's come on the cutting edge of science. And it's NASA, right? They're pretty badass.
Kevin: I'm sure lots of companies have explored outer space and gone to the moon.
Kyle: Yeah, in their universe. He used our platform on a Sunday to find a bug that was over a decade old instead of the software that's on every robot that they launch, every crawler, every rover, right?
Kevin: That they hadn't found otherwise.
Kyle: That they hadn't found for those 10 years, right? And this wasn't even new data. This wasn't a live, real-time data stream. This wasn't any of that whizz-bang, IoT device connected to the cloud every second of everyday kind of stuff. The dataset I think was from 2006 that he used to find this problem.
But just by loading it into our platform and playing around with it for like an afternoon on a weekend, he found that thing. This is the head of software at the JPL. And he's a very smart guy, probably the smartest person I've ever met.
Kevin: A very nice guy too.
Kyle: Great guy, awesome speaker. We should have him on this podcast. If he can do that at NASA with his huge rocket brain, just by having slightly better tools, or maybe significantly better tools, I won't be humbled, significantly better tools. It just tells him that there is a lot of opportunity for everyone, basically, that has scientific questions. And to me, it doesn't matter what you do. You probably ought to have scientific questions about it. That's kind of my bias.
Kevin: So it sounds like what you're saying is, in the present moment, we're at an inflection point where there are a small handful of organizations that are companies, not even NASA or universities, that have the strongest power for computing and for running this kind of analysis. And the playing field, we're starting to see that get leveled with the ability for an individual developer to get what would normally take a team.
Kyle: Yeah, there's the computing power component to do this stuff. It's like a two-part system, right? You've got to have the brainpower in the company to ask the right questions. I don't know exactly how to help companies with that overall. I guess maybe some consulting firms can help with that. In general, I think the market's going to decide.
In general, the market's going to decide companies with more brainpower should win.
You've got to have the brainpower to ask the right questions, and you've got to actually have the technology to answer those questions. And I think what we're finding is that a lot of people have the brainpower and don't have the technology.
I like that example, because like I said, Jeff Norris is like the smartest guy in the world, and it's NASA. Nobody would criticize NASA for brainpower. They have enormous brainpower. But they don't have the technology, so you have to have both.
Kevin: But you don't necessarily, anymore, have to have size. You don't have to be the biggest elephant in the room in order to able to participate in this.
Kyle: Right. I've finished school about 10 years ago. I started my job at Google. Google 10 years ago was almost certainly the best in the world with this stuff. And one of the interesting things, to me when I left, was that the toolsets they have there, if more companies had those toolsets, everybody's got these kinds of questions. To me, a new startup, any kind of scientifically-minded small business owner...
My dad was a small business owner. He's a mechanic. He had an auto garage. He was a smart guy, and he had all kinds of interesting scientific questions and approaches to his business, right?
This is kind of part of how businesses are like, "Okay, how many pounds of sheet metal and how many pipes of a given length do I need to buy? And how is it moving through my system? What's my error rate when I bend pipes for mufflers," or whatever.
Kevin: It reminds me of word problems.
Kyle: Yeah, exactly. It is, right? Those algebra word problems in school are probably the best business operations lesson you can have if you get really good at those. But the interesting thing is how many people I talk to in business who have those questions, and they're like, "Yeah, I really wish I knew the answer to that."
They have this helplessness because their toolset doesn't give them those answers. That's the problem we're trying to solve. I don't know how to solve the other problem, getting people to be smart.
But getting smart people who are currently frustrated by their tools to have better tools, that's something I think we can help with.
So that's what we do now, in 10 years. Even with the products that we have today, I expect that impact to be a lot bigger, obviously.
Kevin: How so? What kind of questions will people be asking, though? What will the implementations be like, do you think?
Kyle: A good example, I always think about, I think it's probably what a lot of people think while they're driving. Because you get stuck in traffic and you think. A good example of this is, what will happen when we know the carbon emission's impact of carpooling, to the dollar? And when we know the cost of routing people in different directions on a road?
What would happen if all of the data from Tesla, from Uber, and from Google Maps, and Waze was under one roof? What could you do with that dataset? I can spitball all day on that. What could you do academically if you had that dataset?
I think you can make a case to governments that's in very clear financial terms, not kind of theoretically, but like, "Here is what we see on the ground and here is how much pollution or traffic congestion." The economy slippage whenever you have too much traffic congestion is fairly well mapped by economists.
We will save by making a certain kind of investment in the infrastructure. San Francisco doesn't have a highway to get from the south to the north. That's insane. I think with good data, we might be able to demonstrate why that would make sense.
Kevin: Tricky political situation there too.
Kyle: Oh well.
Kevin: What about the trees? What about the trees?
Kyle: No comment. I'm not a native San Franciscan. I haven't been slowly brought to a boil. I just got here, and it was already boiling. And I was like, "Hey, everybody, it's boiling." People are like, "Oh no, the trees! The skyline is great!" Yeah, I don't want to look at it for two hours. Traffic costs the city.
I'm not suggesting that if we had this information, it forces us to make certain decisions, but
I think making any decision without maximal information is just stupid, and we shouldn't be doing that ever.
And I'm not the only one saying this. Everybody's saying this. Everybody's saying that, in theory, when we have all of the "data" we'll be able to do everything, right? Like, omniscience is the same thing as omnipotence in a way. Maybe if that's true, that could all be true in the very abstract, philosophical way, like, Descartes talked about this.
The very real sense in 2016, we don't have the tools to do that. Even if we had the political tools to do that, even if we had the connectivity tools to do that, we don't have the data science tools to do that. A few companies do, and they keep it to themselves.
Kevin: I learned word problems in math class, but in English class I learned about Big Brother. Why isn't this a little Big Brother-y when we have all the data all in one place?
Kyle: I think it very well could be. I think right now what we have is, the companies that have all the data pretty much are ad companies and Palantir customers. Palantir is one of the companies that has some really core, awesome technology around the data science and analytics, and they sell it as opposed to the ad tech companies.
I put Google and Facebook in their ad tech companies. They have a lot of data about stuff, and so do Palantir's customers who are three-letter government agencies and big-five banks. So, today we've got people selling ads, three-letter government agencies, and big-five banks. They have the data.
When I think about the kind of Big Brother scenario, how do you fight that kind of centralization of data power? How do you not allow a central omniscient entity to rule everything? It's not to stop him from becoming omniscient. You can't. It's to arm up and make everybody else omniscient.
Kevin: Okay.
Kyle: It's to democratize.
You can't disarm the data overlords. You can just arm everyone else.
And the more data that regular people have access to, the more data that regular businesses have access to, the more data that universities have to access to...
Kevin: So, if you want to build your freeway, and I have a bunch of tree data...
Kyle: Bring the tree data.
Kevin: Then I can fight you, your freeway data with my tree data.
Kyle: Yeah, trees and parks. Yeah. Sunset Boulevard is not the most beautiful road in the world. It could be replaced by a highway, I'm just saying.
But yeah, that's kind of the point. I think a lot of people are afraid of this Big Brother thing, so they just try to fight data collection like, "Let's not do data collection." But the thing is you're not going to be able to do that.
Kevin: No, people are also afraid of data collection for the reason that you have an agenda. You want this freeway where Sunset Boulevard is, and if you have the data, maybe you can tweak the data to make it look like you ought to have your freeway.
Kyle: Oh yeah.
Kevin: So what you're saying is, "If I want my trees, I should arm up and everybody better kind of get in this data game, otherwise you're going to get crushed."
Kyle: Yeah.
Kevin: Okay.
Kyle: It's a practical view. We can debate about whether that's how the world maybe ought to be, or, "Is the world more beautiful that way or not?" But that's the way the world is, and that's the way the world's growing. So my view on it is, arm everyone else.
A good example is one of the things that is coming up a little bit more over the last couple of years, and especially in the last year, is the idea of where the data sits. How subpoena-ble is a given data vendor, or a data owner.
There's some leak I saw this week. I don't know if it's a leak, something, may or may not be true, I don't know. It's not on Twitter. I didn't even put the link. It said something about Yahoo secretly wrote email sniffers to search for certain kinds of activity for the U.S. government through a billion email accounts or something.
Kevin: Right.
Kyle: Well, and we wonder why people want their own private emails server sometimes.
Kevin: What person could you be talking about?
Kyle: All kinds of people. My brother has a private email server. All kinds of people. I don't know what you're talking about. I think the reality is that that's going to happen so one of the things that we're exploring at Keen, is Swiss data center, which to me is going to be the future of the Swiss bank.
People used to hide their money in Switzerland, now they're going to hide their data in Switzerland.
Because it's maybe less likely that people will use the court system to force private enterprises to secretly disclose data to an entity. It could be a Big Brother-like entity. That's something that's coming now. This is the stuff that's happening right now. I don't know, I've got all kinds of hopes and thoughts on what will happen politically, but that's not what I do. What I do is run a private company.
Kevin: Exactly.
Kyle: What we can do is make it so that our customers own their data instead of us owning it, and make it so that businesses of different kinds have access to this kind of stuff. And one of the ways that people use us, for instance, is we're growing traction in Germany. Because we don't force certain kinds of data collection.
If you use Google Analytics, you can record this stocks stuff all the time, because everything we just developed are configurable, and because in Germany, there are certain kinds of data. Germany has some of the most advanced data privacy rights for individual citizens.
Companies in Germany can use us and specifically not collect certain kinds of things that you're not allowed to collect, which I think is awesome. I think that's a really good example of our technology helping avoid some of the Big Brother-scenario stuff, and I'm sure there are people using us in other places where they're using us to record all kinds of stuff. The liability is on them.
Kevin: Yeah, I didn't even mean to take it so much down this dark pathway.
Kyle: You just said the words "Big Brother"...
Kevin: Because I just self-confess here. I'm an old San Francisco hippie at heart, so you start talking about building a highway, and I'm going to yell bloody murder.
Kyle: Yeah, I'm an enlightenment hippie at heart, so you kind of aimed at data companies. So you asked me about Big Brother, I'll talk for like three hours on it because I forgot. In some way, I've rationalized my work.
Kevin: But let's go back, because before you talked about that freeway. I was super into it, this idea of the way that the data that we collect from all the vehicles that we could. I was thinking not only about carbon emissions, but also I was thinking about traffic patterns, and with self-driving cars, presumably you could avoid that the rup-let of people moving from point A to point B could presumably be astronomically better.
Kyle: Yeah, you can find some really interesting stuff. There's some really interesting applied machine-learning analysis that's helping people detect Parkinson's disease by the accelerometers in your phone. Because we got a large enough dataset that we could tag people who have Parkinson's, and we know what their phone accelerator behavior was inside of Angry Birds or whatever for long enough that we can detect Parkinson's earlier through these systems than we can through getting medical checkups. That's fucking awesome.
You can only do that if you have this kind of data in aggregate. This week I was in Denver talking to an entrepreneur who started a company that's using similar concepts inside of Fitbits and health trackers to early-detect diabetes based on their motion. That's the kind of stuff that I think is, to me,
that's the really inspiring stuff is what can we do with broadly aggregated data that you can't even do inside of a science lab.
I think that's where there's some really interesting stuff. Most of this technology has basically been used to get you to do one of three things: get you to spend more money in the casino, get you to click on ads, and to screen you on your insurance premiums. The most sophisticated data science has been used largely for that. I think that it will be used in a lot of different ways when the tools get out there.
Kevin: I'm all for it. You're such a good futurist, and I am such an old hippie. Do you have other visions that you've talked about, that you thought about?
Kyle: I think you're going to have to be more specific.
Kevin: Okay. We've talked about medicine. We've talked about transportation. I am interested in the autonomous cars. Because I'm confused, how do we get from where we are today with a few, we see them driving our office with little spinnies on top, how do we get from a few of those to a fleet of successfully moving-around cars? What's the role of data in that?
Kyle: How we get there, I don't know. What's the role of data in that? There is one really interesting piece, which did you see the fatal car crash that involved a Tesla a few months ago?
Kevin: Yes.
Kyle: Did you see the blog post response that Tesla issued, I think, later that day?
Kevin: No, but can you summarize it?
Kyle: I can't summarize it, but there's one really interesting point that they made, which was they reported on the number of miles that self-driving Teslas have driven to get to this first accident, and the number of miles that a normal driver drives to get to the average accident, and it was pretty stark.
I don't remember the numbers, but it was pretty obvious just from that one dataset, pretty obvious the self-driving cars are a lot safer. And this was the case for the self-driving car probably wasn't at fault.
I don't know if the courts have come down. That's what it sounded like, but of course, I was reading Tesla PR, so they would probably make it sound that way no matter what. But the data piece is pretty conclusive to me, at least as a citizen. I was like, "Oh, well..."
I don't remember exactly what it was like. We've driven like 400 million miles or something, some crazy number, I don't know. And normally drivers have accidents, there's an accident every I don't know, three million miles or something. I don't know the number. You'd have to look it up.
Kevin: I bet it's less than that.
Kyle: But how do we know that? Well, we know that because those cars, while they're driving around and making all these decisions, they're diligently recording the decisions they made and the miles that they've traveled and the routes that they've driven on. So that's a system that can actually get better as time goes by.
The more miles they have, the better that system gets. Whereas it doesn't seem to be that way with drivers. As an individual driver, the more miles you get, the better driver you are.
Kevin: Until eventually, maybe, diminishing returns.
Kyle: If all other things being equal, more miles, I think, makes a better driver. There's motor factors that might correlate with mileage that maybe hurt you as a driver, like your vision gets worse or your reaction time as you age or something. But the machines don't age, and the same algorithm is driving all those miles and learning from all of those encounters with pedestrians and with cyclists and other cars.
So, as that network expands, it actually becomes a safer and safer driver in a way that we don't. And especially with states, like, we're moving backwards on driver education. I grew up near Missouri. I know you didn't, at least in the '90s, have to take driver's ed to drive a fucking car. And guess what? They can take that Missouri license and drive all over the developed world.
I'm not certain that we're actually getting better, even just from a societal level, at driving. And the machines are getting better.
Every day they're out there in the road, and they're taking in new inputs, and they're learning from their mistakes. Lesson number one. Number two is what happens when more of the other cars on the road are self-driving? Now they can synchronize their behavior. I kind of have this vision of the world where two cars, like okay, there's two cars--
Kevin: Okay, you got your hands one in front of the other.
Kyle: Yeah, one is in front of the other, right? You're in the left lane. I'm in the right lane.
Kevin: Okay.
Kyle: You're a little bit ahead of me. So maybe I'm in your blind spot.
Kevin: Okay.
Kyle: But if we're self-driving cars, I'm not in your blind spot. There's no blind spot. You know exactly where I am, because you have eyes everywhere. And if we're synchronized, let's say you needed to come into my lane, I need to go into your lane. Do you ever have this encounter with a driver where it's like, "Oh good, they're trying to come in the same way I'm trying to go, so we can switch a little bit here."
But it takes some recognition and some turn signals, and the other driver has to get it, all that stuff but it's kind of beautiful when that happens, but I imagine a world where those are both controlled by the same algorithm. That just happens. That's just it.
You can imagine those kinds of negotiations happening far in advance. Like, "Hey, every other car in the road, at some point in the next three miles, I need to get over two lanes to the right. Can we do this in a way that's elegant instead of creating a fucking traffic jam?" Because I hit my blinker and nobody would let me in, and I'm stuck, and everything's backing up for miles because of this.
Kevin: And that person's texting.
Kyle: Right, and there's often a way to execute what you want to execute on the road and in a way that doesn't make anyone else reach their destination later than they would. So if you could communicate that, and kind of trade on that future, you could actually negotiate the stuff ahead of time.
Machines can do that. People can't do that. So the more these cars are on the road, the safer and more efficient it gets. The only way we can do that is by monitoring what's going on and learning from what's happened. And I think that's the role that data plays in it.
Kevin: Okay, but if you say you don't know exactly how we get from where we are to where we're going to get with self-driving cars, and you can't tell me exactly what the world is going to look like 10 years from now and how data is going to play a role in it, then how can you be sure that we can come along for the ride, if we're just living in today with our product and helping people today? And we don't know what the future brings? How does that work?
Kyle: Well, I don't know what this future brings, but I can speak convincingly about it. Sky covers something.
Kevin: I know, but how can we stay compatible with the future if we don't know what the future is?
Kyle: To some degree, I think that comes from a couple of things. One, this actually runs to like corporate strategy. How does a business day relevant generally? No business can break the future. There might be a hedge fund in Connecticut that can do that, but nobody else seems to be able to predict the future.
How do they stay relevant, given that they can't tell the future is coming? So this is like totally abstract strategy. There are a few options. One, guess on a certain future and put everything behind it. And then if that future develops, you look like a genius, and you win.
Kevin: Okay.
Kyle: Which isn't always a bad move, right? That can work. Number two is diversify. Let's say that there are three outcomes that are the most likely. Out of all the millions of outcomes, there are three that are most likely. So how do we set ourselves up in a position where any of those three would be acceptable to us, and we would survive?
Maybe we kind of thrive in case A. We kind of thrive in case B. In case C, it's difficult, but we don't die. We survive. If there's a sequence of tactics and a strategic set that allows you to win no matter what, and the situation is looking craps.
If you had the chance, if you didn't have to roll the dice to load up the craps table, you would just pick, "Oh, it's craps. I'm going to load up all the numbers. I'm going to make sure that before it gets rolled a five, gets rolled a six, or an eight, or nine, or 10, I'm going to make money." But you can actually do that in business, which is interesting. You can load up the six even without waiting for six to be rolled. You could also develop craps, but they're going to be worse odds, so.
Kevin: Right.
Kyle: I don't want to disappoint you. I know, as my craps teacher, you would be disappointed if I didn't remember that. So how does that translate to what we do, and how we look at the world? For five years now, people have been telling me to pick a vertical and sell analytics into that vertical. And I have said no.
We might do it occasionally, and we're going to start doing it a little bit more after five years. But if you focus too narrowly on it, what happens if you picked the wrong one, right? What would happen if you were selling analytics to the social games industry in 2010? Well, you'd make a shitload of money until 2011, right? What would happen if you were selling analytics into the daily deals industry in 2011? You'd make a shitload of money until 2013 rolled around.
Kevin: Got it.
Kyle: If you over-index on a given market, you're betting everything on that market. And we just don't do that. That's from a kind of go-to-market perspective. From a product perspective, what we've essentially said is we make a product for developers. Those developers will build us into the products they're making, so this is a very diversified strategy.
The reason it makes a lot of sense is, developers that are using us tend to be employed. And employment is the best leading indicator that a vertical might be working, right? There are 10 million, maybe 20 million developers in the world, depends how you slice them. Those developers, at one point, none of them worked at daily deals companies, and at some point, thousands of them worked at daily deals companies, and now we're at a point where maybe very few of them do. But they were developers the whole time, right? So all those developers who used to work at Groupon work somewhere now.
Kevin: Right.
Kyle: And whatever tools they adopted and enjoyed when they were in Groupon, they're probably using in wherever they are now. And it's probably in a different industry, given that there's no other jobs in daily deals.
By betting on developers, we're essentially saying, employment follows the dollars, developers follow the employment.
If we make something that's generally useful to them, we'll bring us with them wherever they go. So that's kind of the survival strategy. Survival strategy is, we're not smart enough to guess. We've assumed we're not smart enough to guess the market, so we're going to find a way to diversify on the market. And that's kind of how we do it.
Kevin: And from a technology standpoint, what steps can you take not to make your technology become obsolete? I had friend at Commodore 64. It was awesome, but no one would want to use it now.
Kyle: I think playing at the right abstraction level is one of the most important pieces here, right? So, Commodore is not a business I would be in, because if you are dominant, like 64 was pretty dominant, another two years roll around, and you have to come up with a new way to be dominant. So you basically have to win every hand or you die, right?
Right now we're watching Nintendo, which is, of all those OG companies, by far the most successful in the long term so far. But they just had a flop. It's first flop in a long time. They flopped in '90-something with the GameCube, and then they just flopped with the Wii.
Nintendo is struggling because of that, because they have to win every single hand. They can't fold a few hands and then survive.
What they could do is keep trying to build new consoles and hope that the next one hits. You really can't miss two in a row, then you're SEGA and you die. Or they can start diversifying. So what we saw with the Pokemon rollout, what we saw with them developing software for other people's hardware for the first time, putting IP onto the iOS app store and in the Android apps, Google Play Store, we saw them diversify.
We saw them disconnect their business future from "Are we making the top console?" So, how do you apply that to where we are? I would do it by making a highly configurable, API-based product.
Kevin: That's what we did.
Kyle: Yeah, imagine that. You can learn a whole lot about what strategies I would deploy just by looking at what we do.
Kevin: So then let me flip the coin. Let me say, for our customers, how will the best organizations be using data in the future? How will it be important and valuable?
Kyle: I think that
data, it becomes the most impactful when it's invisible or when you don't know it's analytics or data science you're dealing with.
So a lot of organizations, right now the state of the art seems to be make a centralized analytics reporting data science team. And they're the ones that are scientific thinkers, and then all the other departments in the organization try to work with that team.
I don't think that's how it's going to be in the future. I'm not making this up. I'm just kind of stealing ideas from Facebook, which is again, like I said, into the best of it right now. What Facebook has is, inside their product organization, they have analytics as one of the four major citizens.
There's project management, engineering, design, and analytics. Those four citizens work in harmony on everything they make, so it's not, "Go over there to the analysis team, file a request and hope they get us a report back." It's, "We have an analyst here."
And the tools are easy enough for all the teams to use, because their internal tools honestly don't look that different than ours, than what we sell. What they're basically able to do is embed the scientific thinking inside of everyone else's workflow, right?
You're a marketer by training, by professional background, and you have a math background, so you're capable, just as capable, as an engineer. You understand the same levels of math to look at how many page views came from this blog post versus that one, and actually interpret that.
The difficult thing is getting the tools in your hands. But if the tools you're already using, let's say that you're using a content management system as a journalist to write content and to publish pieces. If in that same content management system you've got analytical feedback and subjective feedback in the same stream, you can see the comments from users, you can see the tweet penetration, you can see the read completion rate, you can see the viral rate and the share rate.
You might not think, "Oh it's great that we've got analytics in our system now." You might just think, "I know what percentage people share my story." Everyone can understand what you mean when you say what percentage people share your story on Twitter.
Kevin: Right.
Kyle: What percentage people who start reading it finish it. What's the median amount of time people spend on the page? And you can ask me why median instead of average, we can go on. That's a long conversation, but that doesn't sound like data science and analytics. It just sounds like I'm getting a little bit more detail on what's going on with the stuff that I make.
The best companies are going to be the ones that do it that way, the ones that integrate pretty deeply in another workflow. The worst companies are the ones who are like, every quarter, the editor-in-chief asks the data team for roll-ups of how every article performed, and they try to make subject matter decisions based on that.
Those companies are going to be beat by the ones who move quickly and who, everyone in the organization, that can have quantitative information that can help them with their job is going to get it.
Kevin: The example you gave is blog post and sharing in a very sort of online, fast-moving company. What if I'm making products that aren't like that?
Kyle: Yeah, a good example could be like, let's say that you're in a warehouse, timeless industry. You're in supply chain, logistics, and you're running a warehouse. So there are a lot of things that Amazon did really well. Amazon does a lot of things, scientifically, really well.
One of the things that they've done really well is they know everything about their warehouse. They know the seconds that it takes for a forklift to go get a palette. They know the route distance to pick up items. They know the lead time on everything in their warehouses.
They have tons of options for how to ship anything to point B. They know how to vary, "Which point A should I send from to get you the thing that you want?" They're so far ahead on warehousing, even compared to some of the competitors from the last gen, like the Walmarts of the world.
Why are they so far ahead on warehousing? It's because they have data science up and down their company. They don't look at it like an add-on. It's how they think. They think scientifically, and they needed the answers to various questions. And they had the resources to build the tools to give them the answers.
So, to me, I think
every industry that isn't deeply scientific and getting us close to real-time as possible, is going to be disrupted by somebody like that who is.
Yes, they have the best storefront, but so did Buy.com and Half.com and a bunch of others. Frugal, which is a Google-product thing, they had the traffic, too, but they couldn't get it done.
I think they don't have the warehousing-data-science stuff figured out, and I don't actually know very much about that business, but the point is that that's a really old industry. You don't think about forklift drivers as data scientists. You don't think even the person riding the forklift team, the floor manager in a warehouse, as an analytics professional.
But the ones who win, as I dig into the companies who have won, there's a pretty consistent theme, and it seems to be that when they have scientific questions,
first of all, they're allowed to have scientific questions. And second of all, they have the tools to answer them.
Kevin: You talk about analytics being invisible, and it just being a part of the workflow, and we won't even think about it. But I can think of examples as a consumer, examples where it's non-invisible. It's something that I want to see. I feel like I am increasingly accustomed.
If there's any sort of web service I use or even a game I play, I have this sort of nerdy desire to know how I'm doing. And I, frankly, get frustrated when I can't find that information, because now that I work at Keen, I know it existed somewhere. Why can't I see it?
Kyle: Yeah.
Kevin: Is that a fad?
Kyle: No, I don't think that's a fad at all. We'll talk about the billionaires, or people in the world who are in the most developed economies and have most access to this kind of stuff. I'd say that you're probably in the 90th percentile, plus that you're in the minority in terms of really, really craving that stuff. But it's growing. I don't think that's a fad.
I think curiosity is a natural human trait that hopefully is never going to go away, and quantitative exposure is like all kinds of exposure, it's something that can only grow.
It's interesting that you mentioned games. Games are one of the ways that that stuff is growing, and one of the reasons that stuff is growing, right?
Why is it I have my entire track record from some throwaway iPhone game in my player profile, and with the SaaS product that I'm paying, or maybe the company's paying, $5,000, $6,000 a month for, I can't tell anything about what's going on.
Kevin: Right.
Kyle: The things we learn as consumers using software, they inform what we demand as business users. Because it turns out every business user is also a human. And they consume non-business things most of the time, a lot of the time. So I don't think it's a fad.
I don't think it's going away at all, but I do think a lot of people don't think of that as analytics. They don't think about a leaderboard in their game is an analytics view, right? They don't think Strava and Runkeeper, those are just nothing but analytics apps. Those are analytics apps. Fitbit is just an analytics device. That's all it is. It's a thing that records stuff, and shows you dashboards about it.
But most people don't bucket that as analytics. When you ask them about the impact of analytics, they don't think about the fact that that's what those products are, and that's what I meant when I said "invisible." I don't mean that it's not there. I just mean that people don't, maybe, compartmentalize it. They don't describe it that way.
Kevin: It sounds like you almost feel like people notice it when it's not there, and it's an irritant.
Kyle: Right, right. Yeah, people want all kinds of information, and quantitative information is great. I like to know how many mentions I get. I like to know how my tweets have been performing. Everybody likes to know how many notifications they get on Facebook. That weird and strange, when you open Facebook, and it has like a little "33" next to the thing, and you're like, "Whoa, 33 various things? That's amazing."
Kevin: All about me.
Kyle: Yeah, most of them are not even really anything, but they can't take that away. You can't take away that little number. That little red badge, you can't take that away. There is no way.
The quantitative information's addictive, like all information.
I don't think people even, like the notification center, or whatever that thing is in Facebook, that dropdown, I don't think people see that as an analytics future. I do. And we do, maybe, because it's what we do.
Kevin: Right. It's hard to unknow what you know.
Kyle: Yeah.
Kevin: In many cases, I don't think what I know is making me notice something I wouldn't. It's just making me aware of something that I would have noticed and wouldn't have understood the technology required to provide it.
What does it take to show the customer that history that we're talking about? The same thing that exists in the game, can that be exposed to me? Is somebody hiding it in a black box, or did it just never get captured?
Kyle: Yeah, I've had people ask me, "What's a well-known example of analytics being kind of natively put into a product?" And my favorite example is YouTube. You look at a YouTube video, and in the bottom right there is a little counter that tells you how many views that video has.
That is a super complicated and extremely difficult analytical system to build, where you imagine a video is going viral, and that counter's got to up into the billions range, and it doesn't seem to be that cached, because when you reload it, it goes up.
That's actually a fairly complicated systems-engineering feat to perform. But to the user, it's like, 'Well, of course it has a view count. That's not a big deal.'
Kevin: Yeah, I guess when you say, "Of course," I'm not an engineer, and I feel like, "Yeah, a counter." I picture the guy at the train station going "click, click, click." How hard is that? "Click, click, click, click," on the little clicker.
Kyle: Yeah, it's hard to picture a billion.
Kevin: Yeah.
Kyle: But that's the thing, when I say it's invisible, that's what I mean. It's not a dashboard. It's not a line graph or whatever people think when they think analytics. That's just a number on a page. But imagine YouTube without that number. I don't want to claim to know what it would be like, but it would be shit.
Kevin: Yeah, and if they said they'll remove that feature, there would be an uproar.
Kyle: There would be an uproar, and if they never had had that feature, maybe the product wouldn't have worked at all.
Kevin: Right. So, even though that's not what the core product is, the core product is hosting and displaying videos. That's the core product. But the validation that comes from that counter, or the community that comes from watching something you like, "Ah, I'm in the know, because, honey badger, it's me and 12 million people. We're all watching honey badger."
Kyle: Yeah, and then to some degree, that's the product.
Kevin: Right.
Kyle: To some degree, the product is the quantified popularity of whatever you're seeing. I saw "Gangnam Style" before it was a billion. I'm pretty awesome. Somebody saw Gangnam Style before it was 100 million.
Kevin: And they know it.
Kyle: Probably about 50 million people, because I'm sure everybody watches that at least twice. So, to some degree, that is the product. It's hard to know. This is when analytics becomes so core that you can't really distinguish between the real experience and the analytics, right?
Kevin: And yet if you thought about removing the analytics from it, it would fall apart. Pitchforks.
Kyle: Yeah, that you see in gaming. The product itself is supposed to be the most fun thing. It's a game. It's, in theory, oriented around the fun of actually playing it. And yet a lot of gamers you talk to about, "Oh yeah, what game did you play this weekend?" They'll be like, "I played this game." "And how was it?" "Well, I got to this level really quickly, and then tonight I'm going to get to this next level, and I'm collecting." It's like a whole bunch of counters going up.
Kevin: Right.
Kyle: As opposed to the actual experience that they're having. I think that there will be some backlash from that. There already was in the Facebook world, where some games that only had counters going up, and didn't have any fun components, started to fail. But the trend is clear. Some of these are even really great games, and yet the experience that sticks with people seems to be the analytical stuff.
Kevin: You speak very confidently and compellingly about the role of data today and tomorrow. I am wondering, what do you think some other organizations are getting wrong about data? Organizations or people, like I said, I raised the whole Big Brother thing.
You said, "Well, Big Brother is happening whether you like it or not, but what we can do is make a lot of little brothers to keep him in check." So what are some misconceptions or some things that people have wrong about data in your mind?
Kyle: I think there are a lot of things. I think the main thing right now that a lot of people have wrong, and it's just kind of awkward given how we started the conversation, but the main thing a lot of people have wrong is this idea that bots and AI and machines that learn on their own, they're here now.
They weren't here yesterday, but now they're here, and now everything has to run through them. Because Gartner said a couple things, because Mark Zuckerberg talked on the stage about different kind of bots that are completely unrelated to data. This buzz has begun to develop, and I think that's going to subside pretty quickly.
The technology hasn't made any significant leaps in the last three or four years, from my view, and yet the buzz has grown hundredfold. It's huge now, and this is a thing. The current shiny hammer right now is machine learning, and everyone's running around saying, "We've got this great shiny hammer. There must be a huge market of shiny nails that we're going to hit with this hammer." And they're thinking backwards.
You're supposed to start by looking at what kind of nails are out there, and then build a hammer for it.
So a lot of people have that wrong. There are a lot of new companies getting started in the space. The largest companies, I'd say outside of those four, five kind of internet native companies, the largest companies are becoming obsessed with machine learning and AI, and there's not a ton of meaning to it; there's a lot of PR.
This has happened before. When DeepBlue was a huge PR exercise, when it beat Kasparov and as a result, everyone went apeshit. Now they're saying, "If it can win at chess, it can win at everything." I was like, "No, it's a chess bot. Yeah, it can win at chess. It's a chess bot."
Kevin: Right.
Kyle: Google did a similar PR exercise over the last couple years with AlphaGo, which is a deep-learning bot. See, deep learning, that's not the same as machine learning, which wasn't the same as AI. Now we figured out how to make the thing sentient by rebranding it and making it good at a different game. Now it's good at go.
It is a nice incremental step. It's way harder to make a machine play go than chess. You can't brute force it, and all this other stuff, but it's still a go bot. It plays go really, really well. And Watson was a similar exercise, when Watson beat everybody at Jeopardy.
Kevin: But it doesn't play Wheel of Fortune.
Kyle: Well, you can flip a coin to play Wheel of Fortune. Actually, the word-guessing part is probably, I enjoy that part. This was skill to it. But just because a major company has put a lot of money into research project to do something that gets great PR for a certain tech set, doesn't make that tech set suddenly more relevant to the marketplace than it was before.
The marketplace is the same regardless of whether or not the top go player just lost to a robot.
The marketplace is where the actual signal is, where the actual truth is, to me, not what vendors are saying. Vendors say all kinds of shit to try to grow business. So I think a lot of people have that wrong right now.
You put me across the table with the CMO, the Head of Marketing at any Fortune 500 company, even the ones that are really good at this stuff, and I will probably be able to ask them 10 questions to find something they don't understand and they don't have an answer to.
I'm not a machine-learning super bot. If you just ask people, like you ask a head of marketing at a major brand, "How much money do you spend on advertisements?" And they'll be like, "Oh, $33 million a year. It's what our media buyers did last year," or whatever. Okay, how much of that was spent on online ads? They'll be like, "$10 million." "How did you split that between display ads on random websites, and Google, and paid content, and Facebook, and Twitter, and LinkedIn, or whatever?" They'll know those numbers.
If you dig in one step deeper, nobody knows the freaking answers. Very rarely do they know answers to questions like, "What job title correlates most directly with people converting through your marketing funnel into paid customers?" They'll be like, "Well, we don't really do our campaigns by job title." But the data is in there.
You know the job title of the target of each one person that clicked. They just don't know the answers to these questions, because they don't have, I won't explain why, they don't know the answers to these questions. And yet they're trying to figure out how to deploy millions of dollars on deep learning.
If you can't tell me basic numbers of things that have happened in the past, reasonably up-to-date data where I can segment a little bit, and drill in a little bit on the sample set, basic, simple analytics, I think we're getting ahead of ourselves if we're like, "How do we make an automated decision bot that tells my data team what questions to ask?" And all this other stuff.
It sounds like a lot of fluff. A lot of people are talking about that right now. That's the thing that I think is ridiculous. We're not even counting things very well right now.
Kevin: And where you started out a while back, was saying you don't think the data is going to become sentient. You just think the data is going to become more accessible and allow people, human people, to make smarter decisions.
Kyle: Yeah, but the reality is, the downside of that is, it also allows human people make stupider decisions if they don't interpret it well. And human people will make buying decisions based on all kinds of factors that aren't necessarily rational, right?
They might just think, "Hey, my CIO has an urgent mission for us to make sure we're spending 20% of our money on deep learning this year." You'd think that's an absurd way to make decisions, but that's pretty common, actually. It doesn't matter how many times Gartner rose up their Hype Cycle, where something goes way, way up and then it goes to, like, the trough of, "This is bullshit, and we just realized it."
They'll still spend a bunch of money on the uptick, and because of that, during the uptick, new companies will be formed. They'll get certain amount of sales traction. They'll raise a bunch of money. They'll sell a bunch of bullshit to the bullshit buyer. And then it'll come back down.
Right now I'm seeing that a lot with machine learning, AI stuff. Machine learning and AI are having a huge impact on the industry generally, where there's an actual problem to be solved. And people say, "Oh, here is a potential solution to that problem. Maybe there are some things we can use here." But it's happening the other way around right now.
I've had frequent conversations in the last couple months about this, where they're like, "Oh you're a really cutting-edge analytics and data company. How can we use deep learning in our business?" And like, "Can I tell you about my business, and you tell me how we can use deep learning in our business?" And I'm like, "That's crazy."
I'm like, "Why don't you tell me about your business, and I'll just provide general suggestions?" Don't bias me toward a certain answer before you ask me any questions. That's not a good way to learn. So I'm seeing a lot of that.
Just count stuff right now. That's a good start.
If you're not counting good stuff, you know the answers to basic factual questions right now. Get those first. Even if we do get machine overlords, we're going to have to feed them the right facts. Bots eat facts. They need facts. So a lot of companies are trying to get to the bots before they actually know things. It's totally broken, Kevin.
Kevin: Let's fix it.
Kyle: It's hard to. You can't fight the Hype Cycle. You just have to make sure you have a timestamped tweet pointed out so you can look back later and be like, "See this tweet I wrote, that said that was bullshit?"
Kevin: It's almost as if that timestamp is a form of analytics. We've covered a lot of ground today, but let me just throw a few sort of lightning-round questions at you, and we'll see if anything comes to your mind.
Organization you would most love to work with. We've already worked with NASA, so that one's off the table. An organization you would love to have the chance to work with over the course of the next 50 years.
Kyle: I would love to work with Nintendo. I think Nintendo is awesome, and I know that they have a cultural bias against analytical stuff, like a lot of creatives do. I even have a gut bias against analytical thinking, because sometimes human expertise is better, and you can't measure and optimize your way to art or to culture, and that's what they do.
But I think they would be stronger if they also optimize the things that can be optimized. So, when you asked me that question,
I think a lot about companies who, because they don't want to become robots, they refuse all enhancement. I'm much more like, 'Let's be cyborgs.'
Let's keep the human parts and add the robot parts that make sense, right? Like, I'd love to add some robot knees. Knees are stupid. Nobody's knees last long enough. I want to fix that part.
Anyway, Nintendo would be really cool. I've just been a huge Nintendo geek forever, and as I watch them start to struggle a little bit, it scares me. I'm a dad now. It's important to me that Nintendo be around for 50 years so that my son can spend a lot of money on Nintendo like I did.
I think it'd be really interesting. Some organizations I'm really interested in. You're talking about dream organizations, ignoring all the kind of commercial factors?
Kevin: Yeah, of course.
Kyle: I think a lot of my favorite potential use cases are in the nonprofit and NGO space. For instance, the Bill and Melinda Gates Foundation, the malaria initiative. I think we may be able to help with that.
I don't know if that'll happen or how to do that, but I think there is some really interesting stuff you could do with connected sensors, if you had the perspective on data being easy that we have.
Like a lot of people, and that was started by Bill Gates, he's like one of the most brilliant technical innovators, right? But that group's exposure to data analytics and data science is dated. So what that does is it gives the culture a sort of learned helplessness around data. Like, other top execs say, "That data project would be too big," or, "It would cost too much," or, "It'd take too long." So we can't do that kind of thing.
That's something that you hear frequently from people whose first exposure to systems and computer science was '80s and '90s, is the data stuff is not going to work. And we could help dramatically with that. Most of what that foundation does seems to be right on what humans ought to be working on when they get rich, which I think is a really great foundation.
I think there's a lot of stuff that can be done around voter turnout, and there's also a lot of stuff that can be done around voting-system transparency. One of the most interesting and confusing parts about our system is that there are all these levels of voter transparency that were instilled into American democracy.
I don't know about the rest of the republics in the world very well or much, but at least in America, individual citizens can sign up to watch the ballots, watch the paperwork, watch the counting. We can get citizen oversight. Average citizens can show up, and it's way harder to corrupt average citizens especially if you don't know who they're going to be.
The idea is that we can make sure things are counted well and all that, but now that voting has gone electronic, we don't have a good analog to that. So I think that's an area where a third-party system, that's just collecting stuff to corroborate, could be really useful. By "third party," I don't mean third political party.
Kevin: You mean you.
Kyle: Just some enterprise, like something nonprofit, something that's unrelated. In general, I think the citizenry has a bias towards just making sure that the votes work and the system works, and I think it's one of these things you can't really talk about.
People from major political parties, they will never talk about it. Everyone's like, "This is fine." Well, I guess somebody right now is talking about it from a major political party. He doesn't really count. It comes from a maniac.
But I think it's an important thing to do, to offset when these people show up and start claiming voter fraud is a thing and ignoring the limited data we have around it and just saying, "Voter fraud is such a thing that we need to do these laws, and we need to institute these policies." They're able to say that because we don't have data on how the stuff actually happens.
Why don't we go into the industries where there's huge capital incentives to avoid fraud?
The iPhone app for Illinois Lotto probably has a better system for making sure people don't get extra, right? Because they have to. They have a huge incentive to do that. We could get that data, and we can prove the system works, if it does and if it doesn't work, we can prove that it doesn't.
I think that's really interesting. In general, getting stuff to the citizens is really interesting to me. You ever use that Facebook graph search?
Kevin: No.
Kyle: It's this thing that wants to be like, "Oh, I want to find people who have lived in San Francisco for this many years and that will have these likes," or whatever. It's like a simple search to dig through the structured Facebook graph.
Kevin: Oh.
Kyle: I don't know if it's still a thing, but they launched it a few years ago. I haven't heard about it in a long time, and you haven't even heard of it.
Kevin: I'm a very light user.
Kyle: This was like that year when Facebook was trying to build a search engine and Google was trying to build a social network.
Kevin: Oh, right.
Kyle: They're just chasing each other's tails. They weren't good at the other one's job at all, but it's a pretty cool tool. When I saw it, I was like, one thing that would be really awesome is to be able to do that with the political vote records stuff. So, "Four senators who voted yes on this bill, how many of them received campaign funding from a group that's funded by these billionaires?"
Kevin: Right.
Kyle: Like that sort of thing, to be able to do that quickly. We can do that, but it's like a ton of work and a lot of digging through stuff. To be able to do that quickly, and moreover to be able to do that in a way that's structured so that the analysis could become automated, would be really interesting.
We'd find ourselves in a situation where, as soon as the bill clears the floor or doesn't, we would be able to find the correlations to funding automatically. So instead of, right now, a journalist has to think, "Huh, I wonder what..."
They've got a narrative already, so I'm going to go dig in the data to see if it's true that NRA-backed senators did this or that, or whatever it is. They have to have a hypothesis and then guess. But that data is a matter of public record. If it were in the right system, and this is actually something that Dan and I did at Keen in 2012, imported some of these data just as a test to play around with it. If we're in the right system, we would be able to find that stuff automatically.
I think there's some really interesting stuff that could come from that. I don't even know who the customer is. That's just a project I think is really interesting. And then my dream customer right now, that's an actual private enterprise that kind of makes sense, is Tesla.
I think Tesla would be really cool to work with. If only because if I ever get a car, I want to get a Tesla. I hope I never get a car, but if I do, I want to get a Tesla. And they have some pretty cool dashboards in the car, but there's so much more stuff I would want to see. I think it'd be really interesting.
Kevin: You're saying there's things you actually want to put inside the car.
Kyle: Yeah, inside the car. So much stuff. You ever have this experience where, let's say you have a friend, like recently our mutual friend, Mike, had gotten a Volkswagen Golf GTI?
Kevin: Yes.
Kyle: As soon as he got that, I started seeing them everywhere.
Kevin: Yeah.
Kyle: You had that experience where you--
Kevin: Yes.
Kyle: And I don't know if it's because they are more common and he's on trend, or because now that I've seen it so much up close so many times, I'm starting to look for them?
Kevin: I think Volkswagen may be a little behind trend right now.
Kyle: Well, now, yeah. But fair enough. But it'd be really cool if I had a car that is with me every time I'm out seeing cars, and I could ask it questions like, "How many Volkswagens have we seen on the road?" To me, I don't know, I really like Knight Rider. Did you watch Knight Rider?
Kevin: I'm the right age to have watched Knight Rider.
Kyle: I'm a younger sibling, so I grew up with Knight Rider, too.
Kevin: All right.
Kyle: To me, the really interesting stuff, when he talked to KITT on his watch or whatever,
the interesting stuff was when KITT had a little bit of historical context on stuff.
Kevin: Oh, yeah.
Kyle: Well, obviously I think historical context and "softwares" are interesting. That's my whole career. That stuff's really cool to me, and I think there's a lot of opportunity for that.
Like, man, like I fell off my diet and I ate at Burger King. Have we been passing Burger King billboards lately? If your car is just like, "Actually, we hadn't seen them in years, and now there's this one billboard that we have been driving past."
You could notice that stuff automatically, because I drive it to a fucking Burger King. This is the kind of stuff that we, by having cameras and location data and internet connectivity on a decently strong computer in your car, which Tesla has, we could do all kinds of interesting stuff.
Kevin: I know. The way I think about it is, can we find the same voice actor who did the voice of KITT?
Kyle: Mr. Feeny.
Kevin: Mr. Feeny.
Kyle: Yeah.
Kevin: Yeah.
Kyle: I learned that recently, that was Mr. Feeny from Boy Meets World. Obviously I would want that.
Kevin: Right. That goes without saying.
Kyle: I think there's just a lot of interesting stuff we could do by putting some long-term memory into your car. I think that'd be a really interesting thing.
Kevin: It's sort of like the old phrase, "If these walls could talk."
Kyle: Yeah, and then some trend stuff too. If these walls could talk, but this is like, we don't have the smart home yet.
Kevin: Right.
Kyle: But we do have the smart car. That's pretty cool.
To me, Tesla could be like the iPhone by opening up, so people like us can throw our ideas in it.
Or it could be like the Palm, which is a company that doesn't really exist anymore, that didn't open up its doors and let people write software into it.
I think it would be fun to help them with that kind of stuff. And just as a driver, it would be a lot of fun for me to be able to geek out on this kind of stuff and have it in my car. That'd be the best.
Kevin: Oh God, and you know what I want right now, is to have the Knight Rider music come on to play us out.
Subscribe to Heavybit Updates
You don’t have to build on your own. We help you stay ahead with the hottest resources, latest product updates, and top job opportunities from the community. Don’t miss out—subscribe now.
Content from the Library
O11ycast Ep. #74, The Universal Language of Telemetry with Liudmila Molkova
In episode 74 of o11ycast, Liudmila Molkova unpacks the importance of semantic conventions in telemetry. The discussion...
Open Source Ready Ep. #1, Introducing Open Source Ready
In this inaugural episode of Open Source Ready, Brian Douglas and John McBride embark on a technical and philosophical...
Jamstack Radio Ep. #98, Open Source Data Integration with Michel Tricot of Airbyte
In episode 98 of JAMstack Radio, Brian Douglas speaks with Michel Tricot of Airbyte. This conversation examines data distribution...