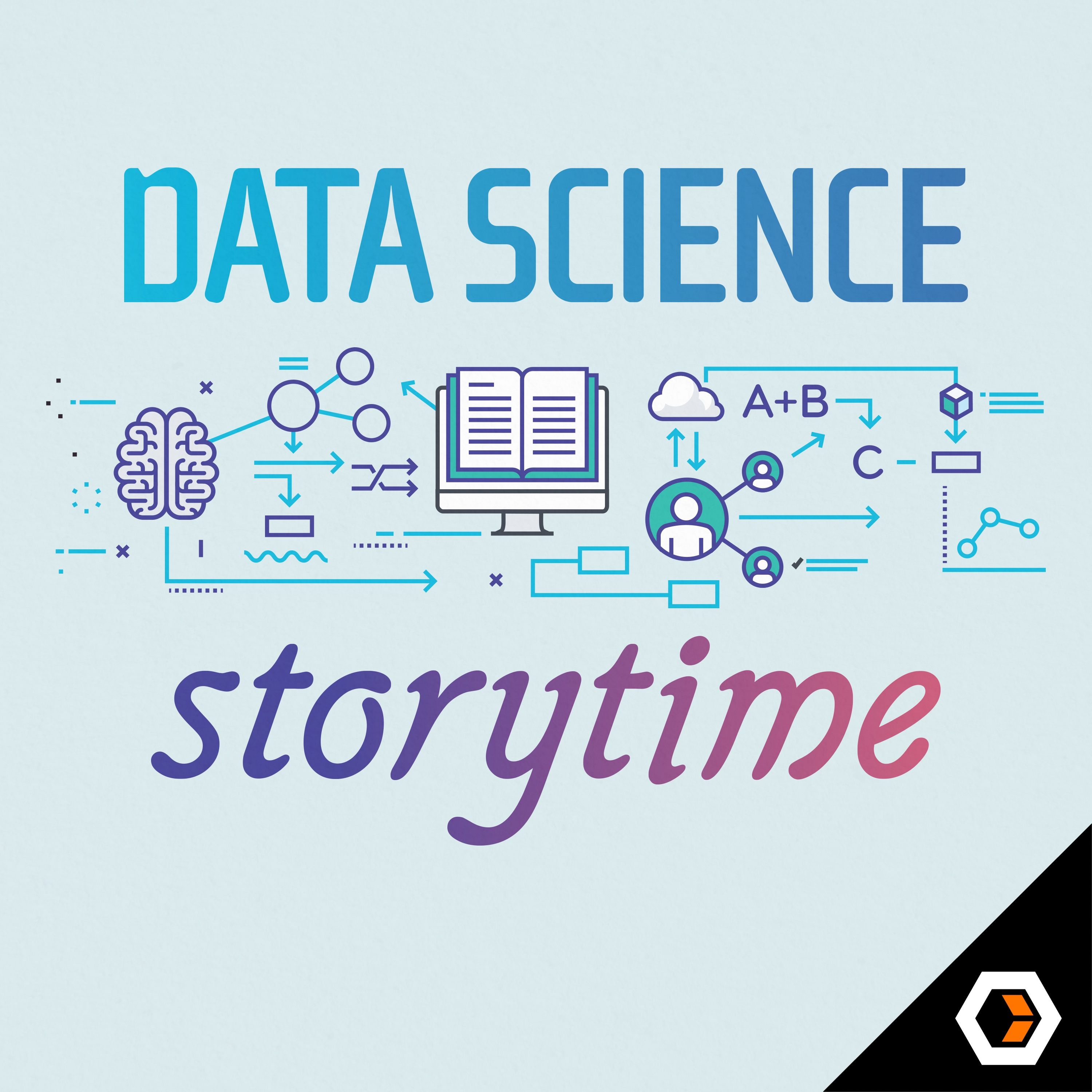
Ep. #4, Building A Cyborg Company
Kyle and Kevin are back, this time discussing the concept of a ‘cyborg company’. What exactly is a cyborg company and how is it relevant? Kyle explains that like the part-man part-machine cyborg, the modern company has machine parts and human parts, each with its own distinct strengths and weaknesses.
Can you identify your company’s machine parts? Why should you even bother trying? Human judgement oriented companies are doomed to fail. Purely machine driven companies also have major shortcomings. They aren’t creative and won’t value life. Listen in on Kyle and Kevin’s take on how to go about building a ‘cyborg company’ that combines the best of both worlds.
Kyle and Kevin are back, this time discussing the concept of a ‘cyborg company’. What exactly is a cyborg company and how is it relevant? Kyle explains that like the part-man part-machine cyborg, the modern company has machine parts and human parts, each with its own distinct strengths and weaknesses.
Can you identify your company’s machine parts? Why should you even bother trying? Human judgement oriented companies are doomed to fail. Purely machine driven companies also have major shortcomings. They aren’t creative and won’t value life. Listen in on Kyle and Kevin’s take on how to go about building a ‘cyborg company’ that combines the best of both worlds.
transcript
Kevin Wofsy: Recently we were having a conversation where you mentioned that when you first had the idea to start a company, you wanted to build a cyborg company, and the truth is I don't even know what you mean by that, and I wanted to dig in. What on Earth is a cyborg company, and why should we build one?
Kyle Wild: Okay, so a cyborg company. So what's a cyborg first of all?
Kevin: A cyborg is half man, half machine?
Kyle: Yeah, I mean I think they're more than 0% machine but not 100%.
Kevin: Okay, it didn't have to be an exact 50/50.
Kyle: Not exactly 50/50, right. So in a way like a person with a smartphone is kind of a cyborg. They connect to this digital hive mind, and pull stuff down, and bring in their organic brain.
Kevin: And then you have a selfie stick.
Kyle: Yeah, if you have like a robotic eye, that's almost a caricature of a cyborg, right? A human walking around with a robotic eye or something. So to say that we want to make a cyborg company I guess is to say a company that has human parts and machine parts.
Kevin: Which one am I?
Kyle:You're definitely one of the human parts.
Kevin: Okay.
Kyle:I believe that there are companies that are pretty much machines, and there are companies that are pretty much humans, and that they each have advantages in how they operate.
Kevin: Elaborate on that.
Kyle: So what's a good example? A good example, so let's talk about deforestation. Deforestation sucks.
Kevin: Agreed.
Kyle: I think it sucks. A lot of people think it sucks. I would say that the vast majority of people probably think it sucks and yet we do it. Why do we do it? Well, it turns out there's a big incentive structure for companies to do that, right? When they got started, they're like, "Oh, there's an infinite forest, so let's build a company that's designed to cut down the forest, and sell the wood or whatever".
How do we do that? "Well, let's build an incentive structure where maybe a given person gets compensated based on how much forest can they cut down, how quickly".
Kevin: Okay. I mean bad.
Kyle: Well, from where we sit today, that might look bad but remember, I said, starting at the beginning, we looked at the world and said, wow, there's like an infinite forest. There are only a million people, and there's so much forest, so it looks bad to us now because we know the outcomes but at the time, they're thinking "let's cut down this forest.
It's all there, and we're going to be able to cut it down forever". It feels like, right? Same with fossil fuels, right? Like fossil fuels felt infinite until maybe 50 years ago, and then they don't feel infinite right now.
Kevin: Right.
Kyle: Now we know there's some externalities, and bad side effects of burning so many fossil fuels but again companies looked and they're like, "well, let's just build a business that finds this crude oil, maybe refines it and sells it, or a series of companies that act in that supply chain". And again we're compensated based on how much of it can we extract.
So this is part of a classic phenomena called strip mining. Where you basically, whatever the limited, finite resource is, you just drill down to zero.
Kevin: Right.
Kyle: And a company structured to make quarterly returns, and do earnings analysis for their public shareholders is going to think in those three-month chunks, and if you had a company, this is very simplified, over-simplification, but if you have a company that's like, how many holes did you drill for oil? And how much oil did you pull out of the core of the earth in the last three months? And that's how you'll be compensated.
Kevin: Okay.
Kyle: Well, then what are you going to do every quarter? You're going to drill as much as you can every quarter. Especially if, let's say that you did that for 80 years and there's no visible impact.
Kevin: Right.
Kyle: Well, people only live 80 years so, even an individual that's been there the whole time doesn't see any impact. Obviously where we sit today in 2016, we know that there's an impact to that stuff. But they didn't know that at the time. So what they did was they built these machines, and I use machines very loosely. I mean a system that acts with or without human input.
Or a system that continues to act without any intervening human judgement, right? You might have a lot of human judgement at the start, like any machine, so far, machines tend to be built by people.
Kevin: Right.
Kyle: We had a lot of human judgement and the humans said, "hey lumberjack company, I'm going to give you this many dollars per tree that you cut down and send to me. And I'll give you this volume bonus if you send more during the quarter". Maybe somebody designed this comp model, this compensation model for lumberjacks in like 1830 and then that was the end of their judgement.
Now, the compensation model is actually part of a robot, in my view. It's part of a machine that's just kind of automatic.
Yeah, the lumberjacks are human, but they come in and they see this compensation model and they begin acting the way this compensation model would predict and would incentivize.
Kevin: Okay.
Kyle: So in that view, they're not human. They're human, but they're acting according to the machine's design.
Kevin: Because it's a repetitive program, we're going to cut down as many trees as we can, as quickly as we can, to make as much money as we can.
Kevin: Right. And repeat.
Kyle: And let's say that after 20 years of running this logging company, the CEO of the logging company has a revelation like, "oh my gosh, we're going to run out of trees if we keep doing this, we better change this model". It turns out that in many cases, even like the CEO of that company can't change that model very easily.
Kevin: Why not?
Kyle: Because well, what's a CEO's job? Recruit, retain, align, and motivate the best people and keep them funded to do their jobs. Well if the funding's all coming from the logs you sell, maybe that logging company has become a paper supplier, how do you change this compensation model, when it's the only way you know you're going to get enough logs to make your paper buyers happy?
Kevin: Okay.
Kyle: So, when I say the CEO has a revelation, what I mean is:
The CEO wants to inject human judgement and reasoning and values back into the system, but the robot, in some cases, has gotten out of control.
So a good example of this would be like, you know, at Google, they have a really amazing machine for search engine.
You go into a search engine, you type in a query, you get a result page and on that results page there's an advertisement. And that advertisement is bought in an auction where advertisers pay, the highest bidder gets that ad placement on the search results page. That's basically their whole business.
Kevin: Right.
Kyle: And it's all very well automated. What happens when they try to make a new product? Like a Google plus, is the people in that organization who have the compensation model to drive ad sales, they have political power internally to push the decisions to be for ad sales. This is actually one of the reasons that new companies usually make better products than old companies.
Because old companies have all this cruft, right? Xerox invented the mouse, the graphical user interface, but because it couldn't help the sales people sell more toner and the toner sales compensation model for printers and copy machines is how they monetize the company and how the company lives.
Kevin: Right.
Kyle: You can't actually introduce new product, especially if it's competitive with the toner sales. Even if your human judgement tells you, and even if the CEO's human judgement tells them, I want to go into this new area. The robot system is so powerful that you can't overcome it. So when we said we wanted to make a cyborg company, the point was, well, to me it's kind of self evident that human judgement could be useful.
The way I'm telling these stories, like in each of these cases, the human maybe is right, but they can't get their way. Robot systems are also very useful, you know? They're very efficient. If you do have a repetitive task, that's what a robot does is they do repetitive tasks well.
Kevin: Right.
Kyle: Just that when I say robot, I just mean that the encoded rules system and the rules engine. The automated stuff, the system design. Doesn't mean like, you know, a little humanoid with C-clamp hands swinging its arms around. What I mean is a thing that happens on it's own automatically, without intervening human judgement.
Kevin: Is it okay if I picture little robot with the hands?
Kyle: You can picture that if you want. I kind of think of it like a factory.
Kevin: Okay.
Kyle: Do you know what I mean? Like a factory, you've already built the machine that's going to put out this widget, three widgets per second on this one conveyor belt. If your business changes you don't need that widget anymore, well you already bought this machine.
Kevin: So tell me now, before you get to the cyborg, what's the other extreme? You've said there are companies that are like robots, there are companies like that are humans, what's the other extreme? What's one that's all human?
Kyle: Yeah, I it's hard to think of things of ones that you've heard of, and the reason is because...
Kevin: Because I'm ignorant?
Kyle: No, the reason is because
Purely human based judgement oriented companies don't usually do very well.
Kevin: Give me an example then, you gave me the example of the logging company, what's another example.
Kyle:So let's say that the logging company I talked about earlier is Paper Corp. And then there's another company called Lumberjacks Incorporated.
Kevin: Okay.
Kyle: Paper Corp, you know, they came in, they evaluated all the stuff, they set up a compensation model to incentivize certain amounts of trees to be chopped down and all this kind of, you know, very advanced system design. Whereas Lumberjacks Incorporated, maybe they were their competitor, Lumberjacks Incorporated has a way simpler model.
We hire lumberjacks, they cut down as many trees as they can, we pay them a fixed rate. We trust them to do the best work that they can do, and we hope that it's enough for our business to succeed. So everyday the lumberjack comes in and makes decisions. Every day, each lumberjack comes in and uses their human judgement. So this is not an automatic system.
Kevin: Okay.
Kyle: This is a system that relies on human judgement throughout. Usually those kinds of companies get destroyed when they go head to head with a company that's got more models, more system design, compensation systems, automation systems and so on. you know, a good example is, so in this world, Logger Corp shows up and they say, I'm going to give you $8 per tree.
And you think to yourself, well, that means if I can get people to chop down trees for cheaper, I can pay them less. If I can find a machine, if I can design a literal robot to cut down trees for me, I can still sell them for $8 a tree.
Kevin: That's my little guy with the hands.
Kyle: Yeah, little guy with the C-clamp hands. You know, scissors or something versus the Lumberjack Corporation, Lumberjacks Incorporated they look at it a little bit differently. They look at it like, we hire lumberjacks, lumberjacks come in and they do what lumberjacks do which is cut down trees and we trust them to cut down as many as they can.
Kevin: And maybe the lumberjacks know more about trees and they know about sustainability and they say, I can't cut down that one, it's too young. or I can't cut down that one because,
Kyle: Right. Whereas the robot says, They didn't say what kind of tree, They said $8 a tree. Or maybe they said $8 a tree that's more than 20 feet. So every tree that's 21 feet gets cut down. And maybe the lumberjack knows it's actually more efficient to wait till that tree's 40 feet, you get way more wood, way more volume, all these things that humans understand that weren't in the compensation model of the other company.
They would make this company, this human company stronger, because human expertise is valuable. But they get beat, typically, by the purely robotic companies.
Kevin: In the short term.
Kyle: In the short term, and if you get beat in the short term in business, that's called being put out of business.
Kevin: Yes it is.
Kyle: Yeah, so there's a long term impact of being put out of business in the short term. Which is, you dont exist.
Kyle: Forever. So when we looked at the business world, we kind of recognized that there's some areas where the sort of robotic, like lack of human judgement, the system that's all automatic and encoded has advantages. How fast can this search result page load. I want it to load as fast as possible. No matter how big the internet gets, I still want this to load fast.
Kevin: Right.
Kyle: Well, that's a very robotic like system. You can design a system where somebody comes in, they're the head of search results speed optimization and you give them a bonus based on how fast the pages load or whatever. And it's all very, like how they do it might be human judgement, but what they do is already designed in the comp model and in the corporate structure.
They're going to come in and they're going to make the search results look faster, period. So that's an optimization problem.
Robots are really good at optimization problems. And these kinds of systems are really good for optimization problems. Whereas a creative problem, like we want to design a new social network, robotics aren't very good at that.
It takes a lot of intuition, a lot of design, a lot of empathy, a lot of human judgement every step of the way. For instance, a human might say the world doesn't need a social network. Thank you for giving me this assignment sir, but the world doesn't need another social network, they're pretty happy with the ones they have.
Kevin: Right.
Kyle: A human would have never made Google plus. If human judgement had enough empowerment. And I'm picking on Google, 'cause I know them a little bit from the inside and because they're just a good modern example.
Kevin: I think they can take it.
Kyle: They can take it. They're a good modern example of a very optimization oriented company that uses systems, comp models and metrics for everything they do. Makes them really good at some things and really bad at some things. On the fully pure sort of human side, I think there have been some recognizable examples.
I think a good example would be Steve Jobs with the iPad. There are many reasons that people didn't build the iPad before. The iPad came about not just because we finally had the technology to make the iPad, but because we finally had a company where human expertise of one person was valued enough to take that kind of a risk.
So they made the iPad. Even during the iPad rollout and afterwards, everybody was criticizing them. The iPad sold a billion dollars per month for the first nine months. That's pretty good.
Kevin: Right, so, I mean, the same company made the Newton.
Kyle: They did, although Jobs wasn't there when they made the Newton.
Kevin: All right.
Kyle: So that's a case of a very human judgement oriented kind of company operating at the large scale. There are some down sides. They're not very good at optimizing things, for instance, they are under Tim Cook and they were in the manufacturing side, but my iCloud still doesn't work. This is an almost trillion dollar company, they can't figure out how to get my files to sync.
That's an optimization problem, very simple. How many, what percentage of files synced? I'm going to fire you if it's not 100%. You know, you can design this kind of comp model or incentive structure to optimize the approach. And I think had they done that well, maybe it would work. This is one of those things that comes very naturally to Google. Comes very naturally to Amazon. It doesn't come naturally at all to Apple.
So, the idea, when we said we wanted to make a cyborg company was to identify the areas in which machine-like thinking and systems design are most appropriate and identify the areas where human expertise which a lot of people call intuition, but that apparently has a negative connotation with some people, so I like to call it human expertise,
Kevin: Okay.
Kyle: Human expertise is very valuable. So, obviously your design functions should be pretty human expertise oriented. There's that classic story of Google trying to decide what color blue to use on a page. So they tested 20 different colors of blue and ran all the traffic through it to see which color of blue lead to the highest conversion, like that's crazy.
Come on. You have designers, they have very well trained, elite designers. Just trust your designers on color choice. I read a blog post about that. That's kind of an overblown example, and maybe it wasn't entirely true, but it does illustrate how a lot of decisions get made there. Like when I worked there, often times somebody would have a hypothesis, rather they would have an idea, somebody would say, How do we test that idea?
And the unspoken constraint was how do we test that idea so that anyone in the company would agree that the test was appropriate. We got unassailable evidence that this was a good idea before we executed on it. Which you can't do if you want to do anything ground breaking. You can't actually test your way doing ground breaking things, you have to be able to take risk.
Machines don't take risks. This is something they haven't figured out very well, is how to get machines to take risks and use their judgement to go for the unknowable things and go after them.
And this is kinda like the balance between art and science in business. We just wanted to make a business that has both.
Kevin: I mean, it's sorta interesting to me, because our business, we're in the business of data. We're in the business of analytics. We're in the business of letting people test hypotheses, and it sounds like you're saying, Yeah, but sometimes, just go with your gut. Is that what you're saying?
Kyle: Yeah, well, to some degree, yes. It's not just go with your gut. You know, all guts are not equal. It's just that expertise can be valuable, but the interesting thing about the scientific method is robots don't do a great job with the scientific method either. There's no robot that you can just ask a high level human language question to and then they figure out which experiment design will test that.
There's a step in the scientific method where you form hypotheses. How are those formed? You know, are they formed from data or are they formed from what you call the gut? And in my understanding of the scientific method, that first step of hypothesis selection is completely human. Like it doesn't say, here's how you pick the hypothesis that you want to test. So even the framing of the problem requires human insight. And then there's designing the experiment.
Like, I can't count how many times I've come across experiments, I don't read a lot of academic papers, but I do read a lot of abstracts. I just really enjoy them. And when I was in college, those were free, but you had to pay for the papers. And very frequently I see experiments that don't seem to be designed very well to test their claims. How do I know that?
They have a ton of funding and big lab and they collected all this data to try to prove this point, but the experiment was actually designed poorly and it doesn't fit to the question that they're trying to answer. And that's actually a big problem in the sciences. And we try to solve this by saying, Well, we're going to have it peer reviewed by a lot of different people, a lot of different universities.
And that tends to work. The reason it works is a bunch of different experts' gut opinions are less likely to be wrong than, you know, one expert's gut opinion. So even the scientific method, I think, requires a lot of human expertise.
Kevin: So, when I think about what you've told me. It makes sense to me that there are these machine companies, human companies. You know, in the real world, we've got robots. I've seen them. They make cars, they do things. You know, some of them are like the little dogs that bark, but they're robots. We have lots of humans, billions of humans running around.
But this cyborg, which is the human with the robotic eye, we haven't figured out how to make that yet. So it sounds like making a cyborg company would be maybe easier said than done.
Kyle: Yeah, I think so, I mean, I don't really know. I bet there are a lot of companies that have had this realization that some parts of your business should be more mechanistic and some of them should be more artful. That's unlikely an original insight. Maybe calling it cyborg company is original.
Kevin: Well, branding is everything.
Kyle: Yeah, like we haven't certainly studied everything out there on the market place. But it is after almost five years of it, it is easier said than done. I think, you know, you design a system and a model and a reporting structure and a compensation or measurement or success model that you can test against. And you hire people into that model and they're performing inside of it.
And then all of the sudden you realize that it's not the right model. It's actually pretty difficult to go to those people and say, "hey, the true north that we set for you, totally wrong". And when you go to people and ask them that or tell them that, they ask, How do you know it's totally wrong? And I'm just like I just know it's wrong.
These are people that you've tried to put into the robotic part of the company and then, the human part of the company just decided that's not an appropriate model anymore. It's actually pretty jarring.
Kevin: Like what's an example of that? What's a part of the company where you had that experience?
Kyle: Yeah, I don't know if there are any that I can really talk about. Maybe by the time this gets published I'll be able to.
Kevin: Then let me ask you another question. You knew from the start that you had this deliberate concept you wanted to create this cyborg company. What were some of the steps that you took early on, deliberate steps you took that you thought would help achieve this.
Kyle: So here's a good example, so we're a company that's built on developer community. So what I mean by that is software developers out in the world find our product, hopefully they enjoy it and they tell their friends about it. And we also have people here called developer evangelists whose job is to go pour fuel on that fire, right?
Get up in front of people and talk about some things we're building, get to know people in the developer community and ask them questions about their projects and build relationships with these people. And provide a point of contact with the company. So this is actually a great example of the cyborg company.
So there are a number of companies out there in our generation that are built in this way, with a developer community approach to how they grow. Often times, like very often, I've seen this pattern where the company gets certain level of success, and all the things I just described are very like, instinct oriented, right?
Kevin: Right.
Kyle: You know, how did Gandhi build his movement? How did Jesus build his movement? Well, they did it by talking about things with people, and getting up on stage and evangelizing. Even the word evangelizing has connotations of how do you build like a faith movement? And in a way,
Building a community oriented company is a faith movement.
Their faith in our company and in our brand and in our values is part of the message that they're sharing. They're not just sharing what this product does, but their faith in the product and in the company behind it. What I didn't say was these developer evangelists, they go up and their job is to get 16 signups per day or they're failing. Or their job is to get this many business cards, or this many intros or whatever it is.
Something that happened, that I've seen happen out in the marketplace with I'd say more than half of the companies that start like that is they get to a certain size, and then they bring in some very smart sales system builder. And the system builder says, "How do I know that those evangelists are working?" " How do I prove that what they're doing is actually going to affect the bottom line?".
I've seen examples where the evangelists get business cards that each have a little weird short code you have to type in. So they meet a developer and it's like, I'm going to give you this weird business card that you have to type in this weird URL so I get credit for meeting you. Which, it does have the advantage of making it more measurable, how well is each evangelist doing, but it has the invisible downside of making each of them way worse at their job, and super awkward and weird.
So it's really hard to actually do the work of converting a stranger into ally, when you're putting them in this weird system, but, the robots took over that company. So that's just how that company does it now, right? So this is one of those things where sometimes you can analyze something without having damaging effects on the phenomenon itself.
Sometimes you can't analyze it without damaging it. So it's better not to analyze it in some cases.
Kevin: I understand.
Kyle: I don't know, I forgot where I started talking about that.
Kevin: I asked you what deliberate steps you had taken to try to create the cyborg.
Kyle: Yeah, so one of the deliberate steps has been even when times where tough here, keeping the community team and making sure that they maintain the agency to do things their way. And believing in them. You know, the long term benefits of having a good community team are huge. If they have to prove what they do every month has an impact on the bottom line, they'll stop doing what they do.
They'll stop being a community team. So, the deliberate step, I mean, I've taken deliberate steps to make sure that the power structure of the company is such that I can make those decisions. I've taken deliberate steps even inside of those decisions, to make sure that that part of the company remains human. As human as possible. We're talking about human evangelism and brand building. Obviously that should be the most, a very human part of the company.
Kevin: Yeah. Okay, you sold me on that. Can you give me the flip side? What's a deliberate step you said to make sure something else ran smoothly in a sort of mechanized way?
Kyle: I think a really good example of this is, so we collect data from a lot of companies. Some of those companies are very small, have very little amounts of data. And some of those companies are very big and have huge amounts of data. And yet we service all of them with the same platform.
One of the issues that we had as we scaled was sometimes these really big companies would come onto the platform and they would use so much, they would consume so many resources that the small companies would have an adverse affect. Right, so this is kinda like, imagine you're in an apartment building and you're in an apartment building with some shared water resources.
So if somebody else is showering right above you, it might decrease your water pressure a little bit. But now imagine that person right above you is using 5000 times as much water. And then you turn on the tap and it doesn't work at all.
Kevin: Right.
Kyle: Until they're done with their shower. That would be broken. And we certainly have those cases where if people use maybe a million times as many resources as a smaller customer. So what that meant was a small customer could have a bad experience through no fault of their own, and you know in multi-tenant cloud companies they call this the noisy neighbor problem.
Like this laptop that keeps being noisy. And you know, it puzzled me for like a year how to prioritize those small customers. I wanted to prioritize those small customers, but how do I get the company to, besides just demanding it? It wasn't a huge problem, but it turns out, so this is a case where I tried influencing and inspiring.
I tried to go into the platform engineering group and saying, You know, it'd be nice if these small customers had a good experience and everything loaded really fast and the platform was really snappy for these small customers. It'd be great. You know, the response would be, one time in particular, the response was wait, but you just told us that we can't build a business on small customers and now you're telling us to take care of the small customers.
And I'm like, it's actually really complicated, because the small customers create brand impact that goes viral, and then the, you know, bigger customers find us through the smaller customers, or maybe they get acquired, the little companies get acquired by bigger companies and they bring us, all this stuff I'm trying to explain in this huge strategic map.
This is a very human way to do it, right? I'm going to use my context, I'm going to share it with you, so that you're intuition becomes educated with the same level of context that I have, and then I can trust you to make the right intuitive decisions 'cause I've given you all the context. That's a very human way of doing management.
Kevin: I appreciate it.
Kyle: Very human. Another way, and by the way, spoiler alert. That didn't work. Another way to do it, so we have this thing, and Kevin you know this, but for the sake of the audience I'll share it. We have this company-wide meeting every Monday called Metrics Monday where we share key metrics from across the company. And you may recall about a year ago, a new metric hit Metrics Monday.
A new thing that, you know, we check on these numbers and we share them company-wide. This new metric that hit was, "what's the platform's performance"? But it was divided up. "What's the platform's performance for tiny companies, for medium companies, and for big ones"? And if you go back to that first slide, you'll probably see that the platform performance for tiny companies was terrible at the time.
Well, I'm proud to say, I'm the one that got that metric inserted into that slide. That's what I think of as a robotic system, right? Here's a number, we have a process we're going to measure this number, we're going to show it to the company.
But it's also human because as the company sees it, they start asking like, Why's that one not, It seems like the small data volume customer, that should be easy, you know? That person doesn't use very much water. Their water should flow no matter what.
Kevin: Right.
Kyle: But by presenting it every week, I never issued an order. To my knowledge nobody ever issued an order. One of the engineers on our team, this nagged at him, and he stayed up thinking about it. And it weighed on him that this seems like a solvable problem. And then at some point a few weeks later, poof! He solved the core of that problem for those smaller customers by doing some better allocation of how traffic moves through the network or something.
I mean, it's technical how he did it, but the interesting thing here is that
The purely intuitive based leadership approach I tried to take didn't work, but a mechanistic one, like here's a metric actually worked.
Here's a success metric, we're going to measure it and we're going to report it. We didn't even go further and like set a goal. Like there are all kinds of different ways to mechanize this stuff.
You can set it as a metric just to report on it, you can set it as a goal, so that if you miss that goal, it's a failure and if you beat it it's a success. Or you can set it as a piece of compensation. You can set it as a quote "this is a part of the job description, you know"? So there are always different levels of power that you can exercised through these mechanisms. This was one of the lightest versions of that.
But it worked. And it worked after. I mean, I'm the CEO of the company, one of the founders, and I tried to use inspiration to get the same thing done, through a very sort of human network system, and probably could have figured out how to do it that way, but this worked really really well. This was expedient, by just putting a number on it.
So that's something that we still have to this day and if that performance every dips dramatically, we'll know about it and we'll be able to respond to it without any meetings. Without any leadership has determined we should fix "x" because the analytics are out there.
Kevin: And we solved it without deforestation it sounds like.
Kyle: Yeah, there's no deforestation that I know of.
Kevin: Okay.
Kyle: So there was a series of tweets about a month ago from a guy named Danilo who is one of my favorite Twitter accounts. And he's kind of riffing on AI and he says, So you know how humanity has feared AI arriving on the scene and destroying the world? Let's pick that one apart. This fear is rooted in two places, 1. Intelligence and 2. Motivation.
So in our fears AI can match how smart we are, but not have our values. That's like the thing we're afraid of, is that AI becomes as smart as people, but doesn't have human values.
Kevin: Right.
Kyle: So like terminator is a great expression of that fear, right? The terminator is a robot, doesn't have human values. Luckily in that storyline, spoiler alert, we infuse that robot with some of our values and he makes some of the right decisions afterward, but that could have gone badly. Super smart, super strong machine that doesn't share our human values.
Kevin: Become governor of California.
Kyle: Yeah, like that's a, super smart machine that doesn't value human life is terrifying to us, then he has his punch line tweet which is, guess what? We have good reason to fear these things, And
They're already among us. They're called corporations. Super smart machines designed with these different value sets. We know that profit motive is part of it. How you get to that profit motive doesn't necessarily need to value human life, right?
So if you could legally make a company called "Conquerors Incorporated" and then you comp everyone based on how much land do they conquer, they would start conquering. If you compensate them highly enough, that they can buy the weapons to go conquering and kill all the people that already are on that land, and then claim it as their own.
If you fund them well enough that they can get around whatever international laws they need to get around to make that happen, in theory, that could happen even now, even after everything's already been quote conquered. But of course, all the conquering that the west did through the dark ages and up to now, was mostly land that other people already had, so in a way this has kind of already happened.
Because the corporations, maybe the people who designed that system have human values, hopefully. Maybe the people acting in those systems have human values, but the corporation itself is sort of an unfeeling rule system for how do people get paid, how do they get compensated? How does the corporation get paid? What does it supply? What does it charge? Who does it buy from and what does it pay for that?
That's kind of a corporation, right? So this is an interesting thing where, a lot like AI, cause the idea with AI is we built that stuff. Like humans made Terminator. Those humans could be very, you know, pacifist, non-violent, love human life kind of people, but they built a thing that has rules and no values. And it has power and no values.
So Danilo's tweet, his tweet storm, really matches my thinking on this, which is that we already have those, they're called corporations. His conclusion is, you know, it's a smart machine, doesn't necessarily value human life. He references the Ford Pinto for instance. That corporations don't inherently value the environment.
So when Volkswagen cheated on its emissions rating so that it could get its cars through a system, that system was designed by people who were trying to protect the environment. Volkswagen looked at it very simply, like this is a test we need to pass to get our money as a corporation. Some of those engineers might value the environment, but the system of rules called the corporation maybe it doesn't value necessarily anything.
And he goes on, "why are we poisoning the planet? Why are worker's wages so painfully stagnant? Why are tax payers subsidizing big businesses?" It's because the machines have taken over. What he declares we ought to do is
Go inside of the machines. Human resistance should go inside of the machines, make corporations and infuse them with values.
So in a way, that's what we're talking about with a cyborg company. It needs to be as strong as a robotic company, but as human as a human company. So that's what we're trying to do.
Kevin: And we talk about that a lot.
Kyle: We do, that's no mistake.
Kevin: I mean, what are some of the values, we talk about our values deliberately and frequently.
Kyle: Yeah, I mean, we have our five explicit values, although that list will probably grow. One of them is empathy. That's definitely something that machines have a hard time with. Even the most advanced machines, they might be able to play Chess or Go, but they really struggle with empathy. Even by listing that as value and reiterating that, it has an effect, but I think we need to go a lot further than that.
We actually have to, it has to be encoded into the system. So for instance, we have a coaching program. Coaching program is an expression of empathy. It also allows people self empathy, in an hour we have our emotional intelligence introspection happy hour where we talk about our feelings with the group.
Or at least we think about our feelings and decide not to talk about them. But in any case, either way, it's still self empathy, right? That ritual, that routine is actually part of the corporate structure. That's actually part of the system and machine design of the corporation, is human empathy programs.
Kevin: I think before people come to work at the company, they have to, or we invite them to participate, right? To make sure they don't say, This is too much human stuff for me.
Kyle: Yeah, and you know, and it's good on them for noticing that. That's great because our number one value is introspection. This tweet storm, I really really liked it because it told me, It's exactly what I've been trying to live my career around, but I didn't know how to describe it so well. I've just been saying cyborg company for a few years. And never even explained it to anybody, I don't think. Until maybe right now.
Kevin: Certainly not to me until right now.
Kyle: But that's really what it comes down to is corporations aren't inherently evil anymore than artificial intelligence or robots, but they're not inherently good. So if we design them in such a way that the human part has a consistent role, and one of those roles, ideally, humans are in charge of robots. Humans can change the system design, but the system design itself incorporates humans.
You actually end up with this really interesting positive feedback loop, right? Like that cyborg with the robotic eye, I really like that example because the other eye's not robotic. The actual eye and the optic nerve are really really cool, and really useful, and so is the data, that, you know there's data, in theory your robotic eye's shipping data into your brain about, you know, all the stuff that's going on in the world around you maybe.
Maybe like it's got a facial recognition and when it looks at you it finds your Facebook profile or your credit score, whatever kind of robot I am. ( They probably already have those robots out there. But the human eye's there as well, and I think that's a really interesting metaphor for hey, evolution did some stuff right too, and the human eye's pretty useful.
And we can make robot humanoids that walk around that have two robotic eyes and no brain, but those, I don't see those competing with us right now. They're not, they don't seem to be as useful. Yeah, so I think I really like that metaphor and I think the idea of if these things are not inherently good or inherently bad, then they're really a technology. A corporation is a technology.
Which means it can be used well, or it can be used poorly. You know, ships can be used to get patients to hospitals, they can be used to ship goods to people. And they can be used to deploy militaries and conquer peaceful people and take their shit from them. The ship itself isn't good or bad, and I think that's kind of how a corporation is, so, building one with human values and a built in respect for human judgement, it's a big reason that we did this.
Kevin: Okay, I think I understand. I think I've got it.
Kyle: Does it make sense?
Kevin: I know what a cyborg company is now. Thank goodness because, I work at one.
Subscribe to Heavybit Updates
You don’t have to build on your own. We help you stay ahead with the hottest resources, latest product updates, and top job opportunities from the community. Don’t miss out—subscribe now.
Content from the Library
O11ycast Ep. #74, The Universal Language of Telemetry with Liudmila Molkova
In episode 74 of o11ycast, Liudmila Molkova unpacks the importance of semantic conventions in telemetry. The discussion...
Open Source Ready Ep. #1, Introducing Open Source Ready
In this inaugural episode of Open Source Ready, Brian Douglas and John McBride embark on a technical and philosophical...
Jamstack Radio Ep. #98, Open Source Data Integration with Michel Tricot of Airbyte
In episode 98 of JAMstack Radio, Brian Douglas speaks with Michel Tricot of Airbyte. This conversation examines data distribution...