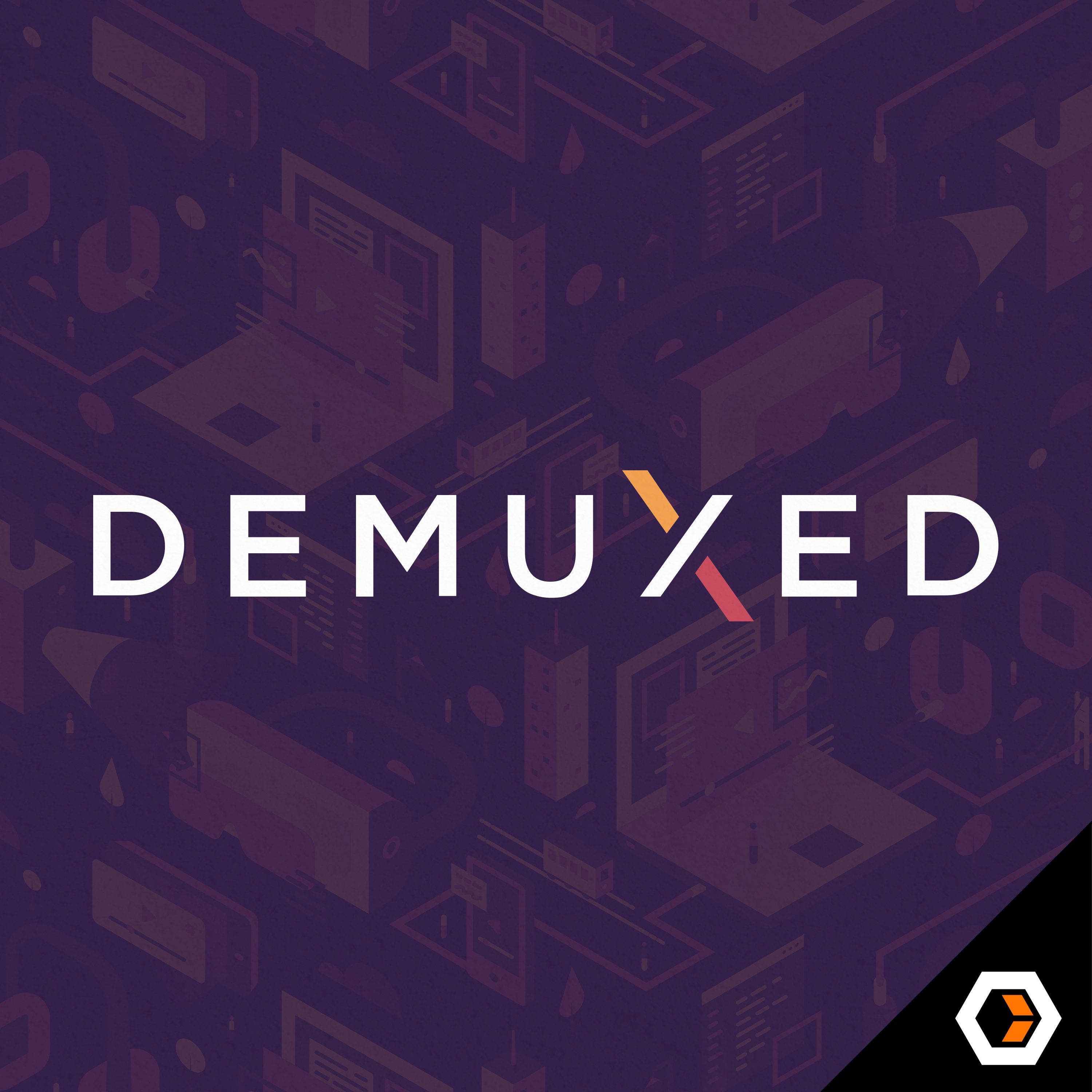
Ep. #12, Combatting Fake Video
In episode 12 of Demuxed, Matt, Steve and Phil are joined by Shamir Allibhai and Roderick Hodgson of Amber Video to discuss the growing accessibility to fake video creation, and what approaches engineers are taking to secure the public from misuse of this powerful technology.
Shamir Allibhai is the Founder and CEO of Amber Video, as well as Founder and CEO of Simon Says.
Roderick Hodgson is VP Engineering at Amber Video, and was previously an R&D Engineer at BBC.
In episode 12 of Demuxed, Matt, Steve and Phil are joined by Shamir Allibhai and Roderick Hodgson of Amber Video to discuss the growing accessibility to fake video creation, and what approaches engineers are taking to secure the public from misuse of this powerful technology.
transcript
Matt McClure: Hey, everybody. Welcome to another episode of Demuxed. This is the third one we've recorded in 2019. It's not even the third month.
We try not to mention time and place in these things, but it's the end of February. You guessed it. But--
Phil Cluff: How far have you moved since the last podcast, Matt?
Matt: OK. We lined up two in a row, so it's cheating a little bit here, but come on. We're lucky to have Phil in person here with us, and we've also got Shamir. Did I say your name right?
Shamir Allibhai: That's right.
Matt: OK, cool. We're going to talk about fake video and how they're working to combat it. We're going to talk about how Amber Video, which I said right this time, is helping combat fake video on the internet.
Shamir: Absolutely.
Matt: Thanks for joining us today, Shamir.
Shamir: Roderick is here as well.
Matt: Of course. I'm sorry. We have one remote and one in person. So, hi Roderick. Do you guys want to introduce yourself real quick and tell us a little about yourselves?
Shamir: Absolutely. I'm Shamir, I have been working with Roderick on thinking about fake video. The idea gestated in 2016-2017. We started thinking about fake news and what happens when this plays out to the Photoshopping of audio and video.
When we can't trust what we're seeing, what we're hearing. Roderick and I have been dabbling and thinking about ways to solve that problem.
Roderick Hodgson: I'm Roderick here, remote. Working with Shamir as he said on solving this problem, building products with Shamir, APIs, and testing out and deploying our solutions onto some real hardware and some real equipment and seeing how that can tackle the problem.
Matt: Where are you based out of, just out of curiosity?
Roderick: I'm usually in London. At the moment I'm in the North of England.
Steve Heffernan: Was there a specific experience they got you guys interested in the idea of fake video?
Shamir: There was three things that coalesced in my mind. One was the Black Lives Matter movement.
It was obviously really sad what was happening on the ground, but if you took a step back, I was fascinated by the movement that was formed by the simple fact that we now all have cameras in our pockets at all times and we can pull it out and record situations and counter other narratives.
Number two was Star Wars: Rogue One. Even if you were a fan of Star Wars, many fans didn't realize that two characters were brought back from the dead. One which had been dead for over 20 years, Grand Moff Tarkin.
Lucas Films did an incredible job and they went back to footage from the past, modeled it onto a 3D head, used a body double, and he looked like any other actor on screen.
Steve: I noticed, but my wife definitely did not.
Shamir: But I was also like, "The democratization of this technology has begun."
Third was all this conversation around fake news, and thinking about "What happens when you have fake audio? What happens when we have fake video? What happens when we can't trust visual and oral evidence?"
That's how we started thinking about, "This is going to happen. Will we regress as society back to our tribalistic roots?" We didn't want to go that direction.
Amber video was born from there and Roderick and I have been thinking through the problem on a twofold basis. One, authentication, and on the second which is detection.
Authentication is where we are fingerprinting recordings at the source level, on the hardware, and the detection would be "We weren't there at the source level. Can you analyze key frames, can you analyze the wave forms as signs of potential malicious alteration?"
Matt: We'll dig more into this later, but do you ever see that becoming somewhat of a game of whack-a-mole?
Shamir: The detection side, absolutely. The authentication side is the better way to go, it's just really difficult to get any SDK onto firmware or onto hardware.
The hardware upgrade cycles is a multi-year process, and you also depend on different partners to get there. The detection side is that stopgap.
Matt: I don't think you could have a conversation about fake video without talking about deepfakes . So, let's start there.
For those of you that aren't familiar, deepfakes was this subreddit where it came into the-- It was a project, but the subreddit popped it into the culture. Why don't you tell us about deepfakes?
Roderick: Yes. There's been in the last four or five years, there's been this evolution of what we call neural networks, which are a type of artificial intelligence modeled on biological systems.
The recent innovation that has happened has looked at how we can create and train different networks to try and compete against each other. What that allows is for these neural networks to be creative, to create new content from scratch.
Through that mechanism you can do some very interesting things. Because you could, for example, train a network to learn a particular art style and apply it to some other content, and that's often called style transfer.
You might've seen some examples online of people creating very creative and visually stimulating imagery from benign content, and it looks like a particular artist. Like Picasso, or it looks like Van Gogh or some other artist.
But that same type of technology could be applied, rather than having it to learn an artist's style, it could learn someone's face and apply that learning to some other content. Through that mechanism you could generate new content that never existed based on someone, an existing person's face.
That means you can make a person say something that they might have never said, or you can make them endorse something dangerous for their interests which could have a political impact, it could have economic impact, and it could have all sorts of far reaching consequences.
What's really interesting about looking at the deepfakes is that they are-- Because we're talking about artificial intelligence and we're not talking about special effects that are done in film, like we're talking before the Star Wars using the imagery of these actors and recreating content for them.
But we can think about what happens at the confluence of this ability to artificially generate on the fly, new content? Making people say something different, combined with all the information we gather from social media and all the reach you can get with social media?
You look at the confluence of social media and these deepfakes, and what does that mean that now I might receive a video with a celebrity or an important political person telling me something that taps into my deep insecurities, or might be fears, or my own political biases.
This idea of deepfakes is more than just fake imagery, it's about using artificial intelligence to trick people and all the impact that comes with that.
Steve: It's interesting. It almost feels like we're at a point of a perfect storm, where you have the social media networks in a place where their AIs have figured out how to serve you the content that's most interesting to you.
At the same time as is this new opportunity to create this fake content, and only takes a few seconds for a piece of content to go viral to your network and to reach a ton of people. It's a scary moment.
Shamir: Absolutely. We think about it too in this trifecta. One, we're recording a lot more video content and consuming a lot more video content. Two, now we can create and manipulate content with AI automatically.
Number three, we can now distribute that globally through the social networks which are really sophisticated in their distribution algorithms.
Phil: Jumping back to deepfakes for a little bit, last week the deepfakes repository on the face swap, particular face swap repository on GitHub-- There's a lot of drama surrounding that because GitHub made the decision to effectively censor that repository.
It's now the only repository on GitHub where you need to be signed in to view the repository. First ever time that's happened. GitHub do have historically certainly made some political decisions around things like that, but it's the first time they've censored but not got rid of something, and--
Shamir: It feels like sitting on the fence, though.
Phil: It does.
Shamir: I'm not sure this solves anything. It seems more like the optics of it .
Phil: I'm completely sure it doesn't. Especially given that forking repository makes it public and viewable by anyone.
Matt: Taking a step back, I don't think this does anything, because this repository means nothing. It's the fact that this technique exists.
For reference, for those of you that didn't follow this bursting onto the scene. I found out about this because of r/deepfakes which was a subreddit where people were using this technology and making fake porn. It would put a famous person's face on a porn actor or actresses face.
Shamir: It's always Nicolas Cage.
Matt: In the repo, it's Steve Buscemi's face on Jennifer Lawrence, which is horrifying.
Phil: Terrifying.
Matt: People were obviously really freaked out on one hand, on the other hand r/deepfakes took off. Obviously these are fake videos, Reddit made the decision to just pull the plug on it, so r/deepfakes is banned.
Banning these things doesn't do anything, it's still out there, people are going to just write new software. You can't ban that the software exists.
Shamir: Now there's deepfakes as a service. There's websites where you can upload the target video, and then upload the faces from different angles, the face that you want to impose or transpose to the target video as a service. $5-6. This touches on something.
Technology is not inherently good or bad. It is people who will use it for good and bad reasons.
Steve: How would this be good? How would you use this for good?
Shamir: Definitely this would be used in Hollywood. Definitely.
Steve: For entertainment?
Shamir: Absolutely. There are deceased actors, and the estate of which will give permission to a studio who will say "We want to bring back this story, we want to tell a part two. The actor is obviously no longer here, go back to old footage."
I could totally see them using that. This has been used in commercials without the permission of the estate of the actress.
Steve: Do the actors get royalties when they're--?
Shamir: Those are real societal questions we'll have to face. No, not right now. The way in which some people read the law there's ways to parse this. The likeness and similarities of somebody, so maybe there is something there and there will be grounds for losses.
But this is such a nascent field. A lot of these questions, b esides the real ethical questions, have not even been worked out. We're still trying to grapple with getting our heads around this, and from--
If you are on the technology side you're more able to see how this is going to be a looming challenge society-wide. Will we ever be able to fully block this?
I think it's really hard and you just need to create awareness, and some of those, this conversation around fake news has done that. But there are blogs being passed off as news which are intended to sow distrust, for example.
We all should have a more skeptical lens when we read posts on social media. There is an awareness, and the same will happen with video and audio at some point.
Matt: It's funny that we're a far cry from when the worst examples of fake news were when it was just annoying that your mom kept sending you Onion articles thinking they weren't satire.
Phil: That's definitely happened.
Matt: There's another project that blew up on Hacker News recently called This P erson Does Not Exist, which was immediately followed by This Cat Does Not Exist."
Phil: That was horrifying. Did you see some of the stuff on there?
Matt: Nightmare fuel. It was like a partially dismembered cat-- Not in that sense, but legs not in the right place. It was freaky.
But this person does not exist is astonishingly good, so it's interesting the flip side of deepfakes , where taking Steve's face and putting it on Phil in a video-- The Phil in the picture isn't a person, he doesn't exist.
Phil: It's interesting. This Person Does Not Exist was down last week when I was writing the outline for this podcast, the website was down and I checked it now and it's back. But it's back with a big banner at the bottom that says "This isn't a real person, we generated this using a generative neural network. This is not real anymore."
I found that interesting that they felt the necessity to take it down, but then also to say "Yes it's back but this is information about how this isn't real, this is not a real person." I doubt the cat one needs that.
Matt: It says, "Don't panic. Produced by a generative adversarial network-- Don't panic, learn about how it works."
Shamir: While this isn't an adversarial network creation, but if you've seen little McKayla on Instagram she's a synthetic character who seems to have this wonderful life on Instagram.
It taps into an Instagram culture of putting your best foot forward and showing this glamorous and great life, and the production company in Hollywood have tapped into that and created this synthetic character. Incredible.
There's no warning, so if you look at first glance you're like "This is incredible. This is an amazing person's life,"and then you realize something's off about the lighting. Then you get through that portion and you're like, "Something's not right here."
Phil: Of course on both of these, the interesting thing is it's about sample size. It's about data training sets as well. This Person Does Not Exist works really well pretty much because it's got a great training set behind it.
This Cat Does Not Exist was not well trained. There was literally photos from I Can Has Cheezburger in there, which is why it sometimes generates random indecipherable text at the top and bottom of the images.
Shamir: This is why Nicolas Cage is in all the porn videos, because there's so many videos of him from so many different angles.
Phil: I had never thought about that.
Shamir: He's got a large body of work.
Matt: It's terrifying that is the floor for this working so well, because we produce so much video as a society every day.
Shamir: But the amount of training data is getting less and less. The amount that we need to create these faces is getting less and less. Right now it's Nicolas Cage, at some point it could be 100 hours of somebody, and it will go down to 10 hours.
Steve: So then the software needed to detect these things needs to be at least a little bit better than the software that's already being used to create the things.
Shamir: Absolutely.
Steve: How do you do that?
Shamir: It is a parallel to antivirus software, where you are taking one approach, and then the bad actors will figure out what approach you are using and their content is getting flagged, and they'll come up with a new approach.
You'll try to stay either really close, one step behind or try to stay one step ahead, and then you're going to play this cat and mouse game or this whack-a-mole game.
Steve: AI versus AI. Basically, Terminator 2.
Shamir: Absolutely. This is really an adversarial network. Exactly.
Phil: You're using adversarial networks to detect? You're training it on things that are fake, and then things that are real and then comparing them. That's absolutely fascinating.
Roderick: It's a very interesting analogy looking at how adversarial networks work. As you say, that's what we would be doing, because we are designing non-professional intelligence to detect.
Which is as we said how they work internally, and the main distinction is that the feedback loop is an instant when you're creating a generative adversarial network.
Feedback loop is instantaneous, and that's how you're continuously iterating and creating so quickly such amazing content and realistic looking content to humanize.
We're looking at it through the medium of social media, that's our feedback loop, but we have an advantage there as a detector because we can see all the content that they're generating and they don't know what we've necessarily classified it as.
So that feedback loop is disconnected i f we don't give out all the information about what we detected was wrong about this video, it comes down to how we score them and how we report results.
But we do, it's not a strict arms race. It is scaled in the favor of the detector, because we have control over the feedback loop on our side. But then also because the adversarial network that's generating the fake content needs to trick not just the detector but still needs to trick the humans.
It's not a case that you can keep feeding back, keep tweaking things more and more until you reach something that looks like it's a gray blob. It's like, "OK, maybe that's not fake."
But you still need to trick the human. You still need to convince them that this does look like a person, and then also treat all the little things that we can detect that are telltale signs of tampering.
It's so much harder on the side of the faker as long as there is that line of resistance and as long as there's someone here to defeat it.
Shamir: I want to say one asterisks on that. There's going to be this period where that's correct until the point where you can generate something completely from scratch.
Right now it is a face to face, like Jordan Peele is recording his mouth facial movements and that's being transposed onto Obama's face. Obama is saying stuff and moving his mouth in that sync.
What if you can generate from scratch a brand new Obama video that's hard, really hard to detect because there's no artifacts and there's no elements in there? No interesting cuts on the wave form?
That's where this becomes really hard is when you can completely synthetically develop something from scratch.
Now it's hard to look at what you're trying to detect there.
Phil: Did you guys see that video where Obama kicks down the door of the White House?
Shamir: I didn't see that, no.
Phil: That's one of my favorites. He gives a press speech, and then he--.
Steve: Is this real?
Phil: No, it's fake. It's a fake video. It's the usual, "Now I got to go, man." He just walks out and someone has edited it so that he kicks the door down. It's so good, and it's really convincing as well.
Steve: That's the other side of it. We might have the technology to detect these things, but there's a long period where people don't even know that exists and that they should care about that.
Shamir: Absolutely. From our mind we have been told since we were very young that seeing is believing. We all have a certain recognition that text, even journalism, has a layer of bias.
What we see, we believe it to be true. What we hear, we believe it to be true. Just like those words were spoken or that action in that video is true, that no longer will be the case, and yes that is a real awakening that needs to permeate society.
Especially if we don't have the technology to address it, or we don't have the systems to remove it or flag it.
Steve: Let's all just becomes skeptics. I'll join the--
Shamir: Let's do it.
Steve: Moon Landing conspiracists, and Flat-Earthers .
Phil: No.
Matt: What are you trying to say about Flat-Earthers?
Phil: Let's touch a bit more on the other side of the problem, which is a situation where the way something has been edited is more impactful. Like it might not be a regenerative neural network.
It's just footage that has been manipulated in the way that it has been edited, and without wanting to get too political, the White House press secretary--
Shamir: With Jim Acosta and the karate chop.
Phil: This is a real thing that happened last year from a government of one of the biggest countries in the world.
Matt: The aid's arm did fall off.
Phil: This is something that really happened in Western society, in major news outlets delivering us. This is the second bit we've got to think about.
Shamir: If for example C-SPAN had used Amber Authenticate and we were hashing at source at the record level, then we could have easily proved that a few frames were removed from that video that was posted on Twitter to make it look like the sequence of the events was a chop rather than as a casual swipe.
But the point is, yes. This applies to almost any video, is that the editorial choices people choose to make, no software will solve that. People for example talk about "OK, I'm not sure this is a real use case, that fake news and the news industry is under duress. I'm not sure this is a real genuine issue."
But in whatever people understand of fake news they say, "OK. Yes. You should apply your technology to CNN, for BBC, for whomever. Because people don't trust the news."
I'm like, "Totally. We can authenticate footage, but the choices people use in the edit room we can't do anything about that. If they choose to show just a clip of a speech that makes it look like he or she said something totally different, there's nothing we can do.
The footage is authentic. They made an editorial choice and you see this between MSNBC and Fox News. Again, not to take this overly political, but you can see those choices are being made in partisan news media.
Matt: Can we take a step back? I know exactly what regenerative neural networks are, but for everybody else, can we take a step back and talk about generative-- Or, not generative. Adversarial. Can we talk about what that is? What are the other different types of neural networks, and why is this one particularly well suited for this problem?
Roderick: The way adversarial neural networks work is because they take that issuative approach of having a generator and a discriminator, the way they work is to attempt to fool another artificial intelligence.
What that means is that if you're building an artificial intelligence, you might already be one step behind, because it's specifically been designed in a way that fools another AI network that's there to try and detect certain things.
What it's trying to detect depends on what you're trying to create, so if you're trying to create a piece of artwork, it might be detecting if it matches an existing style of art.
But if you're trying to create a likeness of someone, the way an adversarial neural network works is it's first generating content and then there's another train network saying "Does this look believable as this politician?"
Looking at it of that's more or less how these systems are set up, and that makes it very dangerous because they're, I suppose, in that way designed to defeat a discriminatory mechanism.
Whether that's another AI or whether that's a human, and that's why for us it looks like the person is saying that content has been designed to trick. That's how it works.
Where the origins came from--we're using this to be creative and use it to generate something that no one's seen before. But of course when it's applied in this way, it's designed and used specifically to trick and sow mistrust and to, depending on how it's applied, you either have a strong political impact or a strong legal impact.
Whether that's tampering with evidence, or making people believe certain people said something different, or whether that's an economical situation, discrediting a corporate executive or anything like that. That's what then makes it dangerous in society at large.
Matt: Just so I'm clear here. The way this works is, I've trained a neural net that says "This looks like a person."
Phil: Real face, fake face.
Matt: Real face, fake face. Then I trained another neural net that puts faces on other faces, and then that neural net continuously tries to put faces on faces until the other net says "OK. That was a real face."
What you are talking about doing is then adding a third neural net that is somehow smarter than the second neural net.
Roderick: Yeah.
Matt: That's wild. My mind is blown.
Roderick: The thing is, we don't just need to rely on using a neural net, and we don't just need to rely on doing what those two are doing. Which is making imagery that looks like something and assessing whether or not it looks like--
We don't need to say "OK, this one is trying to make it look like Barack Obama. We don't need to create another network that says 'Does it look like Barack Obama?' We can create a network or other types of AI that says 'Does it look like there's been some tampering? Does it look like the focal lens of certain cameras don't match?'"
There's all these different tells, these audio cuts, all sorts of different little bits and pieces that on their own might not mean much. But when we put them all together the way that neural networks work, which is combining loads of different pieces of evidence to create a conclusion, then we can derive an assessment on that basis.
It's not just looking at the mechanisms that are used within the systems that are creating the content, but it's looking at the medium itself and it's looking at the context that it's presented in all sorts of other things.
Shamir:
Right now the bar is, can you create a video that is indistinguishable to any other, to the human eye? Can we create a fake here that fools a human?
Because there is no software out there. You can you can post it on YouTube, you can post it on Facebook, Twitter. We're trying to provide that middle layer from when it's posted to when it is consumed, and being able to say "This is something that has been maliciously altered."
T hat's an analysis on the key frames on audio tracks and trace elements that have been left behind by the adversarial networks.
Steve: Interesting. Even in the video file construction itself, not just the imagery?
Shamir: Right, and audio track as well.
Phil: The audio track is really interesting because in a slightly different space, in a different industry, one of the telltale signs that someone has faked a speed run, a particularly good speed run, is audio issues.
Because of the way, I'm sure as we all know, AAC works and audio frames and things like that, most modern editing software and in fact all modern editing software and non-linear editors will end up with tiny audio gaps that are detectable.
This is how a lot of fake world record speed runners, videogame speed runners have been caught, by looking at the audio analysis.
Steve: Are you saying this is going to result in a lot more Quicktime movie edit lists? Because those are a pain to deal with.
Phil: I know, I hate those things.
Matt: This is obviously, especially with the rise of deepfakes and this bursting into the cultural lexicon here recently. I'm sure we have a few, there are a few players in this market talking about how to solve it, and I know I have tried to find it.
I can't find the one that I was thinking of, but I know that there are some block chain projects out there around verification.
Shamir: We're doing that with a first product called Authenticate.
Matt: Really?
Shamir: Yes. Authenticate is hashing at source, and those hashes or fingerprints are written to a smart contract and in our demo we're using Ethereum. You can download it for example, the application on the App Store, the Apple App Store.
When you're recording through the application it's hashing, and then it submits it to the miners and the Ethereum miners to write to the block chain and to write to a smart contract.
It's creating a providence record. When I then share that file with you, it is rehashed compared to the hashes in the smart contract, to verify the authenticity. If it matches then you know since the timestamp record in the block chain, nothing has been altered.
Without going too deep on block chains, it obviously has to be one where it's trustworthy. It's decentralized, there's a whole bunch of block chain theory in this, but it's effectively an immutable but transparent record.
The articles in Wired and Axios were really about Amber Authenticate preserving due process. In the event of a shooting, the footage from a police body camera becomes evidence, and that evidence gets shared with numerous stakeholders.
Some whom are there to serve as checks and balances and should not be trusting anyone else, and just saying "It's the police, let's just trust them." Or the police shouldn't just say-- What block chain does is create that trustlessness.
It's hashing on the police body camera, writing it and submitting it to the block chain, so when it goes through a prosecution or a judge, jury, activist group, media, general public.
They all want to verify the authenticity of a recording, they don't even have to trust Amber. They can hash the clip themselves and look at the transparent record on the block chain. What we're hashing is novel.
Typically hashing is not new, but hashing, the specific characteristic and audio and video files, allows us to clip video. For those clips to maintain a linkage, a cryptographic linkage to the parent recording.
For example we apply this to the media industry, a video news piece might have 10 b-roll shots and 14 soundbites, each one of those clips can be authenticated.
Phil: You can tell the order they come in as well, right?
Shamir: Exactly.
Phil: So if you take audio from way over down deep and then put it back--
Shamir: Exactly.
Phil: That'll be understood, right?
Shamir: Yes. Because it has a providence record. Exactly. It has that link to the parent recording. Even when clips are combined, our software still works, Amber authenticate. That's really trying to counter this whack-a-mole on the detection side.
Matt: It's refreshing to hear such a legitimate use case for that.
Shamir: We get that a lot to be honest. Like, being in the block chain world. I'm like, "Oh my God, do I have to use this?" But we get that a lot, and we're like, "Oh my god. This is a genuine use case for block chain."
Matt: It's understated on your site. I just went back and it's right here under Authenticate, but I'd missed it when I was reading through earlier.
Shamir: Right.
Phil: It's super cool.
Steve: It's interesting that you almost have to go that route otherwise Amber becomes a source of potential issues. That's cool.
Matt: Obviously there aren't other good use cases, but this is so cut and dry. We need an immutable trustless.
Shamir: Absolutely. Especially when people's lives are on the line in prosecution, going through court. I don't want to rely on probability or trusting another party--
Matt: API is 500-ing right now. "Guilty."
Phil: That's an interesting question. Anyone can hash-- Is the idea that anyone can verify the hashes? So you have an open published spec for how to implement that hashing algorithm?
Roderick: We have a standard way of doing the hashing. The spec itself is not published yet, this is something we're looking into. But what we're doing is a standard repeatable way that we expect the users to then do on their own device, on their own own system and their own mechanisms.
So, Absolutely. It's the idea that we want users to gratify the hashes themselves on the content that they're consuming.
Matt: Help me walk through the scenario where there's a more complicated process required than, "I'm a police officer recording body camera footage. That file is hashed, that hash is put on the block chain somewhere, and now we have the video."
Wouldn't that alone almost be enough? Because then you could just say, "OK. You only showed me a piece of this video because it doesn't match the hash, or clearly you edited it because the hash is different."
Shamir: The specific use case for law enforcement is a police man or woman may have been on shift for one hour before a situation occurs, and that situation lasts 15 minutes. Legally you're only allowed to share the footage of the event in question.
You only want to share, or you may only be allowed to share, 15 minutes of that longer recording. Now when I share the 15 minutes with Amber Authenticate, you would know that nothing has been altered other than a reduction in length.
The other thing for law enforcement is redaction. Legally, you have to redact faces of people who weren't critical to the scene in question. The same hashing approach could apply there.
That's what we think, when it comes to creating trustlessness in multi-stakeholder situations, Amber Authenticate is what we're advocating for. There are times though for social networks, where your videos are being uploaded, you weren't there at source.
How do you do that? That's the second part, which is detection. We talked about it as this truth layer, or SSL for video, and we're trying to combat it on both fronts. Authenticate and detect.
Matt: What's next for y'all?
Shamir: We're trying to see whether this is-- It's a sad point, but does anyone care about truth? We're really trying to explore that.
Phil: You have to leave that to the end.
Shamir: We talk about truth a lot and we repeat it often times, I wonder whether we would care about truth as much as we say we do. It's been the insight.
Steve: At least, the true truth. Not just my truth.
Shamir: Exactly. That's been the insight of this presidential administration, and knowing that maybe there's a gap between what we say and what we feel.
We're really trying to figure out whether people do care about truth enough that they're willing, that this product's important, and we're figuring that out.
We're an early stage startup trying to explore who has economic consequences where they need to know that this is absolutely unequivocally truth, and they need products to make sure that in a world of deepfakes they can say with certainty "This is genuine."
I hope I didn't end it off on a sad note there.
Steve: No, it's real.
Matt: It's a really similar discussion that I see happening all the time around privacy. People like to pay a lot of lip service to privacy, and how much they value their privacy and care about privacy, and at the end of the day if they have to pick between paying for something or taking ads--
People have overwhelmingly kept using products and services despite concerns over privacy, because they can, and people keep visiting websites advertising on them because the entire internet economy runs on a lack of privacy. It's a pretty easy parallel to draw there.
Phil: Obviously I'm on the receiving side of GDPR, every single day and every single time I open a website in England I get a pop up that says "First of all, can I give you a cookie? Yes or no? Second of all, do you consent to GDPR? Yes or no? Or, go opt out."
The interesting thing is the wording of the opt out is pretty much always the same. It's, "You're still going to get ads and you're going to get the same volume of ads, but they're not going to be tailored to you. "
Which is interesting because that's done to educate users on understanding that privacy situation where they wouldn't otherwise immediately understand it.
Shamir: We're seeing definitely interest in government, in multi-stakeholder situations where there's different parties and they absolutely serve in the checks and balances situation. Like what we said with law enforcement, so we're definitely seeing that.
Even from law enforcement's perspective, there will be videos where they are manipulated to make it look like law enforcement was bad. So it's helpful for each party to know this is truth, this is what's genuine and what's real.
We're seeing interest there, from a startup perspective, it's interesting thinking about how to work with the federal government who operates on a different timeline to when you're here in San Francisco. It's like a black box over there, and then timelines are much different than what we're used to here.
Working on that pace and timing has been an interesting experience for us on the detection side. It's clear that social networks are increasingly, they felt that they were behind on fake news. They need to get ahead of fake video.
It's clear that there's interest in a detection software, and they themselves have teams who are looking at this.
Steve: Why is it called Amber Video?
Shamir: Good question. So, the linkage is to Jurassic Park.
If you remember the mosquito bites the dinosaur and sucks out the blood from the dinosaur, and then gets stuck in amber for all time and preserved for all time, until thousands of years later human miners dig it out and see the mosquito and the DNA of the dinosaur in the in the blood in the mosquito.
Phil: The interesting intricacy there is they augmented that DNA and filled it in with frog DNA in the movie, because the DNA-- Also in the book, because the DNA samples were supposedly eroded.
That's interesting because that even plays into the idea that it's not completely the dinosaur DNA. It's brilliant. That's a clever name.
Matt: Thank you guys so much for joining us. By the time this is released we'll have hopefully a website up and everything, but Demuxed 2019 is officially set. It's October 23rd and 24th, it's at the Midway here in San Francisco.
Phil: That venue, though.
Matt: It's going to be amazing. There's room, there's going to be more comfortable chairs, I promise. You too could even sponsor those chairs.
Steve: And the cookies.
Matt: And the cookies, so we don't get those despicable cookies from last year.
Phil: Calling them cookies is an offense to every other cookie.
Matt: If you're interested in sponsoring, just e-mail me at Sponsor@Demuxed.com. Speaker stuff will go up over the summer. I hope you guys submit talks, this is a really prescient topic right now.
Phil: Don't forget if you want to be part of the podcast, e-mail Podcast@Demuxed.com and let us know.
Matt: Thank you so much, Shamir and Roderick, for joining us today. This was really great.
Shamir: Awesome. Thanks for having us.
Roderick: Thanks a lot.
Subscribe to Heavybit Updates
You don’t have to build on your own. We help you stay ahead with the hottest resources, latest product updates, and top job opportunities from the community. Don’t miss out—subscribe now.
Content from the Library
Jamstack Radio Ep. #147, Secure Local Dev Environments with Chris Stolt and Ben Burkert of Anchor
In episode 147 of Jamstack Radio, Brian speaks with Chris Stolt and Ben Burkert of Anchor about securing local development...
O11ycast Ep. #69, Collecting Cybercrime Data with Charles Herring of WitFoo
In episode 69 of o11ycast, Jess and Martin speak with Charles Herring of WitFoo. Together they dive deep into the world of...
Jamstack Radio Ep. #142, Decoupled Authorization with Alex Olivier and Emre Baran of Cerbos
In episode 142 of Jamstack Radio, Brian speaks with Alex Olivier and Emre Baran of Cerbos. This conversation explores tools for...