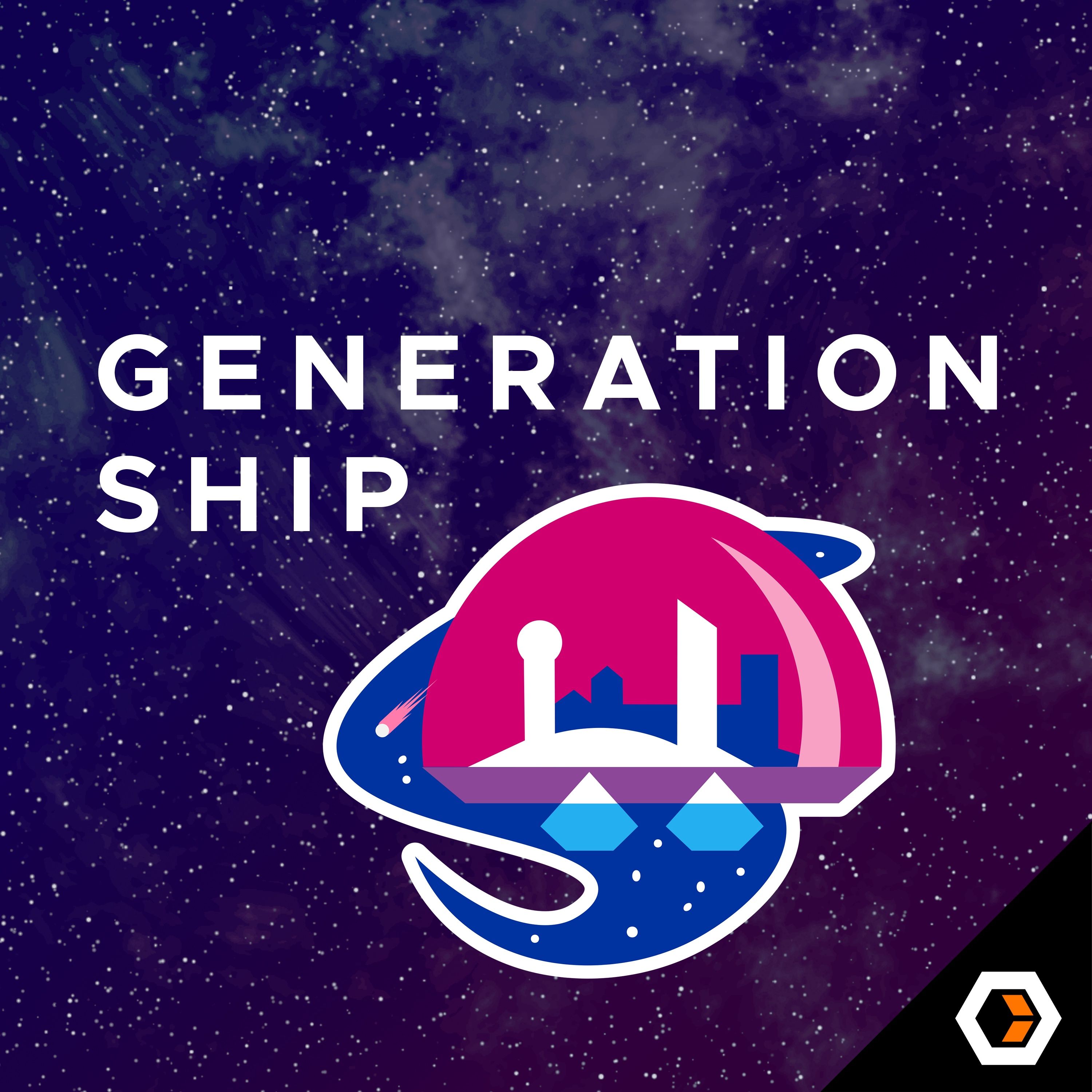
Ep. #10, Ethical Benchmarks for AI with Dr. Marie Oldfield
In episode 10 of Generationship, Rachel Chalmers and Dr. Marie Oldfield explore ethical benchmarks in AI. This conversation sheds light on professional accreditation for AI practitioners and offers unique insights on mitigating societal risks while building better AI models. Additionally, Marie shares lessons learned from her expansive career journey across Government, Defense, Legal, and Tech.
Dr. Marie Oldfield, CStat, CSci, FIScT, SFHEA, APAI is the CEO of Oldfield Consultancy, Executive Board member at the Institute of Science and Technology (IST) & Senior Lecturer in practice at LSE.
Dr. Marie Oldfield is a recognized, published AI and Ethics Expert with a background in Mathematics and Philosophy. Marie is a trusted advisor to Government, Defense, and the Legal Sector among others. Marie works at the forefront of Ethical AI, driving improvement and development. Marie is the creator of the AI Professional Accreditation that benchmarks practice in AI and interdisciplinary modeling.
In episode 10 of Generationship, Rachel Chalmers and Dr. Marie Oldfield explore ethical benchmarks in AI. This conversation sheds light on professional accreditation for AI practitioners and offers unique insights on mitigating societal risks while building better AI models. Additionally, Marie shares lessons learned from her expansive career journey across Government, Defense, Legal, and Tech.
transcript
Transcript coming soon.
Content from the Library
MLOps vs. Eng: Misaligned Incentives and Failure to Launch?
Failure to Launch: The Challenges of Getting ML Models into Prod Machine learning is a subset of AI–the practice of using...
From Labs to Launch: Stories from GitHub Copilot
Discover GitHub Copilot's launch strategies and actionable insights to apply to your product, and navigate the ever-evolving...
Generationship Ep. #2, Putting LLMs to Work with Liz Fong-Jones and Phillip Carter of Honeycomb
In episode 2 of Generationship, Rachel Chalmers speaks with Liz Fong-Jones and Phillip Carter of Honeycomb. Together they explore...