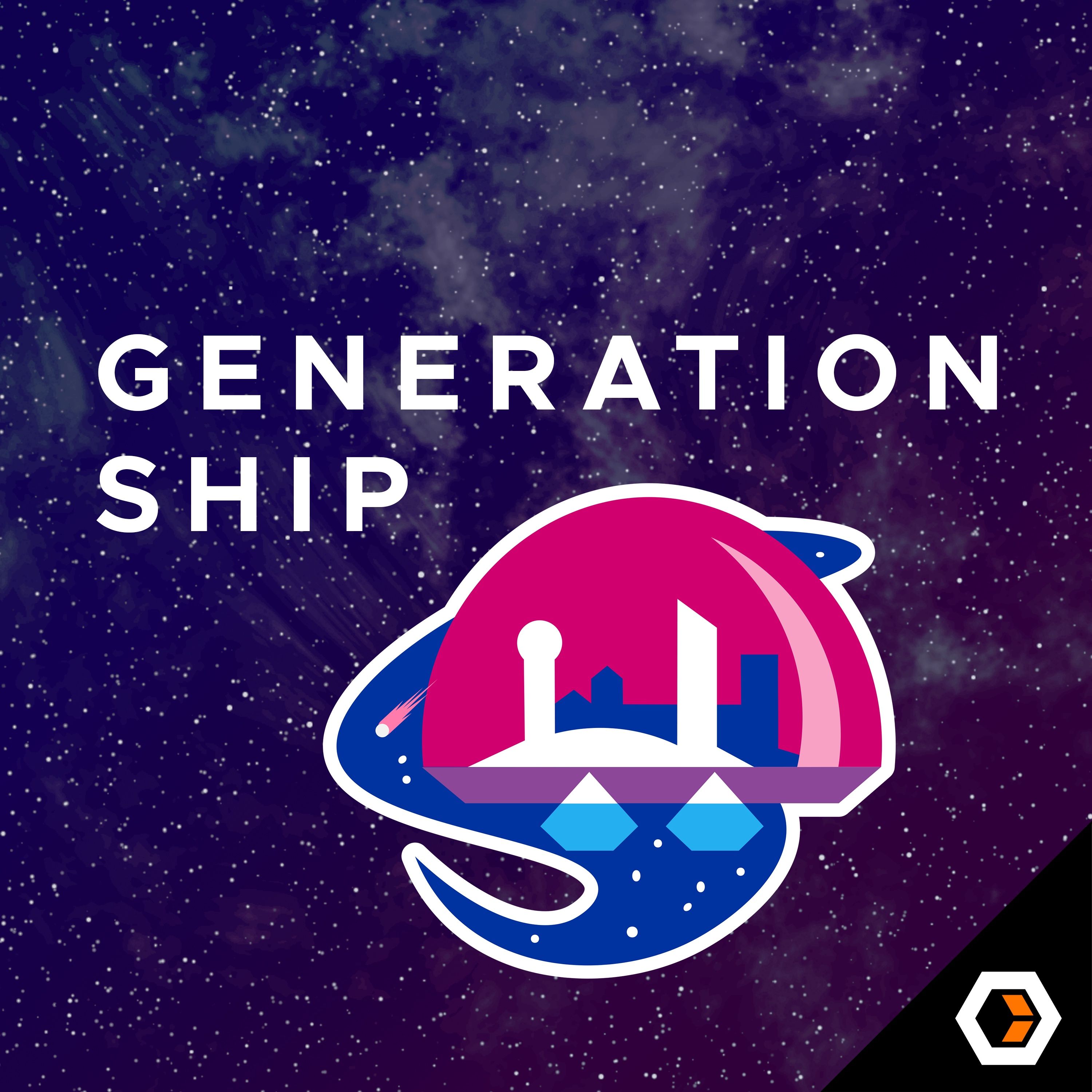
Ep. #21, Keep Up! Featuring Dr. Maia Hightower of Equality AI
In episode 21 of Generationship, Rachel Chalmers is joined by Dr. Maia Hightower of Equality AI. Dr. Hightower dives into the transformative potential of generative AI in healthcare, discussing both its promise for underserved populations and the risks of algorithmic bias. Discover how responsible AI can drive health equity and the importance of diverse perspectives in shaping technology's role in medicine.
Maia Hightower, MD, MPH, MBA is the CEO and Founder of Equality AI, and former EVP, Chief Digital Transformation Officer at University of Chicago Medicine. Dr. Hightower is a leading voice in the intersection of healthcare, digital transformation, and health equity.
In episode 21 of Generationship, Rachel Chalmers is joined by Dr. Maia Hightower of Equality AI. Dr. Hightower dives into the transformative potential of generative AI in healthcare, discussing both its promise for underserved populations and the risks of algorithmic bias. Discover how responsible AI can drive health equity and the importance of diverse perspectives in shaping technology's role in medicine.
transcript
Rachel Chalmers: Today I am so excited to welcome Dr. Maia Hightower onto the show. Dr. Hightower is the CEO and Co-founder of Equality AI and the former executive vice president and Chief Digital Technology officer of the University of Chicago Medicine.
She's an expert and nationally sought speaker in responsible AI and at the intersection of digital technology with health equity, diversity, and inclusion.
Equality AI is on a mission to end algorithmic bias in healthcare. Data scientists are the newest members of the care team. Equality AI empowers digitally enabled care teams to achieve health equity goals through responsible AI and tools to develop algorithms that address bias, fairness, and performance.
Dr. Hightower, welcome to the show. It's so great to have you.
Dr. Maia Hightower: Oh, so wonderful to be here today. Thank you so much for having me, Rachel.
Rachel: It is so clear that generative AI has the potential to extend healthcare to underserved populations, and it's equally clear that there are risks involved. How do you think about the potential for this technology in healthcare settings?
Maia: Yes, I definitely agree with you a 100% that the power and the potential for AI really to revolutionize and address some of our most challenging problems in healthcare is real.
And that across the different things that we do in healthcare, provide care, administrate healthcare, manage risk, all of that is possible to provide better care for underserved populations to provide better care for everyone.
But at the same time that we think of the upside, you always have to think of the risks and making sure that we are mitigating those risks, that we're identifying them. And the risk when it comes to generative AI is that it works for some and not everyone, that it provides benefit for those that are well represented in the training dataset where it provides a lot less benefit for those that are underrepresented in the training dataset.
And I think it's important to understand that in a healthcare, healthcare data training dataset is skewed in a very interesting way. It's not in the same way as say, you know, social media training data sets or the world wide web. It really skews to older, sicker, and those that seek care frequently.
And so those that are underrepresented include those that seek care less often, that may have systemic barriers to accessing care. Could be geographic, rural versus urban. It can be age. Young people are underrepresented in healthcare training data sets.
It can be by disease. If you have a rare disease, you're underrepresented in a treating dataset versus something that's more common, like cardiovascular disease or diabetes. And so healthcare is special in that we serve everyone.
And in order for generative AI to provide benefit for everyone, including underserved populations, we really need to make sure that we're both, identifying risk, risk for widening disparities, and making sure that the technology really works for everyone.
Rachel: That's a really interesting perspective because I'm reminded of that old logical suggestion that, you know, if you see hoof prints, don't think unicorn, think horse, but of course if you have a child with an orphan disease, it is a unicorn.
And the tendency of generative AI in its spicy predictive text capacity is to immediately go for the horse. But sometimes we're dealing with edge cases.
How do you accommodate that in a medical paradigm that's going to be harnessing generative AI?
Maia: Yeah, absolutely. And you know, there's more to AI than generative AI.
We still have really good predictive models, like computer vision, that also have provide incredible opportunity. When it comes to the rare cases, you really have to make sure that models are fit for purpose, that they really are being trained for a specific problem.
And when it comes to rare diseases, there's actual methodology to be able to augment the signal of a rare disease to make sure that you really are developing a model that's going to help identify those needle in a haystack type situations.
And so, you know, from a data scientist or from an AI research scientist perspective, making sure you're really leveraging the entire body of AI methods targeted for what you are developing a model for.
And then for a consumer, then sometimes it's the healthcare system as the consumer the buyer or the distributor of a model, making sure that when it's deployed that that fidelity to the actual intended design and usage of the model is maintained.
Because sometimes, you know, there's this tendency: You've got this tool. It seems to work pretty well for correlated cases, but it's actually in those correlations where it may not actually be as suitable for that particular problem, it's just correlated, that you may be using it inappropriately.
Rachel: Oh man, I read about one of these the other day. Somebody was training a model on detecting skin cancers, and it turned out that the correlation it was turning out was, is there a ruler in the image?
Because if there was a scale in the image, it was much more likely to be from a medical setting, and, therefore, much more likely to be cancer.
Maia: Yeah, exactly.
Rachel: It was amazing.
Maia: Yeah, great example. It's like, okay, all you have to know for skin cancer diagnosis is stick a ruler in there. If you're measuring it, it must be bad.
Rachel: And your point about models' fitness for purpose makes me think about, I remember when ChatGPT was just starting to explode and everybody was writing about it, and one very serious bro online actually used it for like, talking about his career and talking about some of his emotional problems.
And it was remarkably effective. And he said, "I guess this is how people feel about therapy." And I was like, "Yes, everyone should do therapy. You're right."
But I also thought about the risks of that. And of course, you know, if you give it the right prompts, ChatGPT will encourage you to jump off a bridge.
We already had therapy tools like Woebot, and the difference was they had these much stricter guardrails because they understood the use case, and they understood what the risk model was. Is that a good example of what you're talking about with the model's fitness for purpose?
Maia: Absolutely, especially for the chatbots where when they're being deployed for a healthcare specific situation, and there's a lot more rigor when it comes to providing the chat bots that are deployed within a healthcare system, but far less rigor when a chatbot is being deployed, say on a website for a health advocacy group.
And there's actually been cases where to help consumers that may have eating disorders, this is an example, an actual example, an eating disorder type of situation where they were getting advice and the chatbot advised them to go on a diet.
Rachel: Oh my god.
Maia: Exactly, so it's like, ah. And so that's when you have these non-fine-tuned generative AI models that are not fine tuned for the specific use case, and you have the human in the loop.
And so in most healthcare settings, human in the loop still needs to be present in order to provide those checks and balances to be able to mitigate risk and, you know, making sure that the patients really are getting good information.
And when deployed in healthcare systems, that currently continues to be the model that, you know, generative AI specifically just isn't good enough for those rare cases where it may give advice that could cause harm to patients.
Similar to the examples where you gave where it may promote increased risk of suicidal ideation, or suicidal acts, or advise dieting for somebody with an eating disorder.
Rachel: Do you see those as the most serious risks or are there other more serious risks that we're not paying attention to?
Maia: Well, I think that less is the one-off. I think more of a, when it comes to size and scale is just overall utility. Who benefits from, specifically, we're talking generative AI model?
If you have a generative AI model that is, say a chatbot or that's providing some level of triage, who's adopting it? Who is for having another way to access healthcare and who is not?
And so there's going to be inherent differences in the level of acceptance of technology. And we've actually learned this from digital health in general, I'll use the example of the patient portal.
Many of us, you know, we have MyChart or some sort of access to patient portal where you can chat with your doctor, you can get prescriptions, you can make appointments.
Well, we know that the adoption of that technology is higher amongst those that are socioeconomically well off, that have higher digital literacy, and hence have easier ways or more avenues for navigating healthcare, healthcare resources.
You can get an appointment at 2:00 in the morning, right? Where others who haven't maybe adopted the technology as readily have to pick up the phone, and still use really archaic ways of accessing care.
So that can be a barrier to access care, widening a disparity. So just from a utility perspective, you know, how do we deploy it in a way that is culturally appropriate, that's even say language-wise?
Rachel: Yeah.
Maia: You know, especially, we know that now, with generative AI, it should be so that no matter what language you're speaking that you're able to access that technology. But has it been deployed language-agnostic, right?
And so can every patient population access the technology? If you need a computer or you need to use the patient portal in order to leverage generative AI, then already we know that it skews higher socioeconomics that has higher digital literacy. So higher value to those that already have a lot of healthcare resource or disproportionate amount of healthcare resource.
So I think that is actually more worrisome when I think about gen AI and is utility from a utility perspective. And then how do we ensure that we provide the support so that everyone is using the technology.
I mean I say this to students all the time when they're exploring generative AI, when you actually ask students, you know, this is early days, you know, who is using ChatGPT, it'd be almost like three to one, males to females.
Rachel: Definitely.
Maia: And it'd be like no women, you've got to play around with the technology because the divide is already, it starts from day one, right?
Like, that comfort and finding that benefit from generative AI starts from the very moment you start exploring the technology. So I think that's more worrisome.
And then making sure that we provide the support for those that haven't and understand why. And sometimes it's because of the way that it's been designed and deployed in a way that may not be as culturally relevant to populations that typically are not the early adopters.
Rachel: I'm reminded of an amazing book by Anne Fadiman called "The Spirit Catches You and you Fall Down." I dunno if you've read it.
Maia: Oh yes, of course, yes, lovely, wonderful.
Rachel: An incredible book about a Hmong family whose child had a seizure disorder. And you know, they were very fortunately in the California Central Valley with access to very high quality medical care.
However, the Hmong family didn't have very much English, and the medical team had very little cultural understanding of Hmong cultural practices. And the resulting series of miscommunications led very tragically to the child's death.
And in reading it, although it's a very compassionate book, I felt very convicted as a technocrat because the medical team, while proceeding from these absolutely laudable aims, were so enmeshed in their technocratic context that they did not have the imagination to understand where the family was coming from and why the miscommunication was happening.
I worry that with gen AI, we've distilled that technocratic point of view into a powerful technology and now we think it's omniscient and infallible, and it isn't.
Maia: Absolutely, and even that, like, again, the way that we deploy it is very much based on the perspective of those deploying, that we often do not have patients, we do not have community that is involved in making sure that it actually is deployed in a way that resonates with that community, with diverse community members, right?
Like, even from that design perspective, it's just very easy to say, "Oh, but we are all patients. I was once a patient." It's like, nah, not quite. It doesn't quite translate.
Yes, we are all patients, but we all don't have the incredibly diverse cultural competency to be able to address every single culture. And hence why we need to have those connections to communities specifically within healthcare when we're deploying technology and that we have the voice of community, that we have the voice of diverse patient perspectives to be able to design a deployment mechanism that really resonates across populations.
Rachel: And I think this is something people learn as their parents age. It's one thing to access health services for yourself. It's quite another thing to access health services for your ailing and and maybe disabled parent.
And that I think is when people start to learn a little humility and imagination. But by then it's often a little late. What can healthcare providers and technologists do to mitigate some of these risks?
Maia: Yeah, so from a risk mitigation, so often, I'll talk about the AI life cycle, right, from problem formulation to generating the dataset to training for a particular model, developing the model, deploying the model, fine tuning the model, deploying the model, right?
Like there's all these steps across the AI life cycle. And when it comes to mitigating risk across the steps is just making sure that you do have good risk management, and that risk management for specifically bias detection and mitigation can be both social mechanisms by having, say diverse teams, diverse perspective.
I talk about community, about different, say even diseases. You have a lot of different disease communities making sure you have like a lot of representatives across that are part of this design process.
But also from a technical perspective. And this is for the technocrats, there are really good methods out there for both detecting and mitigating bias. And so we need to be systematic in our ML ops process at leveraging these methods so that we know, even if it's just stratifying performance across demographic groups, that the models actually work across demographic groups, right?
Just because it works on the aggregate, do you actually truly know who it's working for? And when it comes to a product for sale, it doesn't care if you have a beanie baby, right, like, and it only resonates with two-year-olds. That's fine.
But this is healthcare. It actually has to work for everybody. And you have to have that stratified performance across populations to know that you're at least not harming anyone, but aggregate can often hide that variation at the subpopulation level.
Rachel: Right, and neglect is a sort of invisible harm, isn't it? You don't have to be actively hurting somebody to underserved them and that may lead to preventable bad outcomes.
Maia: Absolutely. So I mean, I'd say you got to first start with measurement.
Rachel: Yep.
Maia: And all of the methods, especially the fairness metrics for AI bias, and these are all of your AI community, ought to know or be versed on AI bias and different methods for both detecting and mitigating it.
That's just standard ML Ops. My son took data science. He's now a freshman in college. Actually, now he's going to be a sophomore in college.
Rachel: Where does the time go?
Maia: I know, time flies. He took data science in his senior year of high school, and they taught ethics of AI. They taught about algorithmic bias. You know, these are things that kiddos are learning in basic high school data science, right?
And there should not be a technologist out there in AI that is not aware of both its existence, as well as methods, and be adopting and using these methods to both measure bias, but also mitigating it.
Rachel: And in case anybody didn't take that in high school, shout out to Dr. Joy Buolamwini and her book "Unmasking AI," which will catch you up on all that.
Maia: Exactly. If you're going to keep up on the gen AI, you got to keep up on the whole body of literature when it comes to methods on biased detection and mitigation.
Rachel: I know my friends in nursing and midwifery are watching these trends with apprehension for the future of their jobs. And being my friends, they're doing the kinds of hands-on community care that, you know, we hold up as the ideal.
What are some strategies for healthcare workers to coexist with AI in the workplaces of the future?
Maia: Yeah, that is a great question. So definitely from a co-development perspective, nurses, midwifery, we all need to be part of the process when it comes to how AI is adopted within your healthcare system, what kind of problems is AI going to be leveraged to address, and whether or not it's appropriate for that particular use case.
And I'll use an example of, there are some technologies out there where they're advertising that they're going to be replacing nurses, right? Like, $9 an hour for a nurse bot, something like that.
But the question really is, are those jobs, are they threatened or are they doing work that would alleviate the burden that a nurse currently has? And believe me, burnout in nurses and midwifery is at an all time high.
And so the overwhelming need is solutions that help address burnout so that nurses, and midwives, and providers of all types can reconnect with the joy of providing care, the connection to patients, and what really matters. And the only way we're going to be able to differentiate where AI is appropriate to use and where that human touch, and human in the loop, and human oversight is necessary is by having those practitioners be it part of the design, the deployment, the monitoring of the AI within their domain.
So get involved. Be involved in AI governance. Be involved. All these healthcare systems have AI governance or are developing AI governance mechanisms. They have technologists that are responsible for deploying it.
Those technologists are supposed to be deploying it in a way that's assistive and not punitive. And so they're looking for that feedback, they're looking for that engagement, and sometimes they just need a little nudge. Say, "I'm going to be there. You're not going to know."
And believe me, the technologist, generally, doesn't have the background to know when it's appropriate or AI's best used to alleviate burden, and administrative burden is overwhelming in healthcare, and when there's an opportunity to reconnect with our primary mission when it comes to providing care and connecting with patients, connecting with community, connecting with the reason why we entered into care delivery in the first place.
Rachel: I mean, it's true for all of us. We don't want the computers to do the parts of our job that are fun and generative. We want them to do the parts of our job that are tedious and soul destroying.
Maia: Absolutely, and the idea is really so that everyone can work at the best of our capabilities, right? We want tools. That's what a tool does. A tool makes us that much better, right?
And Gen AI is no different than any other generic tool in providing that opportunity to be the best version of us when it comes to whatever task it is that we're trying to do. And so that's how I see Gen AI, is it can make us better at what we do.
Rachel: And give us more time to sit in the garden, and read books, and recover from burnout.
Maia: Yeah, exactly. So there's going to be a tipping point though.
Rachel: Yeah.
Maia: And at that tipping point, I think that you, you know, where jobs really are going to be replaced, but there should be adequate time to understand where there's opportunity for upskilling, where there's opportunity for skilling for other tasks and opportunities.
And that's really what we should be doing concurrently, is figuring out, you know, how do we make sure that each individual, if you're responsible for care team, for a team of providers, for a team of employees, that those employees are preparing for this opportunity to be the best of themselves.
And that best of themselves may not be the current way that they envision their role.
Rachel: And you're living this right now. How is the experience of founding Equality AI different from the very senior academic positions that you've held in the past?
Maia: Yeah, so I think that for Equality AI, what's different, I mean, it's all the stuff that I wouldn't have had to manage as say a technologist, right?
So I'm a chief digital technology officer, I have my technology team, we're very focused on digital transformation. We have project managers that we can work with. We have a marketing team that we work with.
We have a sales team that we work with, but we aren't responsible for sales. We aren't responsible for marketing. We're not responsible for, say, project management per se in itself. We're not responsible for legal. We're not responsible for HR.
We have all these wonderful, helpful people to help us. Well, of course, as you know, as a founder, you're now it.
Rachel: You're the janitor.
Maia: And the janitor and the administrative assistant. And so the wonders of gen AI specifically has been in that, of course, all of these amazing and innovative companies that allow for essentially be fractional HR through your software or fractional lead for this other software.
I won't name specific software that we use, but we have all sorts of technologies that we use to be able to enable me as a novice salesperson be perhaps a mediocre salesperson.
Rachel: Well, underselling yourself right there.
Maia: But yes, so, like, that's I think the power of generative AI. Oh my gosh, I use it all of the time, right?
Like, how do I become an expert as fast as possible? And that definitely is leveraging technology to augment me from a novice to perhaps somewhat of an expert.
Rachel: Are there any ways in which being a startup founder is similar to what you've done in the past?
Maia: Oh, yes. So a lot of it is the same, connecting with people. I love the parts of say sales, like, you know, obviously, sales--
Founder led sales is an important step for every startup. And the part that's the same is that human connection. It's actually very similar to patient care. It's very similar to speaking with colleagues. And that once you've realized that 80% of, whether it's sales or presenting to investors, or talking with investors, a lot of those communication skills are universal.
Rachel: Yeah.
Maia: So that part has been pretty seamless.
Rachel: What are some of your favorite sources for learning about AI?
Maia: So how I keep up is I'm still very much steeped in the academic world. So I give keynotes frequently. I'm a frequent invited guest.
I've recently presented at the National Library of Medicine on responsible AI. Tomorrow I'll be giving a presentation to the general accountability office.
Rachel: Wow.
Maia: Yeah. So that'll be a lot of fun. So I'm very connected to academia, and so that helps me. My colleagues help me keep current. And so I write as well. Generally, these are academic publications for like New England Journal of Medicine AI, and I'm usually a co-author.
So I'll write with my colleagues and then, you know, that process enables me to keep current. I go to conferences as either speaker, usually I'm as a speaker, but I stick around around for the rest of the conference 'cause you're going to learn something amazing.
And like, Symposium for AI for Learning Health Systems, that's a conference that I help organize. Harvard's Department of Biomedical Informatics is the one that's a key sponsor. And I've been on that committee for quite some time.
So I get to read, review 50 or hundreds of abstracts. So that early exposure, this is when they pre-publication and just reviewing what people are doing.
And that's actually probably the most cutting edge because it's like, before they've even been published, you're get to read their abstracts, or their publications, or their manuscripts prior to publication.
So that's the ways that I keep up. Wouldn't say that's going to be your general way.
Rachel: Just get a couple of postgraduate degrees, and get affiliated with Harvard.
Maia: Exactly, right?
Rachel: If everything goes exactly how you'd like it to for the next five years, what does our future look like?
Maia:
I think that we have an incredible opportunity to address some of the biggest challenges, societal challenges, whether it's healthcare, education, finance, you name it, that we have an opportunity to harness technology in a way that it's never been harnessed before, but really to help elevate everyone.
So five years from now, what I would see is a world in which we each have what we need to be successful and live to our fullest potential. In healthcare, that would be specifically models that are debiased and that work for everyone.
But I think that it's beyond just healthcare. And that's if we do AI responsibly, and I think there's enough interest, and we all should be mandating that regulations are in place that ensures that there is some safety net, that there is this floor that we all can benefit or find foundation from in order to be our best selves through technology and through our own humanness.
Rachel: That sounds really good to me. Finally, my last question, my favorite question. If you had a generationship voyaging to the stars, what would you name it?
Maia: Well, I have always liked my name.
Rachel: I was just thinking that actually. Hightower would be a fantastic name for this ship.
Maia: Both names are pretty cool. Specifically, Maia is my first name, and it is just like a universal name.
So something that resonates across cultures. No matter where I've been, people have said, "Maia is a name in my country or in my culture." And so something that we can all connect with and, you know, Maia itself is very easy to say.
Hightower has its own power. But words that I think that resonate across cultures are always fascinating because of course a generational ship would be a little microcosm of all of us.
Rachel: Yeah. Dr. Hightower, thank you so much for being on the show. I'm looking forward to embarking on the Maia to the Stars. It's been a joy as always.
Maia: Absolutely. Thank you so much for having me, Rachel. It's been an absolute pleasure. It's been absolutely fun. Thank you.
Content from the Library
Generationship Ep. #24, Nudge with Jacqueline-Amadea Pely and Desiree-Jessica Pely, PhD
In episode 24 of Generationship, Rachel Chalmers speaks with Dr. Desiree-Jessica Pely and Jacqueline-Amadea Pely, co-founders of...
Open Source Ready Ep. #1, Introducing Open Source Ready
In this inaugural episode of Open Source Ready, Brian Douglas and John McBride embark on a technical and philosophical...
Generationship Ep. #22, Back to the Real World with Elijah Ben Izzy
In episode 22 of Generationship, Rachel Chalmers speaks with Elijah Ben Izzy, CTO at Dagworks. Elijah emphasizes the importance...