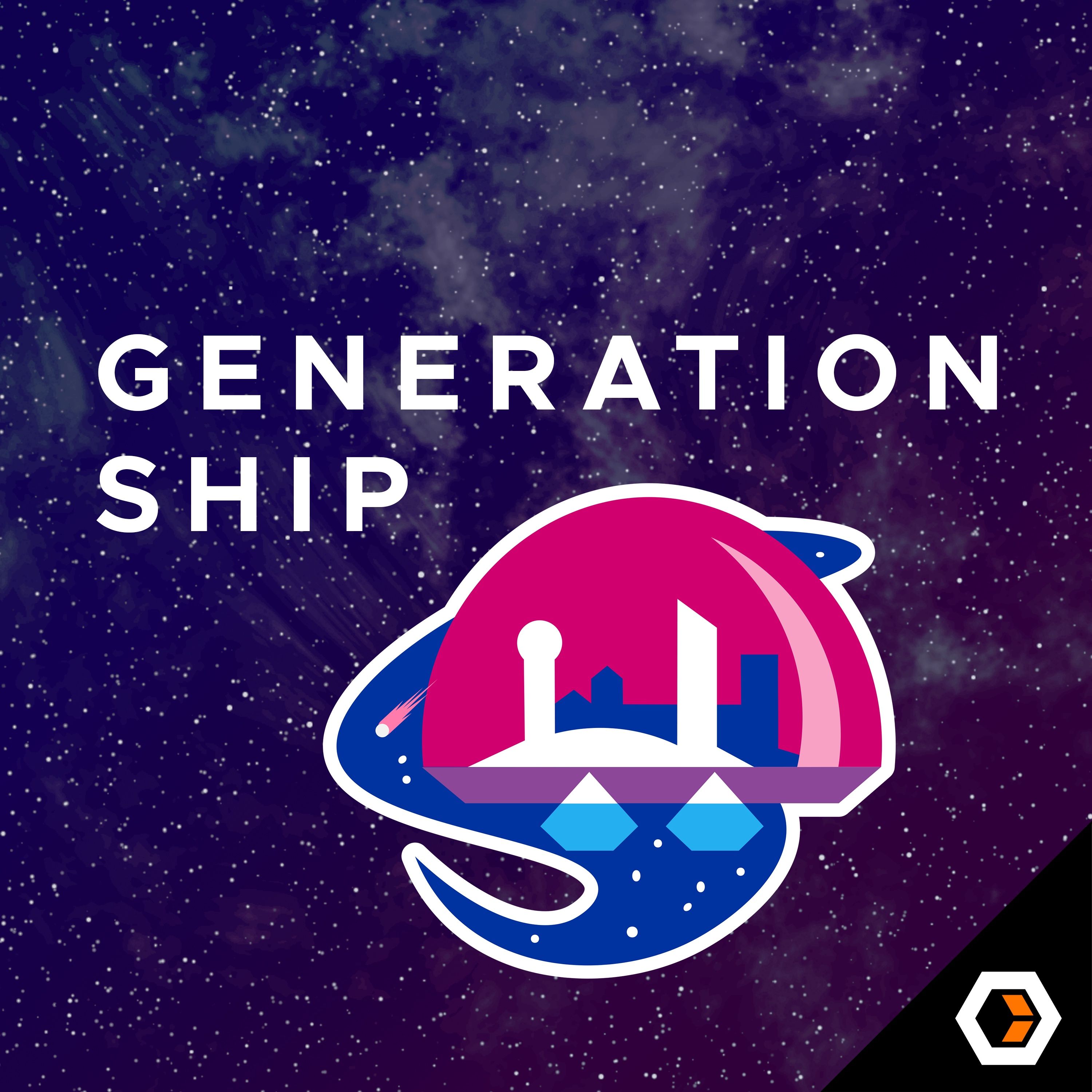
Ep. #28, Collective Intelligence with Emily Mackevicius
In episode 28 of Generationship, Rachel Chalmers speaks with Emily Mackevicius about intelligence in all its forms—from songbird learning to group cognition in subway rats and humans. Emily explains how her research connects the dots between neuroscience, AI, and our ability to collaborate, envisioning a future where technology amplifies collective problem-solving.
Emily Mackevicius is a neuroscientist and co-founder of the Basis Research Institute, where she leads the Collaborative Intelligent Systems Group. With a PhD from MIT and postdoctoral work at Columbia, Emily studies how intelligence emerges in both animals and AI. Her interdisciplinary research explores distributed cognition and its applications to real-world problems, from climate change to collaborative technologies.
In episode 28 of Generationship, Rachel Chalmers speaks with Emily Mackevicius about intelligence in all its forms—from songbird learning to group cognition in subway rats and humans. Emily explains how her research connects the dots between neuroscience, AI, and our ability to collaborate, envisioning a future where technology amplifies collective problem-solving.
transcript
Rachel Chalmers: Today, I'm absolutely thrilled to welcome Emily Mackevicius to the show. Emily is a co-founder and director of Basis Research Institute, where she leads the Collaborative Intelligence Systems group.
She did her postdoctoral work studying memory expert birds in the Aronov Lab and the Center for Theoretical Neuroscience at Columbia. And her PhD work studying how birds learn to sing in the Fee lab at MIT.
She's interested in how intelligent behaviors emerge, especially in distributed and recurrent systems. Her theoretical work is strongly grounded in experimental practice, currently high resolution behavioral recordings of groups of animals foraging in environments ranging from New York City parks and subways to Arctic Alaska.
Emily, it's so exciting to have you on the show. Thank you for your time.
Emily Mackevicius: Thanks so much. I'm really excited for our discussion today.
Rachel: I, like many people in the Bay Area, coped with the pandemic by putting out a bird feeder and getting to know my local avian neighbors. So, I'll bite. How do birds learn to sing?
Emily: Yeah, so I guess first of all, not all birds learn to sing. So songbirds are the birds that learn to sing. And birds like chickens and geese don't actually learn their songs.
And birds learn to sing kind of like people learn to speak. So when they're very young, they will listen to other birds around them, especially their parents. And in some species, only the father will sing. In some species, both will sing.
And then the baby birds start babbling, similar to how people start babbling and they'll practice their songs. The songs will get better over time because they form a memory of their tutor's song and then they try to match their tutor song. And that's basically how the process looks like.
Rachel: It does sound a lot like human speech. My niece sent me a video of her son who's four months old, lying back and babbling, and the babbling is clearly mimicking the rise and fall of conversation. It's fascinating to watch that.
Emily: Yeah, yeah. It's interesting how they'll kind of like pick up on some aspects whether it's the rhythm of the song or whether it's particular notes that they'll repeat and they'll get parts of it. And then by the end, they will turn each of those different parts into a great song at the end.
Rachel: And goodness, you defined as sounding more like their tutor, their father, in many cases. What is the song for? Why do they need to replicate that the sounds that their father is making?
Emily: Yeah, so the song is, in many species, part of how they find a mate. And so birds will sing to attract other birds.
They'll sometimes, if they have territories, they'll sing at the edge of their territory, almost these kind of singing battles so they don't have to actually physically fight each other and they also just sing to practice.
Maybe they enjoy singing possibly as well. It puts them in a good state of mind. But how they use the song is in mating or some kind of territorial type of things.
Rachel: And are there negative evolutionary pressures on birds that can't reproduce a good quality of song?
Emily: That's a good question. I think one thing that is interesting is that like each species is very different, and the song is a way that the species can tell, this is my species and not a different species.
Some species of birds look very similar but have different songs to each other, and it's a way to kind of evolutionarily distinguish themselves.
And I think it's probably also kind of an indicator of overall brain fitness, kind of like the peacock's feather has evolved as kind of like a mating display.
Rachel: Yeah.
Emily: The song would be seen like that as well.
Rachel: What exactly are memory expert birds?
Emily: Yeah, so I guess for some context, I studied song learning in my PhD. And then in my postdoc, I studied what we call memory expert birds in the Chickadee family.
And these birds hide food in many different locations and they're memory experts because they can remember all these different locations where they've hidden their food.
Rachel: That's wild.
Emily: Yeah, so it's kind of like instead of migrating for the winter, they'll stay in cold climates and just manage their food supply by remembering where they've put all these different pieces of food.
Rachel: We have woodpeckers here in California that peck holes in the oak trees, and so you'll see these incredible oaks that are just like peppered with acorns tapped into their trunks.
And I wonder if the woodpeckers go back to their own acorns or if they just like snack on whichever acorns are handy.
Emily: Yeah, yeah. They've done really cool studies with different species of birds. I don't think woodpeckers specifically, so maybe the woodpeckers just go to whoever's acorns, but using radioactive seeds to see...
Rachel: Oh wow.
Emily: Whether birds actually retrieve their own seeds or somebody else's seeds. And then you can tell because they'll have a band in their feathers. So I think these studies were done a long time ago. I'm sorry, I forget who did them. But yes, they do go back to their own, yeah.
Rachel: It's so fascinating talking about animal intelligence. We are an AI podcast obviously. So all of this research has led into your current work. What did thermal images of groups of subway rats teach us about emergent intelligence?
Emily: Yeah.
So I'm really interested in intelligence in the context of multiple people or animals working together. I think that there's been a lot of focus and progress on looking at what does human intelligence mean? What does it mean to be the best chess player or the best go player? Or something like that. But a lot of the really, to me, impressive things we do, we do in groups and that's true with animals as well.
And so I'm interested in looking at how groups of animals together can, for example, survive the cold winter outside. Survive, in the case of the subway rats, the subway is very different from the bulk of their evolutionary history.
It's a very different type of environment and yet a lot of them are thriving, you know.
Rachel: Eating pizza.
Emily: Eating pizza, and all of that. And that's kind of like where people are at as well, is like we're living in ways that are different than a lot of our evolutionary history and we are interacting with other people and figuring it out.
So I'm studying rats and birds as a model of just how cognition works in groups.
Rachel: We've had this conversation before on the show, I think. We came through 50 years of, you know, talking about rats getting addicted to cocaine and talking about alpha wolves being super dominant.
And it has turned out that a lot of those behaviors that we see in animals were specific to captive animals that were under a lot of stress, and you saw this aggression and dominant behaviors.
And now that there's been a move to more like ethological approaches studying animals in the wild, we've uncovered evidence of a lot more commensal behavior and collective behavior.
Is that something that you've seen in your work? Do you think that we've overlooked the potential of collective intelligence for a little while?
Emily: Yeah, I think that in some ways, it's just been hard to study. I think that's kind of where AI comes in for me, at least in my work, is that I think that a lot of the lab-based studies that you're describing, it was kind of like the best you could do with the tools at the time because you couldn't really quantify behavior very well, or at least like in that much detail.
And you go outside, it's totally uncontrolled. You can't keep track of what's happening. I mean, there are obviously amazing like ecological studies, but like at the kind of high resolution that you were getting in the lab, it was really hard or even impossible to do that in kind of real world environments.
And now with computer vision and algorithm done, the audio recordings and everything like that, you can really get kind of the type of high resolution information that you would otherwise need to do in like a very tightly-controlled lab study.
So yeah, I think there's, it's kind of a really exciting time for looking at like real world animal behavior because I think this really motivated a lot of lab studies. But it just was kind of impossible to get that kind of quantitative data before.
Rachel: That's so cool, just being able to process all of this data at unprecedented scale opens the door for so much more research and so much more insight into how animals behave.
What about the flip side? How does what we know about animal intelligence inform the way we build AI systems? What are the strengths and weaknesses of neural nets versus language models, for example?
Emily: Yeah, that's a really good question and there's kind of a really cool long history of this interface between AI and neuroscience in both ways AI helps us understand the brain and then the brain gives us ideas for different AI systems. It's obviously not a coincidence, it's called a neural network, you know?
So I think that in the past, there was just like a lot of crosstalk of literally some of the same people that are interested in understanding intelligence were also interested in building intelligence systems.
And they looked at how the brain had these units, neurons, that are connected to each other in networks and they wanted to create similar systems in computers. And I think that those ideas were around actually for a while before there was enough computational power to get good results with them.
But yeah, I think, still used a lot. I would say there's not a very hard boundary between like neural networks and transformers. I see transformers as kind of another version of a neural network.
And through the whole history of AI, I kind of feel like people are making lots of different modules or parts and then stringing them together in different ways. And that's kind of made them more and more powerful in addition to having more data, you know?
Rachel: And this does seem to mirror the way brains evolve, right? You have a wonderful talk where you're actually looking at neurons firing inside a bird as it's singing and you can synchronize different parts of the brain to different passages in the song.
Are these multimodal AI systems that we're setting up, do they behave like different parts of the brain? Do they light up the way neurons light up?
Emily: Yeah, that's a really good question. I think that what we're starting to see is AI systems that really look like multiple brain areas, getting threaded together.
I think originally, and for example, if you take a deep network, each unit might be analogous to a neuron or a collection of neurons. And together, they might model the visual system or the early visual system.
But what about like deeper brain structures like the basal ganglia, which people think does reinforcement learning or the hippocampus which people think does memory? And so if you string together like a perceptual system, a memory system, a reinforcement learning system, that's how you start to get even more powerful AI system.
So now instead of just stringing together neurons, you're almost like connecting different networks together where the output of one network forms the input of the next network.
Rachel: Yeah, it's interesting to think about reinforcement learning in the context of machines because when you're raising children or animals, reinforcement learning is about pleasure.
Typically, it's about positive reward, positive reinforcement. You need a sort of evaluation layer in computers to provide that reinforcement, don't you?
Emily: Yeah, and that's kind of a really neat area of research because people definitely just kind of come up with their own rewards. You know, you might invent a game that you're playing with people and just really, really want to like get this thing into that box by throwing it and whatever it is.
But it's like, that's not something that you were born with. That's something that you might have just invented. And then that's kind of something that you can use as a reward.
Actually, songbirds do that too. So they compute whether their song sounds good or not, and this depends on what their tutor taught them. It's just like, does it sound like the tutor?
If it sounds worse than expected, then if you record dopaminergic neurons deep in their brain, they'll get, if it's worse, they'll get a little dip in dopamine. And if it's better, they'll get a little spike in dopamine, compared to what they expect.
So that's an example of birds learning a new reward. And people obviously do this a lot. And I think with AI systems, you're starting to see people being creative about what type of rewards that they give AI systems.
And I think that one of the things that is like really creative about what people and animals do is they can come up with their own rewards that are not really tied to food or something like that.
Rachel: Yeah, humans are very strange.
Emily: Yeah, yeah.
Rachel: What led you to co-found Basis Research?
Emily: Yeah, so I was doing my postdoc, studying these memory expert birds. I had been interested in AI for a while, but more as like talking to friends about it and using some of the techniques.
And basically, I just got more and more interested in it and started talking with the people that became my co-founders in their research and connections with my research and figured that it would be really cool to start something new there.
And I think also at the same time, like, I was considering applying to tenure track faculty jobs and I was realizing that there are some things that I really love about academia and that kind of job path and then some things that I wish were a little bit different.
And this was a cool opportunity to kind of create or define for myself collaboratively, like what type of work environment I wanted to work in, so.
Rachel: So you left the lucrative world of academia for the equally lucrative world of nonprofits?
Emily: Yeah. It's like, I knew I wanted to do research. I knew I wanted to be surrounded by interesting ideas and all of that. So I kind of took the parts I knew I really liked, and then, yeah. Mm-hmm.
Rachel: So Basis is a nonprofit lab looking at wicked problems and we face so many wicked problems as a species, as a planet. How optimistic are you that we can harness some of these AI tools to address them?
Emily: I'm pretty optimistic. I think obviously, there are ways that things are not going to be as smooth as we think they're going to be, you know?
But I think that a lot of these tools are becoming more and more accessible and also more and more interpretable in the sense that like you can specify an AI system by its cost function, which is something that you can talk about even if you don't have a really advanced like CS training. It's basically your values. Like, what do you want this system to do?
And so I think that that and kind of the coding that's enabled by large language models, make it the case that a lot more people are empowered to build some AI systems to solve their real world problems that they're seeing. And they can talk about them and design them at the level of like values and cost functions as opposed to at the level of like more of the engineering details, which if you don't have a CS degree, you kind of don't know how that impacts a lot of these things that you actually care about.
Rachel: It does feel like a democratization of computing at a scale that we haven't seen since the early web.
Emily: Yeah, yeah, which is quite cool to see.
Rachel: But on that note of the cost benefit analysis, do you worry about the carbon footprint, the water intake of these data centers that are underpinning the large language models? I know Basis does a lot of work on climate change.
Emily: Yeah, I do worry about that. I think that that's an important line of work to try to reduce the carbon footprint of large models and not just use them like crazy, you know, to try to focus on more like targeted problems. It is something that I do think needs to improve.
Rachel: It's wild. I never thought that anything would like get people to restart Three Mile Island in my lifetime. I'm actually sort of agnostic on nuclear. It's obviously potentially very dangerous, but so are coal and gas. Do you have a perspective on the energy that will be needed to power these systems?
Emily: Oh gosh, yeah. I'm really not an expert in energy, you know, but we should be able to do better in terms of the energy consumption.
I don't think we should take it as a given that these systems need to use as much energy as they are now. I think maybe the first versions are, but our brain is incredibly more energy efficient than any of these models. And so we should, and I think we can make them more energy efficient.
Rachel: That's the utopian promise, isn't it? That if humans could harness collective intelligence, if we could use our big powerful brains in concert, we would be able to address these wicked problems. Is that something you think is feasible?
Emily: I hope it's feasible, you know? I think that we need to think about the ways that we communicate with each other. I guess, back to like evolutionarily, you know, like historically, how many people were we interacting with? Not that many. And now we can interact at a much higher scale.
And I think that we need ways of doing that, that are really kind of harnessing all of our intelligence where a lot of the current ways of interacting with a ton of people might not harness our intelligence as much as it really could and might tap into some, you know, just following the crowd, that kind of thing.
I mean, things like this podcast are great for kind of communicating ideas, different voting systems, different prediction systems. I don't know.
I think it's a cool time to think creatively about like, how can we collaborate this way? And we see that with like open source software for example, which is a really cool way that people collaborate with each other and review each other's code and together are creating something.
It's not like just one lone genius created this, but a lot of people created it together. But you really need to think about like the systems that we communicate with each other.
Rachel: Yeah. What are some of your favorite sources for learning about AI?
Emily: Yeah, I often like hear about things from colleagues. Also, just like following people on Twitter is one way, setting up paper alerts for people that I read a paper of theirs that I really like.
You can get emailed by Google Scholar about when they have a new paper. And yeah, just kind of talking with people, seeing what people are excited about.
Rachel: I really loved your GitHub profile page. It was really rich and I encourage our readers to go and look at that. We'll include the URL in the show notes, but lots of your talks and then lots of stuff that you're interested in and working on the side.
Emily: Oh, thanks.
Rachel: If everything goes exactly how you would like it to go for the next five years, what would the future look like?
Emily: Yeah, so at Basis specifically, we have started a couple just like very ambitious projects that could go in many cool directions.
There are projects that are more basic science about understanding intelligent. There are projects that are more applied about trying to design tools for local policy makers.
Then there's what we call our core tech, which is connecting these with general tools that could be used for a variety of different applications. And I think that we've started them, we've come up with some cool prototypes.
And I think the next five years could look like really making serious progress in each of these directions for Basis. And then I think even broader than Basis, I think that Basis is an example of almost like a slightly new creative way of doing science and we're seeing more and more people doing that.
We talked earlier about this kind of democratization of AI tools and so I think that just seeing more people create things that they wish were in their life with AI tools and actually being able to do that and being able to share that with people would be great, being able to communicate about like what our values are through these, kind of discussions of what the cost functions of these models should be.
Rachel: How can people get involved with the Basis community? How can they play with your core technology?
Emily: Yeah, so we have a GitHub that has... So there's ChiRho, which is part of our core technology that is for causal reasoning and dynamical systems. It's all open source.
For my project, the Collaborative Intelligence Systems project, there's a GitHub that's Collab Creatures, but yeah, I think it's Basis org on GitHub, is where all the different Basis' open source code repositories are there.
Our website, which is just basis.ai, has sections for like if somebody wants to collaborate or wants to apply for a job position or an internship or wants to collaborate.
Rachel: Very cool. Last question, my favorite question. We are Generationship the podcast, we are imagining ourselves on a journey to the stars. If you had a starship, what would you name it?
Emily: I guess I'll start with what would I want on it? I would want kind of a lot of different people, maybe different animals as well, different plants.
Rachel: Birds.
Emily: Different birds. What would I name it? I'm not good at names, but...
Rachel: I don't know, Basis research is a pretty good name.
Emilyl: I think in some ways that we, I think of Basis as this kind of like starship that I'm on. So, yeah.
Rachel: That's a beautiful name for a starship. The basis of everything, our ecosystem, our interdependence on each other.
Emily: Yeah.
Rachel: Emily, thank you so much for taking the time to come on the show. It's been a delight.
Emily: Thank you so much. Yeah, this was really fun.
Content from the Library
Open Source Ready Ep. #12, Exploring Flox and Nix with Ron Efroni & Ross Turk
In episode 12 of Open Source Ready, Brian and John welcome Ron Efroni and Ross Turk from Flox to explore the world of Nix, a...
Platform Builders Ep. #4, Building Affinity: From College Dropout to SaaS Leader with Ray Zhou
In episode 4 of Platform Builders, Christine Spang and Isaac Nassimi interview Ray Zhou, co-founder of Affinity, about his...
Generationship Ep. #34, Together with Nathen Harvey
In episode 34 of Generationship, Nathen Harvey brings data, humor, and heart to a conversation about AI, DevOps, open source, and...