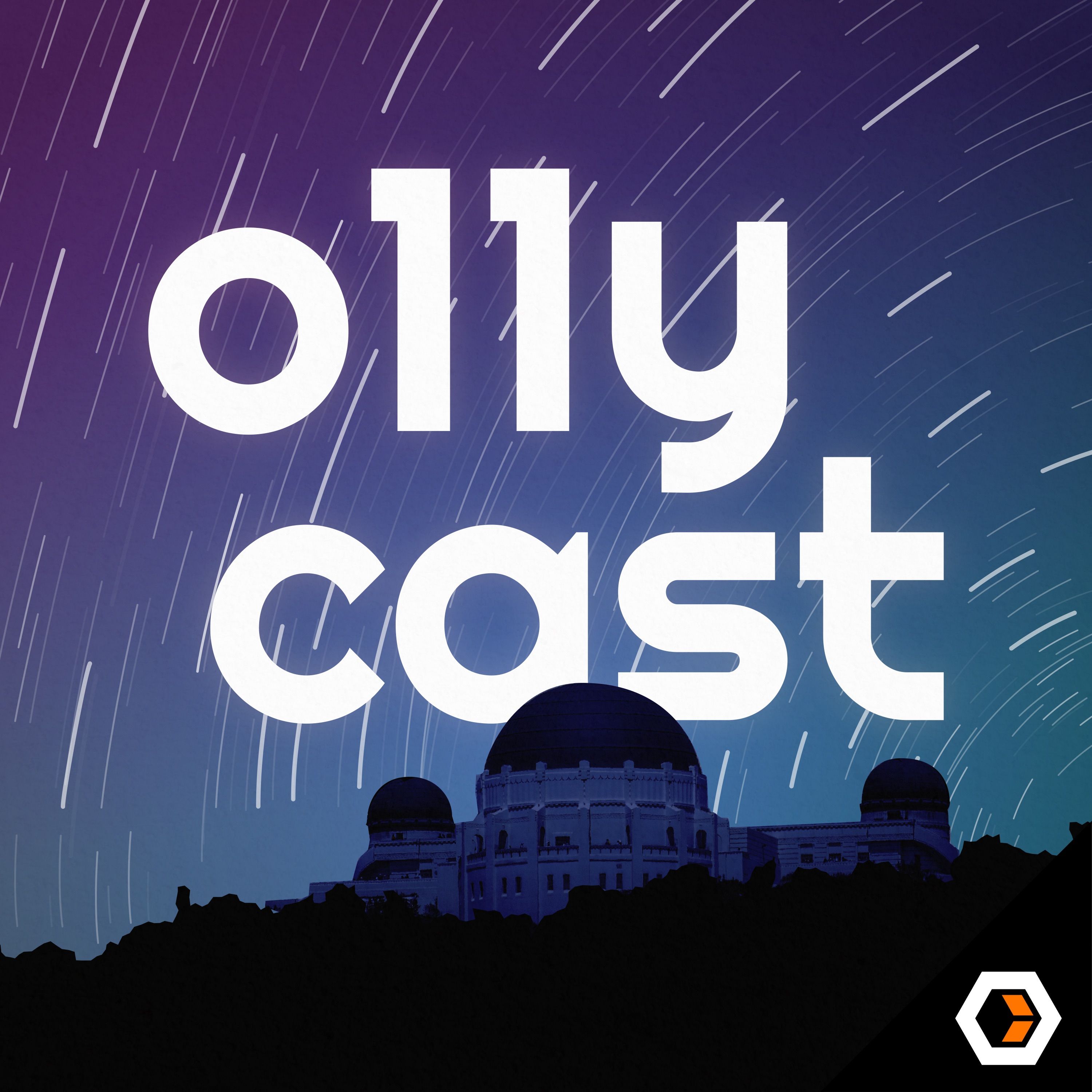
Ep. #61, What Comes After o11y with Heidi Waterhouse of Sym
In episode 61 of o11ycast, Jessica and Martin speaks with Heidi Waterhouse of Sym. Together they explore the sensations of proprioception and interoception, how they relate to the future of observability, and the ways machine learning could assist in simplifying that future.
Heidi Waterhouse is a marketing advisor at Sym, where she works with the team to refine messaging, target the right customers, and consider the future of their product. She was previously the principal developer advocate at LaunchDarkly.
In episode 61 of o11ycast, Jessica and Martin speaks with Heidi Waterhouse of Sym. Together they explore the sensations of proprioception and interoception, how they relate to the future of observability, and the ways machine learning could assist in simplifying that future.
transcript
Heidi Waterhouse: I'm not an observability expert, I'm just a person who talks to a lot of people.
Jessica Kerr: You're just a cat whisker.
Heidi: Just a cat whisker out there, feeling the vibes of SRE and DevOps and observability, and a little bit of security. But I think that what we're getting to is the next step beyond observability, which I'm calling proprioception and interoception.
Jessica: Proprioception?
Heidi: Proprioception.
Jessica: And interoception?
Martin Thwaites: I'm not sure we can pronounce those words in the UK.
Heidi: I bet you can. So what they are is they are bodily senses that tell you where you are in space, and whether or not you need to pee.
Jessica: Okay. Now, proprioception, that's like the where is my hand thing, right?
Heidi: Right. Like can you touch your finger to your nose with your eyes closed? Do you know where you are in space? And do you know if you're upright or sideways?
Martin: Sorry, I needed to try it.
Jessica: Well, we're all trying that. We're all trying that right now.
Martin: I think I can do it.
Jessica: Martin forgets to close his eyes.
Heidi: Right. So proprioception is interesting because a lot of people in technology are also neurodivergent and both of these senses are something that is sometimes affected by neurodivergence.
Jessica: So what is interoception?
Heidi: Interoception is an awareness of your own body's sensations and needs.
Jessica: Ooh. That's the do you have to pee thing?
Heidi: Do you have to pee? Are you hungry? Are you angry? Are you stressed out? Do you feel feverish? These are all interoceptive.
Jessica: Oh, so this is that thing where I'm like, "I'm angry," and then I pause for a minute and I notice that also I haven't eaten in too long?
Heidi: Yes.
Martin: Cause and effect?
Heidi: Sometimes, yeah. But both of these sensations are not what we think of as a sensation. If you ask somebody what the senses are, they're all like, "Seeing and hearing and touching and smelling." But we have a bunch of other senses that we use all the time and we just don't talk about the same way because they're less direct to manipulate.
Jessica: That's true. So your usual five senses are all about noticing the world outside of you, but there's also this whole world inside of you. Does that correspond to the world inside of your software?
Heidi: Right. Yeah. I think that what we're getting at with the next step of observability is going to be something more like, "I feel a little rundown, maybe I should have some vitamin C." Observability has taken a huge step toward being able to be proactive about break-fix, but it is still a lot of break-fix. We're much faster at being able to detect what went wrong and with high cardinality data we can correlate a lot of points that point to multi source failures. But we're not doing the thing where we can say, "hey, something is a little bit off. It is not yet broken, but it is exceeding safety parameters."
Jessica: We're approaching the boundary of safety.
Heidi: We're approaching the boundary, I feel a little rundown, I think I should take a nap. I feel like maybe I should pee before I get in car for a long trip. I want our software to be able to do that, to be able to say, "Look, this system as a whole has this level of health."
Jessica: So this is like with kids, we've gotten as far as observability which takes our systems from babies which just scream no matter what's wrong, to toddlers which can tell us, "I'm cold, I'm hungry, I want more," and now we're going toward the level of taking care of your own needs. Which my teenagers do pretty darn well, they put themselves to bed, they make themselves food as long as we have mac and cheese and ramen handy.
Martin: But you wouldn't leave them to their own devices.
Heidi: Yeah. They become less carbivores as they go on, I'm sure. I live in hope.
But I want observability to take all of this high cardinality data and all of this understanding of how our systems interact with each other and start giving us whole systems feedback. Not just, "My disc is full," but, "Several of my discs are a little fuller than normal. Is there something going on? And does that rise to the level of alerting?"
Jessica: Or, "My disc is approaching its boundaries and my autoscaling is not coming online quickly enough."
Heidi: Right. And I think we're actually pretty close to it if we thought of it that way. When we started doing observability, we were taking things that we already had, we already had logging and monitoring and time series databases. It was assembling them in a way that was useful to be able to interrogate that made it observability.
Jessica: Or creating entirely new databases that make it easy to interrogate either of those things and more?
Heidi: Right. But the very start of it, we could assemble things to give us an approximation and then we iterated on that to make it much better and faster.
Jessica: Once we raised our standards in how much we want our system to be able to tell us.
Heidi: Right. And now that we're collecting all of this high cardinality data, I think this is a, I have to say it, place that machine learning is going to be super handy.
Martin: Ooh, she said it.
Heidi: I did say it, I did say it. I did not say AI, though, because I'm allergic to that whole concept. I think that there's a lot of opportunity for us to say, "In a system that has an architecture a little like yours, this caused a failure. You are approaching that configuration. Do you want to take a look at that before it fails?
Jessica: All right, I want to talk about this some more. But first, Heidi, how about you introduce yourself and tell us how you became a cat whisker?
Heidi: My name is Heidi Waterhouse, and I am a DevRel, a technical write and right now a Go To Market Consultant. What I do a lot is listen to what's going on in the industries that I've chosen and help people figure out where they want to be headed. That's kind of what I've been doing all along, even in technical writing that's what I do. It's, "What is it that you want out of this? What's your goal and how do we get there?"
Martin: I think that is something that is lost in a lot of companies, they don't ask that question early enough. They have some cool idea, but don't ask why, don't ask how do I get there, don't ask what I want from this particular thing I'm going to create. Then they lose their way.
Heidi: Yeah. And so I think it's super helpful to come in and say, "What's your success metric for this company?"Not, "How do you know if you're making money?" A much more complicated question, but, "How do you know you're making the right thing? Who do you want to be helping?"
I think a lot of us get into software because we're frustrated with the way things are and we know it could be better, so how can we make it better?
It's a lot like buying real estate, which I'm doing right now. I'm like, "You call this a process? Where are the checkpoints? Where are the safeties? Where's my rollback?"None of these things in purchasing real estate, it's just like this asymmetrical narrowing of options where they can pull out at any time but I can't. I want to make that process better, but I also don't want to deal with it.
Martin: So I want to get back to the machine learning aspects because it's a hot topic right now, machine learning, generative AI, all of those buzzwordy things that are going on. I think you hit on something around the similar systems, the idea that we want to know what is unique about your system, what's the same about your system and the other systems that we know about.
That's kind of like our own inbuilt knowledge where you hire somebody who's done a SaaS platform before and they know how SaaS platforms work. So they know this idea that, "Well, if it's a SaaS platform, maybe it's to do with tenants, maybe it's to do with noisy neighbors, maybe it's to do with this."
And they have this inbuilt idea of how these kind of systems work, which means they've got this inbuilt context which allows them to be able to go, "If this happens, maybe this is the problem." I think that is potentially where some of this machine learning can come in by identifying some common aspects of your system, and applying it to some other systems. Is that the sort of thing that you're thinking about?
Heidi: Yes. I think that as we build a richer corpus of failures, we're going to start to see more indicators that we didn't know were indicators, that we wouldn't look for as humans but computers don't have the same biases that we do. So we'll find out that it's actually a terrible indicator for six months down the line if your social media platform starts ticking up a lot.
You're like, "Oh, actually it turns out that this is an indicator that people are doing a lot of searches on what else is available." If they're on a platform and they start searching a lot for my platform versus other platforms, they're about to churn and we might not know that. It's sort of a marketing example, but I can see how that would also be true for all sorts of systems and things.
It's like, "Okay, the switch failing is well within the normal parameter of switch failure, but it's increasing in frequency. Not enough to trip something, but we've seen this switch fail this way in other times and places."
Jessica: So computers like people, but differently than people are good at noticing patterns. But just like, "Hey, this pattern reminds me of this other pattern that eventually was followed by some sort of doom," isn't actionable. Can these things ever get at causality, get at explaining why and what we should do about it?
Heidi: I think maybe at some point, but certainly not in the near future with what we have. That doesn't mean it's not going to be valuable, but causality, it's almost always retrospective. You can't say, "If you eat that, you will 100% get a tummy ache," to a kid because 50% of the time they won't and then they'll be like, "Nyah."
Martin: And then all of your credibility is lost for everything else that you tell them to do. I mean, we had no credibility to start with, so that's not really a thing. But I can 100% see where you're going with that.
Heidi: So when we say, "Can we do causality?" I don't think so, not with what we have now and not with what I see on the horizon. What we can do is pattern matching and alerting. It's like, "Hey, did you know that just like the Kaiju Gates in Pacific Rim, the frequency of this is increasing? And it's not so close together that you will notice, but it is in fact getting closer and closer." I would like to be able to do that kind of monitoring and say this is a little unhealthy. It's not broken, you don't need an emergency response. It's just not healthy.
Martin: I think this is all just information data points, isn't it? It's the giving people more things to go on, it might be that maybe things aren't healthy or things are healthy but maybe you want to do something. But you're not going to get somebody out of bed. I think it's kind of like that idea of SLOs, around the idea that it might go wrong in the next year, it might go wrong in the next week, it might go wrong in the next day. Providing people with more information, providing people with an idea that maybe they shouldn't go to bed early tonight because it might go offline, it might be a good thing. I don't know.
Jessica: So one reason that at Honeycomb we're generally against things like AI Ops is that our systems are constantly changing and what was normal in the last month might not be what's normal this month because we just brought a new service online or we got more customers or whatever it is. The point of observability is to support us changing the system, and therefore the system is changing. They're talking about even noticing stuff across systems, which were never identical. If we were going to do that, would we need a lot more standards, standard signals, standard, 'This is what this means'?
Heidi: I think it would be great if we had them. I think every time we create more standards, it makes it easier to interoperate and transfer the things that we want from where they are to where we want them to be. But I also think that when we say system, we're talking about a bunch of different things. Jess is like, "Oh yeah, let's talk about the-"
Jessica: So many!
Heidi: But I think that, although it would be nice if we could tag and meter everything we do, that's not a realistic goal. What's a realistic goal is figuring out some way to take the system's temperature to say, "Overall, are you running about the same or are you running a little hot? Are you running a little cold?
Jessica: Okay. If we looked at, say, Kubernetes, because Kubernetes has the wonderful property of putting a common vocabulary around things and putting abstractions on top of workloads and volumes and whatnot. You could say, "How's this node running?" You can say, "How is this deployment feeling?"
Heidi: You could say how many times have I had to repopulate these nodes? You could say how many times has this workload hung? You could say is this image more or less likely to fail than another image?
Jessica: Yeah. Like which ones are the problem children?
Heidi: Right. That's sometimes hard for us to detect. I think that's one of the things that ML is good at, is they're very large numbers and we have squishy, little Jell-O brains, sometimes we can't detect those patterns and machines can.
Jessica: Okay. Then what do you do about it?
Heidi: Well, then what you do, if you find your problem child, you still don't know causality. You don't know if it's a problem child because it was misconfigured or because the workload is somehow idiosyncratic and is just interacting poorly with something. But it at least directs your attention, it at least says stop looking at the good kids and go look at this one. Something is here.
Jessica: Just like we can't tell you why your customers are suddenly searching for you versus competitors, and we can't tell you what to do about it, but we can direct your attention.
Heidi: Right. And human attention is the thing that makes computers seem smart. They're just sand that does a lot of math. Yeah, I am insulting my computer while I'm online. I live dangerously. What makes computers seem smart to us is that they allow us to figure out what we want to look at, what we want to pay attention to. There's no reason for us to need to understand machine language, because that has been successfully abstracted. So what we're talking about is another abstraction layer on top of your system or systems that allows you to look at the thing that needs attention. Preferably before it breaks.
Martin: Yeah, I think what I'm struggling with is the precognitive ability of these things, that idea of this will go wrong in the future. We live in the observability space around asking questions of our systems, the causality thing, you can only do the causality from the past. What's interesting is can you use that sort of information to say, "If this thing went wrong, well, let's use that learned experience from all of these other systems to say this thing went wrong, in similar systems we saw it go wrong because of these things." Now, the only way we get that is by having that corpus of knowledge of people going, "This thing went wrong. This was the cause."
Jessica: Oh, okay. So you get like, "Hey, here's this signal that we recognize in yours, and here's the stories that were constructed post-hoc by other people who had that problem."
Heidi: Right. I also think of it like airline safety. So planes have an error budget, and no matter what exceeds the error budget, we recognize that exceeding the error budget means we need to pull the plane out of service. Sometimes this looks like we can't take off because the latch bin is broken, and you're like, "Are you kidding? Just duct tape that thing up. I need to be in a place." But what that symptom is saying is that this airplane has not been sufficiently maintained and it needs to be pulled offline and fully scrubbed for safety issues. And so the symptom points us to something that may or may not be severe, but because air travel is so safety conscious we care enough to err on the side of safety.
Jessica: Which, in general, software is not. I mean, your business software typically, every nine is really, really expensive and we don't want to... Well, we certainly don't want to take things offline preemptively but we don't want to spend all of our time investigating similarities to somebody else's problem.
Heidi: Right, especially if it's not actually going to cause a problem in our system because we have guardrails around that or we have some other way to mediate that. That said--
Observability has not solved problems, observability doesn't solve problems. It just helps us figure out where they are. But it's addressed a lot of problems and yet I would want to go further, I want to be able to say, "Okay, something is hinky with this image. Do I want to just recreate it and see if I can do better? Do I want to understand what's going wrong? Is this something that's worth my time? How business critical is this?"
Jessica: Yeah. Because that problem child in among your Kubernetes deployments might be like, "Yeah, okay. You're not going to get optimal recommendations. There's a good fallback for that."
Martin: I mean, the idea of maybe it's the same base image. Everybody who's using this particular base image for their containers, everybody always has this problem. Therefore, like you say, it's not worth spending the time. You might as well just switch your base image for your containers. But again, we need that massive corpus of knowledge in order to make those judgments.
Jessica: Then the more knowledge you have, the more patterns you see and the more competition for your attention.
Heidi: Right. The other problem is the standards problem. If we feed into this corpus who can back trace it to us, can we anonymize it sufficiently? Are we feeding more into the commons than we're receiving from it? And if we're just really good at this and don't need the help, why should we contribute to the commons so that our competitors can do better?
Jessica: So now there's some sort of open source analogous problem to the corpus of system health data.
Martin: I mean, is that similar to the CVE database?
Jessica: Oh, CVE, the Common Vulnerability Ease?
Heidi: Yeah, but the security thing that says, "Hey, look. This is a problem. It's probably a problem for everyone who uses this software. You might want to get on that." But every time a company has to do a CVE on their own stuff, they're revealing their own vulnerabilities. Literally.
Martin: Yeah, so the idea of saying, "My system went down and here's the root cause," we at Honeycomb pride ourselves on being transparent about outages and downtime. That's something that we really pride ourselves on, but there are other companies out there that, for various reasons, some legitimate and some maybe not, that will keep those investigations as to why, a secret. And, yeah, without those large scale companies being really open about why things fail, you miss out on a massive chunk of information.
Heidi: Right. So it's possible that the machine learning could be just internally trained if you have a sufficiently large system. I think that there are lots of solutions that are only solutions for people doing a lot of scale, and this is unlikely to be a solution for somebody who isn't working in the huge range. So we used to talk about this metaphor where servers were cattle, not pets, and it was this mind blowing transition where we stopped naming individual servers after Simpsons characters.
Martin: We went with Norse gods because you can also then name the underlying VM platforms after the tiers of existence that exist. It was a whole thing.
Jessica: It used to be fun. You've taken this from us.
Martin: It was the privilege of those lead developers to name something. It's like, "I'm going to create something because I get to name the server it lives on."
Heidi: Right. And now we're not dealing with that. What we're dealing with is not cattle, but amoeba colonies, like bacterial colonies where you do not care about any individual. All you care about is the health of that Petri dish. All you care about is the health of the system as a whole because it doesn't make any difference how many you pop up or push down, as long as the colony is healthy. I think that at that scale, it makes a lot of sense to be doing this kind of awareness where you're not monitoring a server or a disc, or even a fleet.
Jessica: Because the experienced scientist in the lab can look at that Petri dish and look at its color and look at the distribution of the bacteria across the dish and say, "Healthy, not healthy."
Heidi: Right. Why is my soup navy blue? Well, we're going to figure that out.
Jessica: Or get new soup.
Heidi: Well, you should get new soup. But there was a whole Twitter microbiology investigation in what kind of bacteria turned soup blue, and they finally figured it out but it only happened at refrigerator temperatures.
Jessica: Okay. So again it would take a lot of experience with a lot of soup to recognize that pattern. And in the meantime, you'd recognize a lot of patterns that were false like it only happens in leftover soup, and it only happens in... I don't know, yellow soup which might just be coincidence.
Heidi: Right. Only soup with beans has this problem.
Jessica: It only happens on weekends, but really it's just that people put their soup in the fridge on weekends.
Martin: I don't know whether it's true, but that idea of the server that goes off at 5 PM on a Friday every week and then comes back on 20 minutes later and nobody knows why.
Jessica: And it was the soda machine that was plugged into the same outlet.
Martin: Well, the example I heard was that it was the cleaner that came in and unplugged the server to plug the vacuum cleaner in, did the vacuuming and then plugged it back in and everything came back online. We know it goes off but we don't know why, but it turns out it was actually something really easy and really simple.
Heidi: My wife had a tech support job where there was this constant monitor degradation, and it wasn't until she walked around and looked and found out that the imaging, the X-ray machine was on the other side of the wall from the monitor and it wasn't properly shielded from that.
Jessica: And these are things that we're never going to find out from our observability data because the factor that is causing the outage, the commonality there is outside of the boundaries of our system as we've defined it, and not feeding data into the same place.
Heidi: Outside the boundaries of our observed system. But I think that when we define a system, we inherently as humans also have fuzzy edges about it. So my sister talks about nines, she works in Google networking and she's like, "I don't know why we're trying for five nines because the electrical grid is not five nines. Maybe we should address that first." And she's not wrong, I'm not sure she's allowed to say that in public, so oops.
Jessica: Your hypothetical sister.
Heidi: Hypothetical sister. But as humans we have this titration of interest out from what we think of. It's like I have defined this system, but of course it matters that I have electricity and nobody is using it to do the vacuuming. Why would I need to say that? It's obvious to me. So does our defined system include power generation? Does our defined system include back hoes? No, not usually.
Jessica: But can we recognize that assumption that we have, and put in some information on a nice metric on do we have power? Do we have power? Do we have power? As that is a totally necessary input.
Martin: I think if you ask the question, do you have power, and you don't get a response, that's a negative because... Do you have power? No. How are you sending me the metric?
Jessica: It could be just that Google networking went down. That's less likely.
Heidi: It's like asking are you asleep? Well, I was. Now I'm not.
Martin: Schrodinger's Power.
Jessica: Some questions are rude. But that is a point, asking are you asleep, that affects what you're trying to measure and health checks affect the load on your system.
Heidi: Right. Every time I am trying to figure out why my computer is so slow, what's the first thing I do? Open System Monitor, is this useful?
Jessica: Yes, and what's using most of the CPU? System Monitor.
Martin: It's normally my virtual machines on my machine, actually. But there you go.
Heidi: But I think that it'll be very interesting to see if we evolve into thinking about system health instead of system breakage.
Jessica: Yeah, degrees of health instead of degrees of broken?
Heidi: Yeah. So is 80% okay? Can we accept that? How many nines does that represent? Is that three nines? Okay, now I know. How do we make systems healthier? What is chicken soup for the system? What is the vitamin C? What is the exercise regime that makes it healthier?
Jessica: One thing I've noticed personally is that if as I'm eating I taste my food and I can try to eat a new food. For a long time I didn't like celery, and then once as an adult I'm like, "Okay, I'm going to eat this celery and I'm going to eat the whole stick," and I did and my mouth was like, "Ugh, what are these awful stringy things?" But my body was like, "Oh, I don't know what you just ate but it was good. Eat it again!"
And now I love celery, and I've even come to like the stringy things because I associate it with this improvement in health. So that's part of interoception too, is that now if I'm feeling really tired and I've tried the usual things like had more electrolytes and coffee, if I go to the produce department and look around, something will look really tasty. It's the food that has the vitamins that I need right now. So, that?
Heidi: Yeah, that. I want that for our systems. I want our systems to be able to have cravings before they get scurvy. I want them to be like, "I could really go for some oranges right now."
Jessica: Yeah, or in the database, "I could really go for less of this one query, and more RAID replicas."
Heidi: Right. "This is yucky, I'm tired of eating it. I don't want it."
Jessica: "I've had spaghetti three nights this week, only one more."
Martin: I like the idea that this particular database query, this is lentils. Stop it now.
Jessica: Yeah. That's kind of reasonable really.
Heidi: It is, and if we ask the right questions we can see what the heaviest query is, but that may not be the one that's bothering it the most.
Jessica: It might be the one that holds the lock for longer.
Martin: It could be one that happens at the same time, accessing the same data.
Heidi: It's like, "I'm tired of answering that question."
Jessica: Right. Or, "I've gotten the same query five times in two seconds. Really, people?"
Martin: "Have you heard of cache?"
Jessica: As a dev, I'm really skeptical about machine learning figuring this stuff out for me. I want my software to know what's unhealthy for it, and maybe it's something I've noticed by querying our observability. Sometimes I get the same query five times in three seconds. Maybe I want to start, one, caching it and then, two, notice whether I hit the same cache record a lot of times and be like, "Hey, why are you using the network? Cache this yourself."
Martin: I think this is about the growing up of systems. These are things that you can't have on a newly birthed system. To your point around babies, toddlers, teenagers, adults, these are individual systems that grow up. It's not about, "I'm a distributed systems engineer, I've worked on these sort of scale systems. I can do all of this with my eyes closed."
These systems need to grow up, they need to have instrumentation that's added, they need to understand themselves. We might be able to say that there's a similar system like this, so there's babies in this particular area. They all act the same, they all look the same, all babies look the same. They all kind of do the same things and then they diverge as they get older. But in systems like this we need to start teaching them, like you said, how to answer the questions that we want them to answer.
Jessica: Yeah. You could put in a baby signs module into your Rails app, add a dependency Rails as a common baby app format, and it would do things like notice your own plus one queries. And tell you about that, so there are common things per web framework and database client that now we settle for, "You can see in your trace that you have an N+1 query."
But really, why can't the ORM? An ORM is an excellent place for some things are healthy and some things are not, and it doesn't tell you. It just suffers in silence and let's your app be slow and your database be a much larger instance than you really could've paid for if you designed it differently. So an ORM that complains with taste, an ORM with tastes.
Heidi: Right. It not only has tastes but those tastes are configurable to fit the system and constraints that you have.
Martin: But it also needs to be constantly evolving as well, doesn't it? Because the example of 2020, what is a normal system? Well, in somewhere at the start of 2020, everybody's system started acted a little bit differently. They got more load if you were an online system. If you were an office based system, then all of a sudden you got less load. Normal changes constantly because of those, like we said, those external factors.
The things that we don't take into account as something that affects our systems. We didn't think that there would be these things, we didn't factor them in. So that normal has to change over time, the idea of saying, "Yes, we'll put it in the ORM. The ORM can tell us these things." Well, that thing needs to understand what normal is and then has to recontinually work out that normal has changed, normal has changed. So what is normal at that point?
Heidi: Right, it needs to be contextually aware.
Jessica: Can we as humans give it clues about that?
Heidi: Sure. So maybe you don't like lentils, but maybe if you're a vegetarian they're a lot better and you're like, "Oh, lentils. Those things with protein. I like those."
So I think that we have to be telling our system how we want it to optimize and how we want it to behave. I think we just don't need to micromanage it because we have abstractions for a lot of that and we should be continuing to develop abstractions for what we want out of our systems.
Jessica: So we should continue to develop abstractions for what we want out of our systems at this meta level, not just give me the data, but give me the data efficiently and tell me when you need help with that.
Heidi: Right, and we have all sorts of things that do that. All of the driving assistance that we have now, we have now told cars that we suck at parallel parking and we wish they would do it.
Jessica: That is an excellent use of automatic driving, excellent.
Heidi: It is. But lane keep assist is the worst feature ever in Minnesota because when it snows, the tire tracks are dark and the snow in between is white and it thinks that I am veering out of the lane all the time and it jerks the wheel.
Jessica: Oh no, that's terrible.
Heidi: It is, it's terrible. It's a slightly older one, I hope that it continues to progress. But in that context, a snowy road, this learning is not useful to me and is in fact destructive.
Jessica: Which is why we always need a human at the wheel of our software. It's a matter of that, in fact, a little lane jerk, the lane assist, steering wheel jerky thing is tapping into our senses directly and our sense of where are we in the world and it's giving information. But the human has to stay in charge.
Heidi: So--
I think that abstractions need to continue to evolve so that we can delegate things that are not important for us to do and pay more attention to the things that are important for us to do.
Jessica: And then double check.
Heidi: And then double check, because parallel parking is a very low stakes thing. It happens at very low speed, and the very worst thing that happens, pretty much, is you dent somebody's car.
Jessica: Yeah. It's a relatively closed system.
Heidi: Right. It's much more simple than I am driving down a street in my neighborhood and there are dogs and cars and kids with balls and people driving their riding mower. Is that a vehicle? Is this a vehicle? That's very complicated. But for the closed system, yeah, I can delegate that and then I have more ability to think about the things that require value judgment. Until we all agree on values, which is unlikely-
Jessica: That's not a thing, that's not a thing. It's important that some of us have different values than others.
Heidi: Right. But even in software and technology, some people have valid reasons for prioritizing very fast compute and some people have very large compute. It's really hard to have very large, really fast compute.
Jessica: Yeah. Well, and when you're first making your Rails app and you have two customers, you really don't care about your query performance. You need to add features and get customers and it totally doesn't matter until it does. It would be nice if your app can say, "This is about to matter."
Heidi: Yes. I have a talk that I did years and years ago called The Seven Righteous Fights, and it's the seven things that people don't think about early enough in product creation. I can never list them all, this is why I had slides. But it's things like localization, API security, user management, the very basics of what you are going to run into first when you outgrow rails or whatever.
Jessica: And the stuff that really handicaps you later.
Heidi: Right. Because if you're doing localization, you can either hard code it in English or use strings that are just English. Then when you go back later to localize, you have these hooks for your next language. Or you have to redo everything that is hard coded in English.
Jessica: Right. So a little bit of acknowledging this is a piece of text, put it over here, that little bit of abstraction leaves you a hook, like you said.
Heidi: Right. And I think that when we're thinking about these baby systems, we don't need to put everything in them at once. Just like rails doesn't scale beautifully, but it does what it does really beautifully, which is be a starter app. It gives us the capacity to climb the ladder without having to know everything all at once.
Jessica: Yeah. We can at least get far enough up to know whether this was the right ladder to climb.
Heidi: Right. And then it's going to be painful to transition, but if it was the right ladder to climb, you have the money to do that.
Martin: That's the thing at small scales, everything runs fast. That idea of just a small Rails, it's going to be fast enough, it's going to have enough of the small features you want.
Jessica: And it would be neat if enough of those companies pooled their beginner data, and the Rails app and modules and things that go into Rails apps, had the instrumentation to output signals into a how are we doing in a way that could be collectively compiled into a knowledge base that would tell future beginner Rails apps, "This is fine now, but when we see this, we know it's about to be a problem."
Heidi: Right. Like, "You have now exceeded 2 million queries. You really want to think about your next step here."
Martin: And looking at the idea that these sorts of systems, you're probably going to have a problem soon because you don't want to hire somebody to help scale your system when you need to scale your system.
Heidi: Yeah, it's too late.
Martin: You need to hire them before because nobody starts the next day. Well, not in the UK anyway.
Heidi: Yeah. And I think that you are reaching the end of the sliding walkway, right? Please gather your bags and prepare to step off to the next one.
Jessica: Perfect, perfect. Okay.
Heidi: Try not to sit near the end of the slide walk, because you hear that over and over again. But when you're on the slide walk it's a super useful notification, like, "Yo, pay attention. You've been on this thing for a quarter mile and you've completely zoned out and you're going to fall on your face at the change in velocity."
Jessica: Great, great. Thank you. Okay, that is a beautiful place to end our discussion. Before we go, Heidi, is there anything else you want to say?
Heidi: I just want to say that I'm not sure this is the future, but I think the future tastes a little bit like this.
Jessica: Mmm, yummy, vitamins.
Martin: Lentils, eww.
Jessica: And how can people get in touch with you if they want to learn more?
Heidi: You can find me at H.Waterhouse@gmail.com, or at my website, HeidiWaterhouse.com.
Content from the Library
O11ycast Ep. #69, Collecting Cybercrime Data with Charles Herring of WitFoo
In episode 69 of o11ycast, Jess and Martin speak with Charles Herring of WitFoo. Together they dive deep into the world of...
O11ycast Ep. #68, Observa-What? with Michele Mancioppi of Dash0
In episode 68 of o11ycast, Jess and Martin speak with Michele Mancioppi of Dash0. This talk examines what it takes to make...
Building Apps with LLM Observability
In this talk, Honeycomb Principal Product Manager Phillip Carter discusses what LLM observability means, how Honeycomb approached...