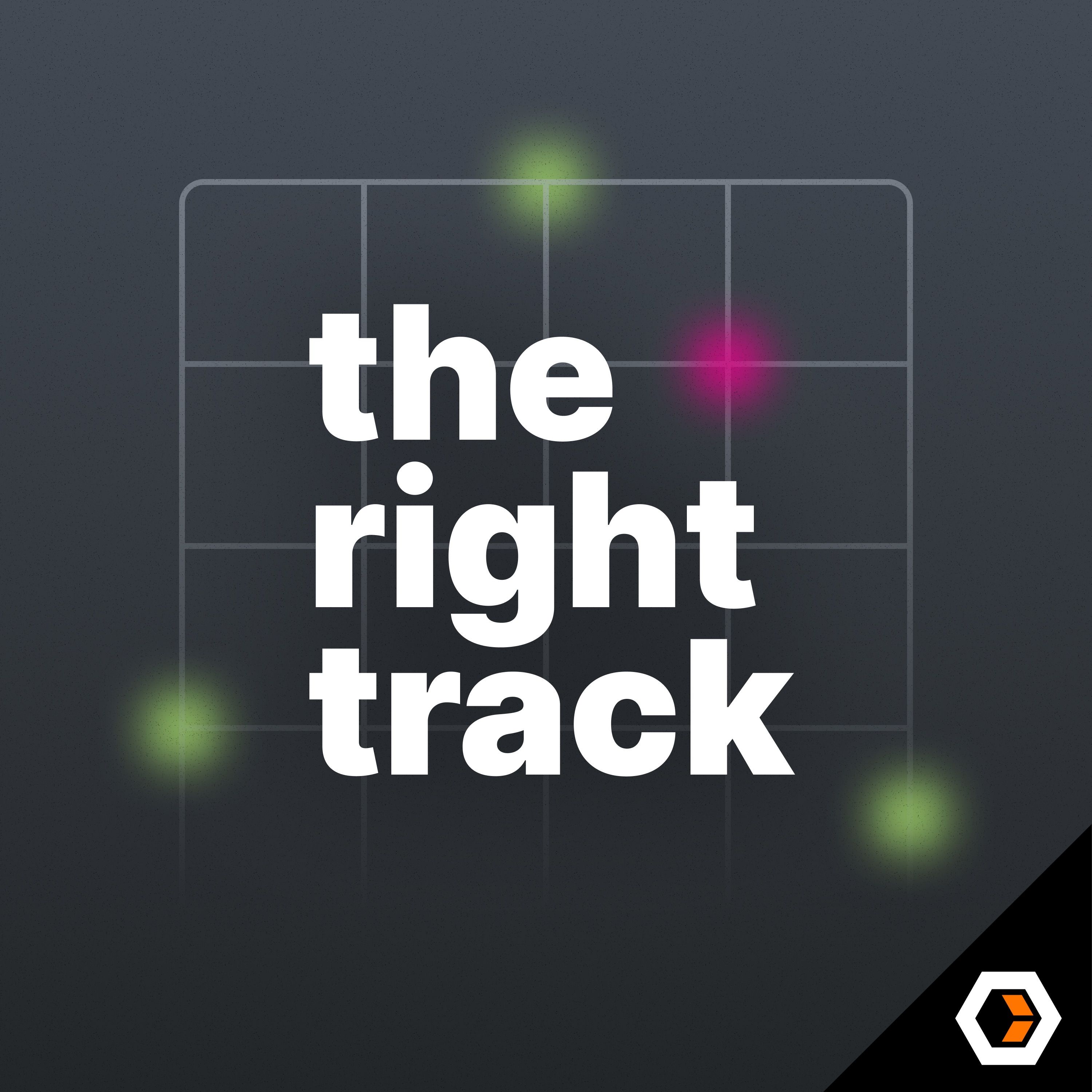
Ep. #4, Data Stewardship with Claire Armstrong of Fender
In episode 4 of The Right Track, Stef speaks with Claire Armstrong of Fender. They unpack the digital products Claire’s overseen at Fender, how her career has interwoven her passions for both music and tech, and tactics for approaching data with user empathy in mind.
Claire Armstrong is the Director of Digital Product at Fender. Claire launched and maintained Fender Tune (iOS, Android), Fender Tone (iOS, Android, web) and Fender Play (iOS, Android).
In episode 4 of The Right Track, Stef speaks with Claire Armstrong of Fender. They unpack the digital products Claire’s overseen at Fender, how her career has interwoven her passions for both music and tech, and tactics for approaching data with user empathy in mind.
transcript
Stef Olafsdottir: Hello, Claire Armstrong, and welcome to The Right Track.
Claire Armstrong: Thank you for having me.
Stef: Such a pleasure having you here.
To kick things off, Claire, could you tell us a little bit about who you are, what you do, and how you got there?
Claire: Yeah, of course. So my name is Claire Armstrong.
I am a Director of Product Management at Fender, focused in our digital department.
My background has actually been in UX and UI design.
I've also dabbled in front end development.
I started off making websites for bands.
My degree is in graphic design.
And I actually started with Fender back at the beginning of Fender Digital.
So I've been here for about five and a half years and I've touched pretty much every digital product at Fender.
So I've worked on our e-commerce platform a bit our marketing side at Fender.com.
I helped launch Fender Tune, which is our mobile tuner app.
Now we have chords, scales, metronome in there, so it's like kind of a cool tool kit for our guitarists.
I also launched a Fender Tone, which is a mobile and desktop app that connects to our digital modeling apps, for controlling presets and effects and that sort of thing, that was a really cool project to work on.
I also helped launch Fender Play which is our flagship digital product, which is, guitar bass and ukulele learning app.
And we have almost 2 million users in Fender play today, very exciting.
And I also worked on a short-lived product called Fender Songs which was based on our riff station algorithm which would listen to recorded audio and tell you what the chords are.
So we hope to leverage that technology in the future. That was super cool.
And now I am the product owner on our data squad.
So I'm doing a lot around data stewardship best practices doing some tracking audits and really focused on improving our data quality at Fender.
Stef: Nice. This is a really impressive backstory, Claire.
I can't believe all of the products you've launched at Fender. That's really impressive.
Claire: Thank you.
Stef: I assume it's been a pretty fun learning journey going from also building websites for bands.
That's a fun backstory as well.
Claire: Yes.
Stef: And now helping the guitarists of the world do amazing work.
Claire: Yes, it's like the perfect blend of technology and music, which, you know you love what you do every day when you're, you know teaching people how to play music and also working in technology and solving some interesting problems.
Stef: That's amazing. I remember one of the first thing we bonded over was Iceland Airwaves.
Claire: Definitely.
Yeah if anybody hasn't been, Iceland Airwaves is amazing because you're seeing these like up and coming Icelandic bands and everybody is so humble but it's amazing music and you're just, hopping around to different bars and venues and concert halls, and seeing such a wide variety of music.
It's really exciting.
Stef: Yeah I love it too.
It was a huge part of my sort of teenage years to always go to this.
And now if anyone is considering it, you will get a, two for one by also seeing a, an interrupting volcano.
Yes which is happening in my backyard which is also pretty fun.
Claire: Yeah I've had some friends stuck in Iceland before because a volcano was erupting.
It was like, oh, shoot your vacation is now extended due to volcano.
Stef: Oh shoot. Thank you so much for sharing this.
This is a really exciting journey that you've been on and it's been a pleasure to hear about it but also just to work with the Fender team on a bunch of these really inspiring products that you're building.
So thank you for sharing.
Would you mind kicking us off maybe, by telling us an inspiring data story?
Claire: Yeah so just to give you more background on Fender.
So obviously we're a very old company, we're 75 years old.
All of our products have traditionally been guitars physical items made of wood and metal.
And you know, the musical instruments industry is a pretty traditional and analog industry.
So back in 2015, when our CEO, Andy Mooney joined Fender, he kind of recognized an opportunity.
We weren't talking to our customers very much. We didn't know a whole lot about them. So we did some surveys and found out more and we realized that a lot of our audience were actually beginners and 90% of our first time players were giving up within the first few months to a year.
And also a lot of our consumers were actually female.
We weren't seeing them in Guitar Center and guitar shops because it's, maybe a more intimidating environment but you know, we wanted to better serve that audience.
So it's been exciting for me coming in as a female musician and guitar player to help encourage other women to also pick up the guitar and to stick with it.
So in 2015 Fender Digital was started with our Head of Digital Chief Product Officer, Ethan Kaplan.
So, you know, we saw this opportunity to better support guitar players at Fender.
So where we've traditionally been making products out of wood and metal.
Now we're making something a little more intangible out of code, but what we're doing is still supporting the player through every aspect of their journey.
We, you know wanted to support how people learn differently now.
So we started off with Fender Tune, Fender Tone, and then launched Fender Play.
And when we first started that all up, we set up Segment and we just started blasting all kinds of events.
So let's put events on everything on every button, on every screen and we'll sort it out later, right?
So we're evolving, we're growing along with our data, we're trying to establish a little more consistency.
So we have Fender, we have digital that brings us up to COVID hitting.
So at the time, you know, the United States was going into lockdown last March.
I was actually five months pregnant at the time.
So I was about to go onto parental leave and Fender recognized.
We had kind of a captive audience. People were going through a stressful time.
They were stuck at home and they were hopefully willing to learn a new life skill, so.
Stef: I am one of those people.
Claire: Oh yeah?
Stef: I spent COVID as most people or so many people.
Been dabbling in guitar throughout my life and then now I have a goal.
I had a goal, I have a goal for 2021 to master all of the scales and guitar.
Claire: That's so cool.
You're, you're kind of fitting into, you know what we were seeing, with our data is we were seeing people that really wanted to start learning.
I'm going to check in with you and see how you're doing in a few months.
Stef: Yeah, totally.
Claire: So Fender saw folks just like you wanting to learn, and we wanted to give back and do kind of a Goodwill gesture.
So we set things up so that folks could start using Fender Play our learning platform for three months free no credit cards, nothing.
Just get in there start watching some videos and learning how to play guitar or bass, or ukulele.
And this was super exciting.
I was kind of watching from afar cause I was on my leave but I noticed, you know, there was an incredible response to it.
We had a hundred thousand people sign up in the first couple of days and we had a ton of new users in the app crazy event volumes as well.
And we better closed the gender gap, which was pretty huge.
We were seeing still a lot of male players in Fender play before COVID hit, but now we're seeing more female players in there and our sales took off.
So we started kind of learning from that experience.
We started kind of optimizing our LTV and looking at the financials.
Stef: For those listening LTV stands for?
Claire: Lifetime value.
So many companies, you know just toss out all these crazy acronyms and you have to learn, you know, what's CAC, what's ARPU, what's LTV, but--.
Stef: You'll all get there one day.
Claire: Yes. So, you know, we really wanted to dig more into LTV.
This was kind of like a scrappy ad hoc process we had to do.
You know, we were using Tableau our product analytics weren't really there yet.
And we were having a lot more users in our product and we realized we were getting higher LTV because there were more people on Fender Play.
So we realized, you know, this is a great signal. We need to do more of this.
So recently we opened up that paywall more.
So now users can hop in and take a test, drive of Fender Play with no credit card information at all.
And we're able to kind of guide them through that journey a lot better than we were before because we learned from that, that campaign at the beginning of COVID.
Stef: That is so inspiring.
So you're saying that, you know you tried out this experiment during COVID to allow people to go further into their learning journey and then you're able to detect using your sort of product analytics or understanding the user experience through that journey, that, that really kept them going for longer, basically.
Claire: Yes. And not only retaining them within the digital product itself, but also they're more willing to shop in our e-commerce site.
And it also positively affects our retail experience as well.
So it was kind of a win-win win.
So now we've really optimized that experience even more kind of opening up that funnel to more people.
So I think that was a great learning experience data-driven and it made us realize even more, you know, how much we need to understand and nurture our analytics so that we can do more experiences like this future.
Stef: That's amazing. And it's such a good example of an inspiring data story.
I think it always starts with some successful learning that you can take and apply to the business.
And, I mean this sounds like a huge one.
Claire: Yes.
Stef: But typically even if you could start with a smaller one, I think that really drives company-wide and team team-wide engagement and excitement about using data to make decisions like these or to discover opportunities as well.
Claire: Yeah, I think, you know, with a company as big as Fender you know, kind of starting off with that big business metric of LTV got a lot of people excited and interested in data.
And so because of that now we can spend our time digging further into things like our user journey through curriculum.
So now that we know how, you know, we can engage people right at the start, now we can help keep people engaged as they're learning.
So where are they hitting snags?
Is it, you know, the E minor to A minor transition that people are getting hung up on?
How can we, you know, get them to that point where they become a guitar player for life?
Cause that's what we really want is we want to keep people learning and excited to learn and motivated to learn.
So now we're really focused on, you know, optimizing that learning journey and it's our data that's getting us there.
Stef: That's amazing. Thank you so much for sharing all of that. Very inspiring.
Thank you, Claire. I'm sure you've also experienced some issues with data.
I feel like, you know, typically there is a lot of inspiration, but there's also a lot of frustration that drives to sort of change, you know how do you experience frustration with analytics?
Claire: So as I kind of alluded to earlier, you know when we first started our event tracking it was just slap events on everything.
We weren't really, you know, asking the big questions early.
It was kind of like let's track it all and sort it out later.
And so I think we've kind of suffered from that over tracking a little bit.
There's a lot of noise. And then there's a lot of ill defined events.
Like we don't know who put this in or when or why.
And so there's kind of a lot of cleanup and auditing we're doing to kind of fix that even down to little operational issues like properties misnamed.
So when I'm looking at a chart and we're calling things lesson, lesson title, lesson name, I'm having to do a lot of extra work to combine that and clean that up is super frustrating.
So we're kind of taking a step back and trying to fix that earlier in the stream so that we're doing fewer workarounds downstream to make things less broken and easier for people at Fender to engage with.
Stef: Exactly and I think this, you know, "small example" that you mentioned that lesson, the lesson title, there's lesson name--
That's such a great example because there's a seemingly small thing that can really increase the time to value from your data, particularly if you're not an expert.
So can you talk a little bit about what that workflow would look like for you once you were experiencing that?
Like what was the trigger for you discovering it and then what process would you have to go through to fix that?
Claire: Right.
Stef: Or to find information in the data?
Claire: Yeah so I think it usually begins with somebody having a question, like, you know what's our most popular song lesson?
So, okay I'm going to hop into amplitude, help you create a chart for that and looking through the data.
Oh, you know, there seems to be a lot of no values. Let's break it down by platform.
Oh, web is passing this as lesson title but iOS is passing it as lesson name and Android's passing it as just lesson.
Now our charts kind of screwed up and how are we going to fix that, you know?
And in the past, maybe it's like we stick a little band-aid on it. Like let's just fix it in amplitude govern or something but I'm really trying to get us, you know now that our product line is mature now that we have a longer runway for our development, let's start fixing things up at the top of the stream and keeping things cleaner downstream.
But it always starts with a question that needs to be answered right?
And once we started actually playing around with the data and trying to answer it, then you realize the issues around it.
And something that I've kind of been doing as we go along is just in the event description maybe, or the property description.
I'll kind of note these caveats, you know this is only happening on web right now.
We need to fix this, things like that.
And then in Jira or my own notes or whatever making sure like, okay, this is a high priority that we need to get this fixed because, people can't accurately report on what's our most popular song lesson because we're passing incorrect properties.
Stef: Yeah and you mentioned so there are two things that come to mind now, this sounds like you're dipping your toes a little bit into talking about how does data and data literacy impact people's willingness to sort of dig into data.
But before I go into that, you mentioned sort of you would prefer to fix and confirm, and govern the analytics a little bit more upstream.
Claire: Yes.
Stef: What is the main driver there?
Like why isn't that enough to patch it with something?
Claire: Because we use so many tools downstream, you know we have our CRM team using something.
We have our analytics team using something.
We have our product team using something else everybody's using these different tools that suit their needs.
And so by patching something downstream, then, you know you haven't fixed it for everybody else.
And, you know, I think it's something that every product development team struggles with is, we don't have enough time let's be reactionary, let's just like do a temporary bandaid and we'll get to it later.
The problem with that is that then no one else benefits from it.
You're only fixing it for one team.
So I do think it's really important to take that step back fix things, you know, on the client or the server side, up at the very top of the stream so that everybody can benefit from, the better described events, from the correctly firing events.
And also you don't know when you're going to be switching tools out.
You could invest a lot of time into a little patch for amplitude or for Iterable or whatever you're using.
And then a year or two later you swap that for something else.
And now all your fixes are broken again.
Stef: Exactly yeah. I think this is very spot on.
I remember Maura Church at Patreon has talked about a similar thing with me, maybe not here on The Right Track but we've had this discussion before where the reason why she wouldn't want to make patches in any of the downstream tools would not only be what you've just mentioned, where while that only fixes it for one team and not all of the others, but also when you, for example, cause in many cases you're looking at some of the seltzer analytics tools but you also want some broad data analysis.
And if you patch it in one of those tools that can be very misleading for any of the other data sources where you'll assume that this is the case, like this is the structure and then you go and deep dive into doing the like digging into the raw data or something.
And all of a sudden you have a completely misleading report basically.
Claire: Yes, definitely.
Stef: Yeah thank you for highlighting that.
This was super helpful and I'm assuming that a lot of our audience can relate to these frustrations.
What is a moment when you realized you needed to change something around this and around your analytics stack and your processes?
Claire: Yeah, so a few years ago, our digital product management team started being involved in, you know more of our roadmap planning, similar to how we do it for our physical products.
So this was really kind of vetting our product decisions goal setting, identifying opportunities and risks with our new features, or new products that we were launching.
And part of that was we to be really be on top of our data.
So the executive team would ask us for a metric for a number and we needed to have a response rather than just, yeah I'll look into that I'll get back to you.
We couldn't wait on our analytics team, you know a couple of weeks to get that response.
So we really wanted to set up something self-service, so that we could start testing out our theories and driving our product development process.
So we implemented amplitude in 2018 so that our product decisions could be less based on instincts or ideas and more data-driven.
So we rely on a lot of qualitative data with surveys and interviews, but we needed that easy access quantitative data to start testing out our theories.
You know, I also realized we had a lot of operational issues, so people wanted to play around with the data, test their theories, but they weren't sure what the events mean, meaning, you know when exactly is this firing, is it, you know, when we get the server response when the user clicks the button I'm not totally sure, it was kind of undocumented.
There was, you know, no single mega spreadsheet there were multiple spreadsheets, for different feature launches.
And there was really no owner of our data tracking.
You know, we would bug some of our marketing folks and some of our data engineers to be like, okay we're doing this new tracking, is it okay?
But there was no one really like owning that process and fixing it at the tracking plan level.
And we also realized we had too many tools.
So there was too much overlap between what our different tools did and people didn't know where to go for different types of data visualization.
So, you know, by setting up amplitude and cutting out some tools we no longer needed we kind of simplified the process of, where do I go to get my data questions answered.
Stef: Yeah, that sounds really great.
So there was a turning point back in 2018 and then you've been on that self serve analytics journey since then.
A really interesting journey that I often spot is this transition from the centralized analytics team where basically decisions are bottle necked by human throughput, the analyst through put.
And then you migrate over to that social analytics structure.
And it sounds like there was a turning point there for you as well, where decision making was bottle necked by a lack of sort of data quality and data literacy as well.
So that sounds like another turning point for you. Would you say that is correct?
Claire: Yes, definitely.
Stef: That sort of brings me on their trajectory which I love to talk about. It's the statement I don't trust this data.
There are various versions of that statement, going around, can you talk a little bit about that why happens and how you see those symptoms over at Fender and how you're addressing that?
Claire: So, you know, we've heard people on our teams say, you know, it's broken I don't trust it kind of more of a negative spin but what I think that's indicative of is that it's not necessarily just about it being broken.
It's about the understanding, of why things are the way they are.
And, you know, you mentioned data literacy and that's kind of what it boils down to is our teams understanding how the data is coming through, you know certain caveats around how it's tracked or stored.
And so I think, you know, my role joining our data squad as a product owner is really around that kind of coaching and education with our team and kind of instilling that that data literacy of, you know, you can't just say all of the data is broken.
You can say, oh, I'm trying to answer this question. And something is not quite right, or we're missing a part or something like that. But it's really about starting at the beginning and getting people to understand why our data is set up the way it is.
And to also understand when the data is directional.
So, you know, I could use it for some basic, you know quick product decisions like, oh yeah more people are engaged with this feature than aren't.
You know, basic things like that.
And then if you do need to dig down and have really accurate data especially when it comes to financials or something else then we can find a different way to do that.
But yeah, I think, you know as people working in the field of data, I like the idea of shifting away from that negative language of no it's not broken, it's not wrong.
We just need to understand it better as a group and kind of arrive at those solutions together, so that everybody kind of understands why it is the way it is.
Cause some things will stay broken or the way they are because they have to sometimes.
Stef: Yeah exactly. So I think this is such a strong theme.
I mean, obviously often data is broken and you'll see things like the lesson and the lesson title and the lesson Stef.
But I think boiling down to this educational piece and the coaching piece is super important.
Not only so that people trust themselves with the data but also so that they are well versed enough in the data to be able to spot the actual tracking issues when they are there.
Claire: Yes.
Stef: So what are you doing in your role to increase the education on coaching?
Claire: Yeah, so I've been doing these data stewardship workshops at Fender.
Stef: Nice.
Claire: So yeah, so I didn't see anything out there like this.
So I kind of developed my own deck and started bringing in some people I thought would be interested and we kind of walked through our high-level data stack and our stream.
So we help people understand what is a tracking plan.
Why do we want to have a tracking plan? What is segment?
What is a stream of events? What's a track event?
What's a page event? What's an identify on call?
What are user properties?
And we really talk about what those best practices are around tracking.
I can either get super into the weeds on it or just keep it very high level depending on who the audience is.
And then talking about that kind of goal setting and looking at those metrics.
And that's actually something I borrowed from Avo from your tool is that idea of looking at the metrics at the very beginning.
So, you know, are you defining a funnel or segmentation?
And then I'll kind of put up some different UI examples depending on the audience.
So is it a marketing site? Is it, you know, sign in UI, that sort of thing.
And then we kind of talk through what would my goals be around this screen?
Do I want to see how many people are viewing the video?
Am I going to be looking at it just in Google analytics?
Am I going to be looking at it in Tableau?
What are some of the questions I want to answer from it?
So we kind of do a data design exercise and I feel like this is just a great way to level set with people and kind of get them thinking about data and why it's important.
Even if they aren't the ones deciding what the event tracking should be on their projects, at least understanding why it's important and why we want to ask the big questions early.
And I've actually, I call myself a AAA girl because I love Avo Amplitude and Atlassian.
So we actually, I set up a confluence template for like tracking plan definition.
So just like the very high level stuff the goal setting stuff, looking at different parts of the UI and creating those events so that people that might be intimidated from going directly into Avo to plug stuff in can just, you know kind of set their high-level goals with data tracking.
So I think doing things like this, I've already had people reach out to me.
Like, I only understood our data like 20% before and now I understand it like 80%.
Stef: Yay.
Claire: So I'm like, cool some metrics awesome.
Stef: Great.
Claire: But just, you know, kind of instilling some confidence with people, empowering them a little bit.
Like you are all data stewards now you get, a special badge to wear.
But really it's about, you know when you're spinning up these new projects you're asking those big questions early.
You're helping us to do a better job with event tracking.
Whether you're not super involved in that process or you're really involved in that process.
Stef: I love the story, this is so fantastic.
Thank you for sharing this with us, Claire.
And I've noticed great discussions in this direction also on the right track community slack lately, where people have been sharing, you know similar to you're encouraging the stewards to think about it top down to think about it from the metric perspective.
And it looks like people are drawing up some mural boards.
That seems to be a common theme that I'm also seeing.
People are drawing up mural boards with like user flows and how you would define a metric that explains a user flow.
So that would be super fun to do a session where we sort of review all of those different ways to educate people on or help people learn a little bit more about what it means to define a metric.
Claire: Yeah and it's interesting, you said user flows because you know, with my background in UX design I loved doing flows and wire frames and stuff.
And with our, when we launched, you know, the kind of test drive version of Fender Play, we actually documented the entire onboarding flow, but we indicated what events were happening when and that was really essential for getting everybody on the same page.
Our paid media, CRM analytics anybody that would be reporting on the data or triggering workflows based on the data.
Showing them the visual of, okay, this event fires when they move from this screen to this screen in the background sometimes it won't fire correctly.
If it's this kind of use case and like documenting out that visual as kind of a user flow, but with our data tracking, I think we're going to be doing a lot more of that in the future.
It was like a very handy reference to have.
Stef: That is amazing. Thank you so much for sharing this.
And it's fantastic to hear that this has increased data literacy already.
And you mentioned that there were workshops what's your cadence for doing them, just for anyone listening who wants to take inspiration from you?
Claire: Yeah, so I've probably been doing them once every couple of weeks.
It's kind of on an as needed basis.
So first I started with our product managers because you know, I sit with them, we're all buddies.
They've been the ones, you know designing the event tracking in the past, where I've been kind of supporting them.
And I just wanted to empower them more of like, okay passing the baton, you all now do this and I'm going to be in a support role for you doing it. And then I did a follow-up with our marketing team.
So really focused with our analysts, with our CRM with our paid media, with producers that are, you know helping to facilitate these kind of standalone marketing micro-sites.
And then, you know, we have all these other teams doing we have e-commerce for specialty brands like Jackson, Gretsch, Charvel, things like that.
We have Fender Academy, which is our training for our retailers. We have Fender Play foundation.
So we have a lot of these digital properties that aren't, you know directly Fender Digital Apps, but everybody, you know can benefit from this information.
So we're trying to loop in people as we hear, you know they're asking, I want to know how many people are in this status or how many views these videos are getting.
It's like, okay, we're doing data stewardship training.
We're going to get everybody on the same page and a better understanding of it.
And then we'll move forward from there.
Whether it's we're going to help you develop a tracking plan or you're going to do the tracking plan and we're going to help you, or, you know what you're doing just hop into Avo and get it entered.
Stef: Amazing so it sounds like you are actually a volunteer taking the step from moving away from the centralized analytics team into the self-serve analytics.
And then you've moved into sort of centralized data governance to support the social analytics.
And you're starting to move into self-serve analytics governance a little bit as well.
That's fantastic. And it all started with better data literacy.
I really relate to this. This is very similar to the journey that we took at QuizUp.
Where I just saw a huge increase in interest in using data, as we involved people more in the data design piece.
Talking about the goals of the feature and how we would potentially measure the success of it.
Because it makes the, for example the analytics tracking "task", it just takes it away from being a "task" that you have to do.
That's not related to the end user and takes it into being, oh this is actually the fundamental reason why we are able to improve the user experience.
Claire: Exactly. Yeah I think, you know, in the past when we would create requirements documents, and we'd be you know, setting up those KPIs or those metrics you weren't as attached to it, you're like, oh yeah we want to improve this and this and this.
Well, when you feel a little more ownership about how you're tracking it because you're going to be the one consuming it because you're going to be the one improving on that feature.
People are a little more dedicated to doing it correctly at the beginning because they know that they're going to be the ones consuming it.
So I think if you're starting some kind of data stewardship education with your team I think starting from the level of who's consuming it, who's having the questions.
And even if they're not the ones directly doing the data design they're the ones that are going to be invested in having that be an improved process in the future.
Stef: Amazing. Thank you for that tactical advice. I really appreciate it, Claire.
Onto sort of a little bit, because this is relevant.
You've talked about who are you training and who are you inviting to these workshops?
Can you talk a little bit about the Fender org structure a little bit from the data perspective, you know.
What do the product teams look like? How to stay to work with product and engineering?
Are the data folks integrated into the product teams or a separate team?
Can you talk a little bit more about that?
Claire: Yeah.
So we've, we've shifted our structure quite a lot especially since COVID and pandemic times to kind of stay agile and, you know, focus on the right stuff.
So we have product squads that are a bit cross-functional.
So we have, you know, our client engineers, product manager, QA, design, platform engineering, all on kind of the squads.
And currently we have our data squad sitting separately.
So our data squad is currently me as a product owner and then all of our data engineers.
And how we kind of operate is that we are hired out.
And we also, we see ourselves as cross-functional as well.
So when we do our roadmap planning we're peppering in marketing and analytics items, we're peppering in engineering items in order to improve our systems and make them more efficient and store and curate our data. And also from a product perspective I have my own needs around data governance.
So we're making sure all of that is represented but we currently sit separate and how I would like to see things go in the future is to have data engineers sit on our cross-functional product teams so that we could have, you know, somebody well-versed in our subscription data or somebody well-versed in royalties or curriculum, that sort of thing.
So that it's less context switching and more, you know ownership around these areas of data.
Stef: Right exactly. I think that's a classic evolution that I think I see often.
It's really important to start with a centralized data squad that can sort of optimize the entire processes.
And then you start by like, you have data folks for hire and then eventually maybe they specialize a little bit but it sounds like currently when you are integrating with the product teams there's no specific person that always integrates with the curriculum team or something like that.
You just sort of take on a bunch of different supporting roles or educational roles in that.
Claire: Yeah and as product owner, when it comes to event tracking with other teams and data governments, that usually falls on me as kind of the representative that visits with that team, which is, you know, my tenure at Fender Digital is really key to that, that I can just, you know hop in and help a team develop some, some new tracking or fix some tracking or that sort of thing.
So that, that way our data engineers can really focus on things needed for our analytics team, for example.
Stef: Right some more intense querying or structuring of the data and things like that.
This is really interesting though that you bring up that your tenure at Fender is one of the things that enables you to support the product managers well, and it touches on something that I've also heard on a few occasions, one of the most important things about building up data culture and sort of supporting the product teams and I don't know adopting stronger data cultures and more productive data cultures is much about relationship building.
What would you say about that?
Claire: Yeah, I mean, I think the roles of product managers has evolved and changed a lot.
You know, I came from a UX design background that would also implement my designs.
And then I kind of moved to a product manager that would do some of the definition and wire frames to more of the business aspect of product managers.
But I think, you know what it comes down to for product folks is definitely that relationship building.
And it's also about kind of carrying this knowledge with you.
So, you know, when people have the complaints about data being broken, the product people, the kind of subject matter experts know the history of why that is the way it is and know what the priority of getting that thing fixed is.
And they also know the right people to ask.
So, you know, somebody has a complaint about data. I know, okay that seems like a client issue.
I'm going to talk to client engineering and file a ticket for it.
Or that seems like a server, you know platform response going awry or, you know knowing the correct people to ask and talk to in order to get the data cleaned up.
So it is definitely a relationship, aspect.
Stef: Yeah I love that. You've mentioned a bunch of tools.
I don't want to maybe spend too much time on it but I'm sure the people who are listening and want to get inspired by you would like to hear quickly a run down of what your stack is for data management, for analytics, all that stuff.
Claire: Yeah so we just started off with Avo for our tracking plan management.
So very, very excited to get going on that.
And we've also been heavy users of segment.
We have protocols in place there we're really looking forward to doing, you know, validation within Avo and Segment, and then Segment pipe stuff out to a ton of different destinations.
Amplitude with govern for that self-service aspect for our product team.
We also put a lot into S3 into kind of a data lake and we use some Redshift curation there and then our analysts use Tableau.
And we also have like a business intelligence team that pulls from Tableau heavily.
We have Google analytics in place, which is much more around kind of web and referrals.
It's more heavily used by our marketing team campaigns that sort of thing.
Also on that side, we have Adjust for our mobile attribution.
We have Iterable, which is used by our CRM team and we've been doing a lot of triggered workflows around our product events.
So that's another consumer of our data that we're is very invested in us making sure that data is well understood and firing correctly.
Stef: And that's piped through Segment into Iterable.
Claire: Yes yeah. So pretty much everything is through Segment.
That was kind of important for me from the start, because at previous companies, it was very much like put this SDK in and put this SDK in and then there was app bloat and we'd be switching things out so much.
So I do like us being able to enable and disable different destinations.
And so then it's more of a matter of keeping the data going into segment as clean as possible.
So we can flip these on and off as needed.
And then we also use Intercom a bit for kind of more qualitative feedback especially around our lesson content.
So users can give us feedback on what's working for them or not in our lessons.
And we use a tool called Prod site to kind of collect a lot of that qualitative information.
Oh yeah and we also use App figures, which pulls directly from Google play store and the Apple app store.
So that's like one of the few things that operates outside of segment so that we can get, you know, app downloads and sales and app reviews and rankings and that sort of thing.
Stef: Yeah exactly and the conversion from installing an app to actually doing anything with it.
Claire: Yes.
Stef: That's the one thing that I learned was surprisingly it was sort of a hidden thing that it was a hidden thing that, you know if you don't get people to actually open the app after you download it, you won't know anything.
And that's super interesting to see that that drop of can actually be pretty steep.
Claire: Yes. Yeah and so I think having that kind of cobbled together with App figures kind of representing the app stores.
Adjust kind of representing that attribution and like the install action and then Segment actually firing up when they launch the app like helps us close that entire funnel.
Stef: Very impressive. I love it thank you for sharing.
Can you talk a little bit about that because you've talked about all of these different stakeholders that care about the data being correct.
You have really important engagement triggers via Iterable and you have the park tours and Entercom you have the directional data in your social analytics tools.
So just so many reasons to have the data work.
And we've talked about the data stewardship workshops sounds like you're making, you know, changes to who is involved in analytics for feature releases.
Can you talk about that?
Who is involved in analytics for feature releases planning it, implementing it, verifying it, analyzing it prioritizing based on data, all those adventures.
Claire: Yes and adventures they are.
So, yeah, starting off with product managers folks like me, you know, we're conceptualizing a new feature and we're really instilling that the data design needs to happen while we're developing the feature.
So we're recognizing what those screens are.
We're recognizing what the steps are what the calls to action are what are those events we're going to want to track right at the beginning?
So in the past, this has been a spreadsheet, Jira tickets and hopefully we're moving more of our product managers into using for that Avo for kind of data design.
And we will be doing more of the goal setting and the tracking plan specification upfront there.
So product managers also prioritize those events.
And really right now people on the feature development teams are not doing much to clean up legacy events.
So that's where I kind of come in. So this is more around new feature development.
And we're starting to involve data engineering a lot more during that design process making sure the data types are correct that we're restricting the types of data we expect to see so that when, when things are validated downstream we can start seeing some of that exception handling start seeing where things are going awry earlier in the process.
Part of some issues we've had in the past is product managers, you know maybe not formatting the events correctly.
So that's what we're getting a lot better with using Avo and also making sure we're putting global properties on every event.
You know, don't forget some essentials there. And then, you know in the past product managers have filed tickets.
So in Jira, and this could be, you know 20 tickets with ticket for each event it could be one ticket with all of the events in it.
It could be a ticket for each part of the new feature with a little thing at the bottom saying like, oh and can you just put these events on it?
So we really need to work on that workflow and process a little more.
And then things get implemented by our client engineers and our QA team.
QA is directly in Segment using the debugger which has been very helpful in the past.
It would kind of be the product managers going in there and kind of doing a UAT thing to like click around and make the events happen and see if they're correct.
Luckily now our QA team helps with a lot of that.
And then it's up to the product managers to set up the visualizations, the dashboards in amplitude.
So they know the events they know the events that are coming in hopefully as correctly as possible.
And then they start setting up their charts to prove the success of the feature as it launches.
And then they're posting about it in Slack and letting people know how well their new feature is doing.
Stef: That's amazing. That's an amazing workflow.
It sounds like you're doing a lot of great work to and already done so many things to make it so much better.
So congrats on making progress. I would say.
Claire: Thank you.
Stef: Moving over to sort of more of like a philosophical discussion.
What do you think people's biggest misconceptions about how data and product analytics work?
Claire: Yeah so the word data is very vague and all encompassing.
Data is made up of many things.
And, you know, as a company, people say like, you know when they hear the word data, they think, okay, yeah data squad will work on it, but not knowing that there are analytics, there are metrics, there's qualitative data, quantitative data.
There's a lot of aspects of data that you can't just like toss the data label on any old thing.
There's a lot of different aspects to it.
And especially at Fender, you know our more traditional side of the business has been a BI team or data analytics team really focused on kind of more of the financials.
So sales resulting from the apps.
That LTV I mentioned lifetime value, but maybe folks aren't as well versed in what behavioral data is.
So like actual product analytics, how are users clicking around and actually using our apps.
And, you know, as I mentioned with the data stewardship trainings, people didn't understand what our data stack or stream was.
How were things set up? A lot of people didn't know that so much of our data comes through Segment.
So yeah, I think a lot of our misconceptions has been around just like, lumping everything into the data bucket but not understanding, you know, BI versus analysts versus scientists versus there's all these different roles.
There's all these different types of data understanding the data as like a stack. People understanding about event tracking, where it happens that it's, you know happening in the clients, it's happening on the server where it goes, how it can be used the whole client side versus server side thing.
A lot of folks still don't understand.
So I think when you talk about misconceptions around data and product analytics, I think, you know, the whole data stewardship and people understanding that the data is not broken.
It's kind of up to all of us when we're setting up these data cultures at our companies to help guide these misconceptions, help educate.
And it is a changing world, you know, tech changes you know, every year or two, it's like a totally different ball game.
You know, I was out on parental leave for a little bit and I came back and stuff was already way different, so.
Stef: I love it. Yeah, exactly.
Yeah you mentioned like guide but also like you've been doing you've been inspiring in my opinion you've been doing things that are really inspiring to folks and sort of trying to think about it a different way.
So that's really, that's really great.
I think you've already covered so many of the things that, you know, the first things that teams should do to get their analytics right.
You know, the data stewardship of workshop is super helpful.
So that's amazing. Is there anything you'd like to add to that like the first thing teams to do to get their analytics right.
Claire: I think to get your analytics right.
Asking the big questions early is essential.
And I know, you know Avo really encourages this and you know, when a product manager is specifying a new feature, culturally we need to get everybody to take a step back and always ask, why are we doing it?
What do we want to learn from it? Because if you just set out like we're going to do this thing but you don't know exactly why or the goal you're trying to get to it's going to make learning from that data really difficult.
So I think, you know, people getting started with setting up a better data culture at your company start encouraging those questions early.
So when people are setting down to, to make a tracking plan or you're helping them get started using an analytics tool, or whatever else, start them off thinking, you know what do I want to learn?
What questions do I have that I need to answer?
And that's going to get them thinking about like, okay I want to know, you know how are people watching this video?
Okay let's dig into what do you want to know about the video?
I'll help you visualize it and run a chart.
And then I want you to go off on your own and start digging down deeper and figuring it out for yourself.
Stef: Yeah that's amazing advice.
And it sounds like also, you know, even though there is, there are a bunch of documentation online for all of these terms that people need to know when they are designing data and using data, but it sounds like one of your recommendation would also be to have an internal repository of information.
Claire: Yes.
Stef: What do the things mean that we're using?
What is the data stack that we're using all that stuff.
So that's really inspiring Claire, thank you so much.
I want to end this with a sort of a, I guess less of a philosophical question but more of a Claire question, you know what's coming up for you personally and like what's next, and what's exciting for you on your journey either you know, in your work life or your personal life some adventure that you want to share.
Claire: Well I think being a new mother is definitely a new aspect of my life.
You know, I think when I was starting off in tech my career journey was a little bit more ego-driven.
And I think after becoming a new mother it's a lot less about ego and more about helping people and kind of selflessness.
And also, you know, what's kind of stuck with me throughout my career journey is this idea of empathy with our users.
You know, Fender makes some great stuff that encourages people to make music.
You can't really feel bad about the work you're doing when you're helping people to make music.
So I've always lauded this idea of coming to the table with empathy for what our users are doing, what are they going through?
And that's why I think data is so important because it's not just about numbers.
It's not just about behavioral analytics.
It's also, you know, putting yourself in the shoes of these users and helping to develop experiences for them that are going to do things like help them learn how to play guitar and make music.
So I think my journey with, with motherhood through my background in technology, surviving a pandemic, I think we're onto bigger and better things.
We're about cleaning things up. Let's clean up our planet.
Let's clean up our politics. Let's move forward and let's all be nurturers.
Stef: Yay. Thank you so much for those beautiful final words.
Claire, it's been an honor having you with us.
Claire: Thank you so much for having me.
This was really exciting and awesome to do.
Subscribe to Heavybit Updates
You don’t have to build on your own. We help you stay ahead with the hottest resources, latest product updates, and top job opportunities from the community. Don’t miss out—subscribe now.
Content from the Library
The Right Track Ep. #12, Building Relationships in Data with Emilie Schario of Amplify Partners
In episode 12 of The Right Track, Stefania Olafsdottir speaks with Emilie Schario of Amplify Partners. Together they discuss...
The Right Track Ep. #7, Data Translators with Benn Stancil of Mode
In episode 7 of The Right Track, Stef speaks with Benn Stancil of Mode. They discuss the value of analytics for the end user, the...
The Right Track Ep. #6, Domain Expertise with Laurie Voss of Netlify
In episode 6 of The Right Track, Stef Olafsdottir speaks with Laurie Voss of Netlify. They discuss the roles in modern data...