Enterprise AI Infrastructure: Compliance, Risks, Adoption
- Andrew ParkEditorial Lead
Heavybit
How Enterprise AI Infrastructure Must Balance Change Management vs. Risk Aversion
50%-60% of enterprises reportedly “use” AI, but 49% report their biggest barrier to adoption is how difficult it is to demonstrate value. This in-depth interview explores how AI tools have to evolve–and how enterprise expectations must evolve–to make the most of the technology.
Enterprises are known to be risk-averse when it comes to adopting new technologies and new frameworks. They face the kind of regulatory scrutiny, public scrutiny, and in some cases, shareholder scrutiny that smaller startups don’t have to deal with.
The future state of enterprise AI ideally involves having well-trained internal teams in possession of a completely secure, pristine corpus of customer data they can feed into a pre-trained and performant machine learning (ML) model–or models–to produce high-quality results. At the moment, the present isn’t quite like this ideal future.
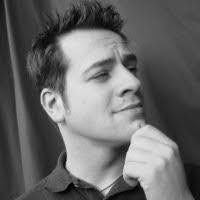
Jos Boumans is a software investor and advisor with decades of experience in developing and maintaining infrastructure. His previous tours of duty include leadership and development roles at Spectrum Labs, Canonical, and early machine-learning leader Krux, later acquired by Salesforce.
Below, he shares his perspectives on how organizations can reach their perfect future. Along the way, they’ll need to identify key challenges, next steps, and organizational changes that will be needed, including:
Resolving the Tension Between Hardware vs. Cost
To set the stage, Boumans suggests an initial wave of vendors may have solved the earliest challenges in building AI products: Collecting and storing data at scale such as via vector databases; running complex and massively parallel compute operations to produce ML models on demand via public cloud providers; and having fast enough hardware to run those models.
Boumans points out that AI compute generally runs on graphical processing units (GPUs), the high-powered hardware chips built to render advanced computer graphics. Not because GPUs were ever intended for AI, but because they just happened to be the best thing on hand.
GPUs from market leaders like Nvidia are the core of modern AI compute. But if and when new hardware emerges–hardware that offers outsize performance at lower cost–we’ll likely see a step function that fundamentally shifts the landscape away from GPUs. In the same way that broadly-available consumer internet and mobile phones revolutionized telecom, a new AI hardware paradigm could completely transform AI infrastructure.
Sensibly Implementing Deployment Configuration(s)
Teams can deploy AI models in a variety of configurations, including on-device edge computing, hosted inference via their company’s own private data center, or using a third-party cloud-based solution. Each has advantages, disadvantages, and associated costs–particularly in terms of building entirely new programs from scratch versus evolving existing infrastructure.
Driving Adoption vs. Hurdles in Compliance, Security, Risk Aversion
Boumans notes that enterprises need to resolve competing priorities and requirements across different departments like development, procurement, and security. In particular, non-technical departments view AI systems as non-deterministic “black boxes” that are difficult to assess for security and compliance checks.
Discussion: How Enterprises Fill in the AI Infrastructure Blanks with Jos Boumans
Boumans sets the stage by pointing out the messy realities of modern AI infrastructure. For example, GPUs became popular for hosting AI inference not because they were purpose-built for the task, but because they were at hand. There are many comparable examples of how modern infrastructure came to be through convenience, rather than through deliberate planning.
AI Infrastructure Limitations: Today’s Affordance vs. a Future Hardware Revolution
Boumans is blunt about the current state of web tooling. “We’re still running IPv4 [addresses] and BGP. Which means that in terms of the conventional infrastructure we have out there, there are protocols, integrations, software that ‘kind of work,’ and from there, we look for affordance.”
Boumans suggests a tension between the huge bets venture capitalists make to innovate versus the capital and operating expenditure enterprises invest every day. A chip that’s 1% better than GPUs won’t make a difference, but 10x efficiency will. The infrastructure veteran also notes the tension between R&D and broad commercialization–which is what eventually establishes new standards.
Boumans notes two potential future shifts: First, the tendency toward affordance across existing tools in the short term, and second, a potential hardware revolution to address the long-term challenges of networking, storage, and even cooling–which could be the next big major in AI infrastructure.
Citing the example of CDNs like Microsoft Azure and Amazon, Boumans notes how impractical it is to try to deliver massive models to the edge. “Instead, you’ll see those providers implement 80/20 solutions, such as splitting up the model, routing, or early decision-making.”
Boumans points out that the cost of scaling inference at the high end will require additional changes. Data centers are so costly to initially build and staff that it’s unlikely many companies will stand up their own new private data halls.
“There's going to be a handful of companies who have expertise in that–and there’s a developing need for this kind of facility for AI. Just adding LLMs into RAM is going to be prohibitively expensive, so there will need to be innovations there–but we might not see those widely available for 10-15 years.”
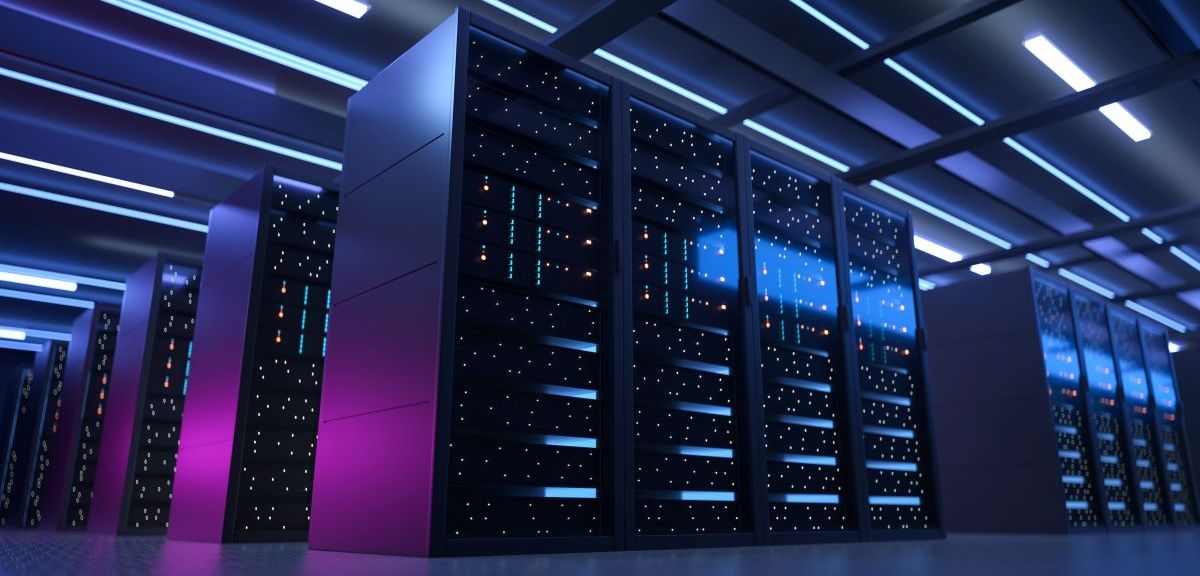
Hardware concerns like cooling may become even more important as the need for AI compute increases. Image courtesy Acceleration Economy
Resourcing Models From First Principles
Boumans advises enterprises looking to establish a strong practice around a machine learning model in production go in with eyes wide open. “For ML, if I feed in more data, the model is likely to come up with a ‘better’ answer, but will also produce a bigger artifact as its output.”
“And this bigger artifact is more expensive to compute, more expensive to produce, more expensive to ship, more expensive to test, and so on. If you front-load with tons of data, you get something that looks very impressive. But then, everything after that step gets harder. And so the tension that you have to manage is having to update this system.”
Hosting inference is potentially more straightforward than running a massive LLM in production, as teams operating models need to carefully manage more variables, such as having a high-quality dataset, inference, and performance. “Big models will have blind spots that I would advise organizations to get very, very clear about.”
Boumans again points to the tradeoff between innovation and affordance. “The closer that you map your process to what you have on hand, the faster your pace to market will be. However, if you’re a researcher, you won’t see this as a path for building the best possible models–which is correct.”
“But as a researcher, your focus is on accuracy and performance–which aren’t the same business questions that enterprises have about who has access to the model, how responses vary at different pricing tiers, and so on. Yes, you can come up with the best model in the world, but that doesn’t mean you can put it in the hands of the customers who want it, with the optimal experience, price point, and support.”
Focusing on Use Cases and Competitive Advantage First
For enterprises simply looking to utilize GenAI apps for productivity gains, Boumans recommends focusing on valuable use cases and competitive advantage. “If I'm a big company and I'm looking to leverage AI to improve our workflows, I’ll look for something that will ultimately make customers buy our product over someone else's.”
The infrastructure veteran notes that AI chatbots were an early success in terms of AI use cases due to known issues with call center-style support–but that a chatbot may not be enough, in itself, for enterprises to differentiate themselves. The path to valuable use cases will likely lie through intelligently using proprietary data in combination with enterprise-grade AI tools.
“It’s possible some organizations will find themselves at an awkward intersection where they have valuable proprietary data, but don’t have all the AI knowledge and infrastructure. But there could also be a second challenge–not even having all the other proprietary data they need in the first place.”
Boumans notes that vendors like OpenAI and Google generally provide useful tools, but for some enterprises, actual differentiation in the market may come from layering their own proprietary data on top of data provided by OpenAI or Google.
“Unfortunately, at that point, you're still beholden to those massive vendors–even if you have all the infrastructure, software, and knowhow–as well as the team to run AI in production. If you lack the data, that’s where the real tension will be. Everyone will try to put a wall around their operation. And vendors like OpenAI will be happy for customers to use their results everywhere–but you won’t be getting their data.”
Obstacles to Innovation: Procurement, Security, Regulation
Boumans is pragmatic about how the security and governance needs of enterprises affect startups. Onboarding individual AI products means procurement obstacles including contract negotiations, budget constraints, and security–particularly in terms of compliance in stricter regions such as the EU. For AI startups, one of the biggest blockers to acquiring enterprise customers might be the lack of an established enterprise presence.
“Example: If I’m already buying Salesforce, I already have the procurement contract done–and now the exciting new AI app from Salesforce is just an extra line item. If there are breach of contract issues, I can sue Salesforce–I’ll have all these rights already built into the contract. I get to bypass a lot of these challenges, without having to go through procurement again.”
“And I think this is definitely where we start to see challenges with the ‘black box’ nature of AI in products. Before I buy, I’m going to want to know: What did this AI product touch? Where did it look? What’s it doing with my data?”
Boumans notes that there are less-established guidelines for privacy and security in AI, leading to regulation in some parts of the world, such as the EU’s AI Act, to compensate with controls that use regulation by enforcement.
There’s a growing list of obstacles in the way of adoption: From regional regulation to requirements for security, compliance, and specific operational checklists unique to every enterprise customer. “If part of your pitch is that your AI product ‘improves’ if it accesses your users’ behavior or your proprietary data, enterprise customers are going to get nervous.”
“If they give you their proprietary data on how they write code internally, how do they know someone isn’t secretly accessing it? You have to prove your privacy and security are compliant. If you can’t, well–there’s going to be a major challenge in adoption there.”
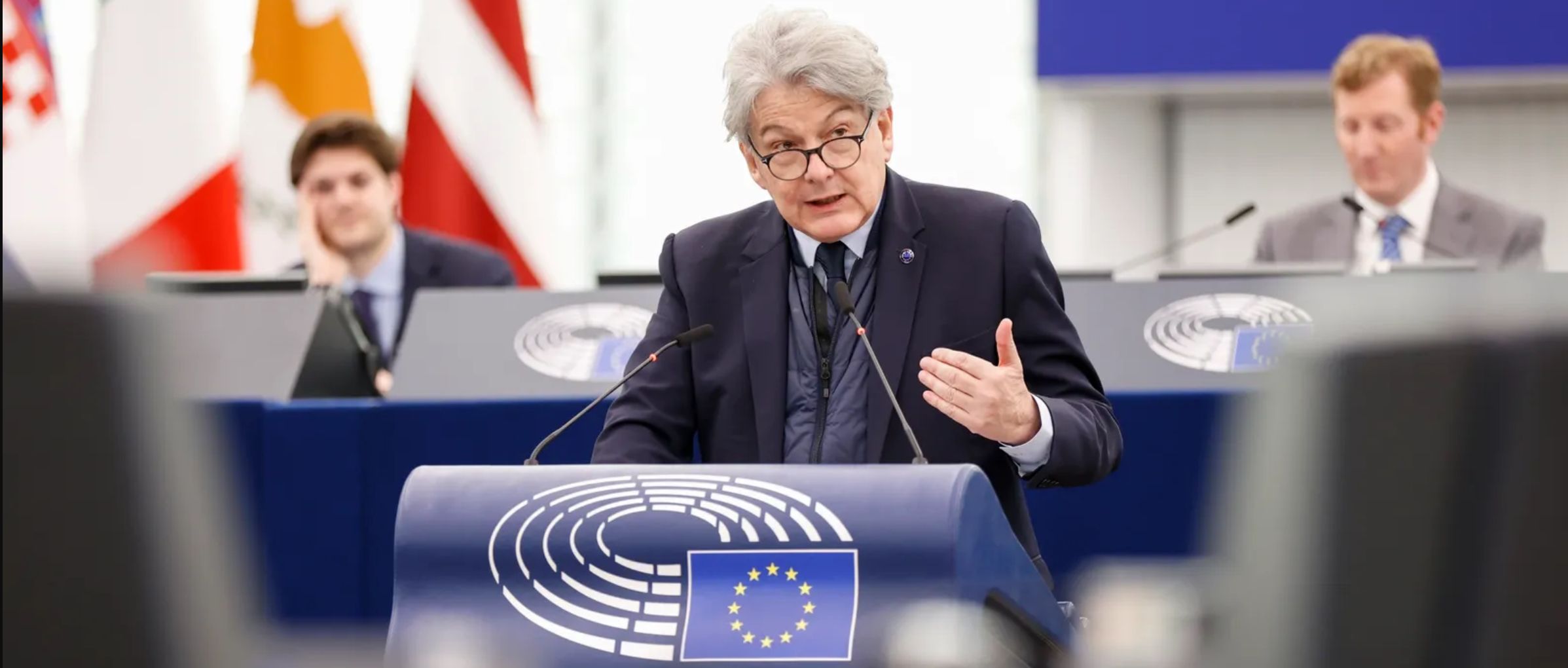
European regulators have passed the AI Act, which attempts to prevent AI abuses by imposing risk-based restrictions. Image courtesy CIODive
Regulated Spaces vs. Strategic Adopters vs. Technically Advanced Adopters
Boumans foresees a few categories of enterprises that may adopt AI products in different ways.
Highly regulated organizations in spaces like government agencies, finance, healthcare, and insurance will prioritize compliance and security above all else. “The conversation for these companies will start with: If you can’t meet my compliance and security requirements, we’re just not going to be able to do business.”
“But, if you can, the conversation will become: ‘Hey, we love what your AI product does, but it can never leave our perimeter.’ Realistically, these organizations have deep pockets and will happily shift both the risk–and the innovation–to a third party first. And then they’ll scoop it up later, if it works.”
Strategic adopters could include the largest, most-resourced enterprises, such as the Fortune 500, who will adopt AI products cautiously. Such organizations might be open to running experiments in smaller markets–running tests in controlled environments but abandoning projects that don’t bear fruit.
Boumans explains, “Strategic customers will basically consider startups to be their external dev team. They’ll say: If your product and processes work, we’ll use them. We have the money for it. But these organizations will be the market laggards no matter what.”
Among enterprises, technically advanced adopters might offer AI startups the most opportunity. Enterprises that are more technically mature may experiment with AI solutions to test their feasibility, and run internal build-versus-buy conversations to understand the complexities of a use case through a third-party product before deciding to buy, or to build, their own.
More technically-advanced enterprises are likely to be more curious, and will consider the opportunity cost of building their own version integrating your AI products. They are the ones most likely to engage, and say: Let’s see what your product can do for our business.
Content from the Library
Enterprise AI Infrastructure: Privacy, Maturity, Resources
Enterprise AI Infrastructure: Privacy, Economics, and Best First Steps The path to perfect AI infrastructure has yet to be...
MLOps vs. Eng: Misaligned Incentives and Failure to Launch?
Failure to Launch: The Challenges of Getting ML Models into Prod Machine learning is a subset of AI–the practice of using...