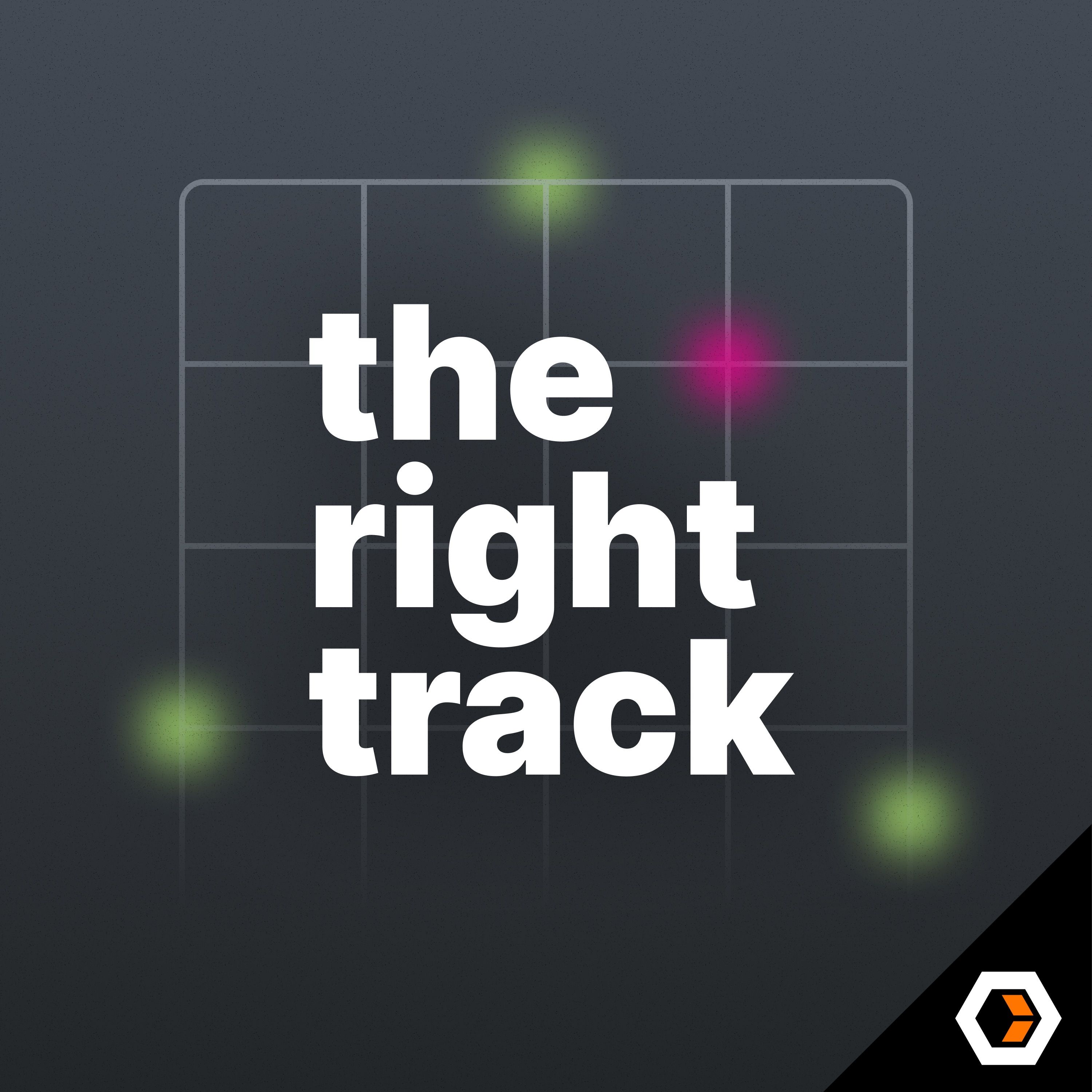
Ep. #5, Intangible Metrics with Elena Dyachkova of Peloton
In episode 5 of The Right Track, Stef is joined by Elena Dyachkova of Peloton. They discuss the intersection of fitness and data, as well as the tools Peloton uses for data management, governance, and analytics.
Elena Dyachkova is Senior Product Analytics Manager of Peloton.
In episode 5 of The Right Track, Stef is joined by Elena Dyachkova of Peloton. They discuss the intersection of fitness and data, as well as the tools Peloton uses for data management, governance, and analytics.
transcript
Stefania Olafsdottir: Welcome to The Right Track, Elena. Great to have you here.
Elena Dyachkova: Thank you. I'm super happy to be here.
It's my second podcast recording ever. So definitely a little nervous, but also excited.
Stefania: Yay. You have absolutely nothing to be worried about because I know you have a really good story to tell us.
To kick things off, could you quickly provide us with an intro?
Tell us a little bit who you are, what you do and how you got there.
Elena: Yeah. My name is Elena Dyachkova.
I am currently a senior manager of product analytics at Peloton.
I lead the product analytics function in the company and I've built it from ground up.
So that's my proud moment.
But outside of that, I am a huge track and field fan, and that was my career for many years before I switched to analytics or product analytics.
And I also have a two year old. He is my whole life, so I'm also a mommy. So that's me.
Stefania: I love it. How did you go from being in track and field to being in product analytics?
Elena: It's a bit of a long story.
My educational background, there's an economics or mathematical methods and economics.
So I've always been in the data field to some extent with more academic focus but I did all my research, all my thesis work in sports because track and field has been such a big passion for me as a spectator and I did some track in college as well.
So I spent many years after I graduated working to make the sport more popular, make it more fun, more engaging.
I did all sorts of things from communications, to event organization, sponsorship, sales, athlete relations, all sorts of stuff.
But I then moved to the US to pursue sports further.
Figured out how to do it the Western way, I guess, but it just so happened that I started off in the US also in sports and sponsorship measurement at the company called Repucom, that got acquired by Nielsen later.
And from there, I transitioned to more general analytics and research.
And then from there to product analytics, just through partly it was just luck and some internal reorgs that just opened up new opportunities for me and gave me chances to work with the product team and then discover what the product management was in general.
But at some point, I felt a need to reconnect with my passion, which is going back to running fitness industry and at that time Peloton announced the creation of the running content and launch of the tread, a relaunch of their mobile app and all that fun stuff where I felt like with my experience in running, I could bring something to the table there and that encouraged me to join the company.
At that time I was already in product analytics or in product management more broadly.
So it felt like a perfect fit, continuing to do what I love, which is data and also be in the industry I love, which is fitness and sport.
Stefania: I love that story. I recently also interviewed Claire Armstrong at Fender, and it's a similar story.
For her, she had a really strong passion for the music industry and people on their music journey.
And then she had a background in UX and merged those two.
So I wonder if that's a theme for particularly, I guess when people are building digital products, they already have a passion for what they are observing their users.
And then, maybe especially when you're going into product analytics, really wanting to tie what you already know with facts and see how that is and how that can be attributed to the product that you're building.
Elena: And I think it's a big help being able to relate to the user as well.
And just relating to the mission and understand what you're bringing to the table.
I think that also can be dangerous, especially in analytics or research fields when you're trying to do me search and it's important to always-
Stefania: Me search.
Elena: Yeah. To check yourself that, hey, probably you yourself are a specific user persona as a user of the product but you don't represent the entire population.
So it's good to have some intuition but making sure that you're not just trying to validate your own viewpoint or your own feelings as a user when you're looking at the data.
Stefania: That is a really strong point.
How do you keep yourself in check there?
Elena: I think I just make a point of thinking about it when I'm using the product and I notice something that maybe doesn't feel good for me.
I always try to be like, "Hey, you are that one person and you know more about the product than other folks."
So you use it in a specific way.
And even as a user, I'm probably not representative of more general Peloton user base since I've run track in college and there are specific training method that I'm on board with.
I'm very metrics-driven and I understand that the majority of our user base probably aren't quite as metrics-driven.
They're training plan and PR driven, but more so just trying to have fun.
And I think also having an experience where I had an intuition about something and then I checked it then the data and it didn't feel like my opinion was representative and just it gets in some of those cases under my belt to validate the point that, hey, you can't speak for the user base just check your intuition.
Stefania: This is bringing up a couple of questions.
I mean, it's also a fun addition to your backstory that you used to be a very metrics-driven athlete.
Elena: Yeah.
Stefania: So you're now helping other people use a fantastic product and become more metrics-driven and you are also helping product teams become more metrics-driven in building those products.
So that's really interesting.
But in addition to wanting to check yourself and being aware that you are not the user base, the entire user base, do you also feel like a missionary purpose that you know that this has helped you a lot and you would like to help other people maybe become more metrics-driven?
Elena: Yeah. I think the general concept of being more metrics-driven is a good one, but I don't think that being driven by metrics in a narrow sense of, here's what my output is, here's what my pace is.
I don't really think that's for everyone. I think for more people, the metrics are more intangible, such as, I guess, how good am I feeling on a scale of one to 10?
Maybe something that isn't in the app, but maybe something that's in my head that's also a metric.
And I think that's ultimately more important than metrics such as your output in a ride or your pace in a run.
We feel differently on different days. We didn't need different things on different days. So it's more so that intangible feeling within yourself. After you complete a workout, how do you feel?
Do you feel like you have achieved the purpose, which on some days could be, hey, I'm really maybe very overwhelmed and I needed this, right?
To just clear my head and maybe I wasn't going for a personal record but then on other days, maybe I was.
So it's given our users the flexibility to find the right content and the right features for the purpose that they have for a specific day because ultimately Peloton isn't about weight loss, it's not about necessarily health improvement per se, but it's more about that empowerment and convenience, empowerment and community.
So it's more of those intangible metrics.
Stefania: No, that's a really beautiful take on it. I love that.
Peloton and the Peloton users are really lucky to have you on board I have to say.
To dive a little bit into the data culture, we here on The Right Track, we are really passionate about helping people build good data cultures.
To kick us off into that segment, can you share with us an inspiring data story?
Elena: Yeah, definitely. I wanted to share a story that isn't fully my story.
My colleagues they were at Peloton before me, they kick started that but ultimately data helped create some of the most important community features that we have in the platform right now, which is the Here Now leaderboard and the sessions feature.
Initially, we only had all time leader boards and I was a part of the main value proposition at Peloton that when you're doing a class, you don't feel alone because in the leaderboard, you see everyone who has done the same class all the time and you can compare your output to other people's.
See how you stack, maybe compete if that compels you.
And at some point, basically when our user base was smaller, there was more fragmentation of different users would pick different classes.
There wasn't as much users working out at the same time.
But at some point, one of our product leaders said, "Hey, let's look at the data and see if, at a given point in time, there is an overlap of users doing the same class."
And when we ran the numbers, we did see that at that point and most popular types of the day, we saw a significant overlap where there would be a same class that multiple people would be doing at the same time.
There would be overlapping by at least a little bit.
So with that we said, "Hey, why don't we make our leaderboard more engaging in a sense that you still can't see everyone who's done this class all the time, but you would also be able to switch to the Here Now leaderboard, where you would actually see people who are doing the same class right there at the same point in time and that way you also get the way to interact with them."
So you can send them high fives, they can send you high fives.
So it makes it even more, mimicking the studio experience a bit more for folks who are really --for whom it's really important.
So that's how Here Now was born.
And then as our user base continued growing throughout the last couple of years, at some point we decided to look at whether people are even starting the class, not just overlapping maybe by a few minutes but even starting the same class at the same time.
So we ran the numbers again and once again, we saw that the most popular times of the day.
It was indeed the case that there was, a decent amount of people starting a class within the same couple of minutes, which means that they wouldn't be able to just interact in class, but they can also actually compete.
In Here Now leaderboard, sometimes there'll be people, you just type in a class they're already almost finished. So you can't really compete like that.
But if you're starting at the same time then you can also compete.
So we recently launched the sessions feature where it's similar to video gaming concept where, it starts every five minutes.
So you sit in the lobby until that start time and then you start at the same exact second.
There's a few more people. It can be five, it can be 100, it can be more depending on the class and the time of the day.
And then you start altogether, you get that really small leaderboard really that you feel like that you're all in there together.
And then, a lot of people like that for the competition aspect, a lot of people like it for the ease of planning to ride with friends because you know that you're starting in the same exact time.
So two of our most important community features were powered by intuition with the data.
Stefania: That's amazing. I love that.
And so I'm curious to understand a little bit more about how this was discovered and how it came about.
And it sounds like someone was looking at time of day, spike usages and things like that.
Can you share a little bit about how the discovery happened?
What type of role was involved in that discovery and who was collaborating and things like that?
Elena: Yeah. The initial Here Now discovery happened before I joined.
So I only know anecdotally but the product managers that were there before I joined the company were extremely data-driven as well.
Even before that they had a specific product analytics function, they had a pretty good tracking set up, they had some self-service tools so they were actively looking at the data.
And similarly, they were constantly talking to the users to gauge what the gaps were, what the opportunities were to add to the user experience.
So it was mostly that, hearing from the users of what was missing, seeing that users wanted a bit more interaction and stronger community bonds.
So that was born out of that where, the product managers were able to operate with the data, already had tracking in place of who was doing workouts at what point in time.
And then for the sessions, it was a similar thing.
The community is a part of our mission.
So there's a dedicated attention that people pay to that area of experience at all times, there's continuous research happening, continuous strategy refinement.
So it's the same concept where we're constantly talking to users and figuring out where are the opportunities, what is missing, how the social dynamics change on the platform with the increase in the user base growth, right?
So it was the same thing where folks were starting to say, "Hey, even the Here Now leaderboard has now become really large. So if I'm riding with my friends, sometimes it's hard to find them on the leaderboard because I have to scroll because there are so many people."
So with that, we tried to think, hey, what are the possible solutions for that use case, for that user problem?
And more purposefully looking at the data to get the sense of the direction that we might go.
Stefania: Amazing. Thank you for sharing this.
A couple of points that I think are interesting here.
Number one is, it's a really good point that before even you joined and building up the product analytics division of the company, the product managers were already data-driven.
So that's one part that I'd like to ask you a little bit about.
And then the other, just get a little bit of further insights into how this cross-functional data review group has developed over time.
But starting with the first point, how much of an impact do you think it has had on Peloton's data functions and the product analytics functions that the product leaders were already data-driven before you started the product analytics section of the company?
Elena: I think that was huge ultimately because the habit of incorporating data into your decision-making is something that maybe doesn't come naturally to everyone, right?
So when I came on board, I didn't have to convince the product managers at least that, hey, the data is important, let's look at the data.
But there was already a bit of a habit around that, a bit of even including that and hiring process and talent review process that, hey, product manager needs to know how to interpret data, needs to know how to use data, how to incorporate it.
So I think that was huge because ultimately, I still had to prove the worth of the product analytics as a separate function as opposed to, "Hey, let's just build the self-service tools and PMs will do it themselves."
Which I think is fine on some stage, right?
But obviously that would hit some complexity limits where, some analysis would really benefit from having someone with the great depth of skill in data science and statistics.
It was a pretty smooth sailing for me in terms of the engineering knowing that the implemented tracking is important.
The product managers knowing that looking at data is important.
Already having some infrastructure in place around that. I think that was great for me.
Stefania: That's amazing. Yeah, this is great.
I'm also curious to hear a little bit more about--
Because I mean, this is a really great way of doing things basically for the important things, for the important features and the important missions of the product and the product teams to have these cross-functional review groups as you call them that were there to discover the session analysis or discover the data that drove the session feature.
Can you talk a little bit about how that cross-functional group review process has evolved?
How often does it occur and how often did it use to occur? Who sits in on it? And things like that.
Elena: Yeah. I wouldn't say it's necessarily a cross-functional review, it's more so just the way we work where, nothing happens without looking at data at this point.
We have dedicated work streams around various areas of this experience or product lines that have engineering representation, product management, product analytics and design.
Design also includes user research in that case. So it is pretty common for before solutioning on a specific user problem to have both analysis if we have some existing user interaction data that can power some insights, which is not always the case because a lot of the product development happens for something that's completely new.
So we might not have necessarily quantitative data to help with that decision.
But if it does, looking at the data from our existing user base, then similarly leveraging user research for qualitative insights, which can be focus groups, can be concept tests, can be surveys.
And that similarly after something has launched, having some sort of a checkpoint for the dashboards review, how things are performing, whether our hypothesis are confirmed or not and similarly incorporating qualitative insights into that as well to gauge more of a user satisfaction, which is an important layer on top of just pure usage data.
And then for bigger initiatives, we also have strategy team, we also have consumer insights team that can do larger scale studies with more of a business focus.
Also, qualitative, quantitative surveys and things like that.
Stefania: Amazing. This is super helpful.
And I am assuming this is also very inspiring to a bunch of different people.
I hope this is inspiring some of our audience here.
I definitely want to touch on this a little bit also later in the episode, but before we do, can you also kick us off with a frustrating data story?
Elena: Yeah, definitely. It's going to be not Peloton related at all.
But in one of my previous companies that I worked in, in the audience measurement industry, we were going to launch audience measurement product in several international markets in partnership with another company where, we provided some methodological pieces, they provided some of the first parts of data, which married together would provide a cross platform measurement figures for a set of websites basically for our B2B.
It's a B2B model so it's not for end-users but for business clients.
And as we were about to launch, we were buttoning up the methodological piece and we've discovered very close to launch unfortunately that there was an artifact of international markets having, some of them had lower population than the US and the panel data, especially in specific age, gender groups was so low, the audience shares were so low that when extrapolated, it just gave weird data where the audience shares across age, gender brackets did not sum up to 100, but they actually were above 100.
And that was not a fun discovery with a week or a couple of weeks before launch.
I think we also made the mistake of not being very transparent with our partner at first and assuming that, hey, we understand that's a methodological blip but there's not much we can do about it because the population surveys happen every year, every five years in some markets so it's not that we can just go ahead and do a new one right away in two weeks.
So we assumed that the partner would either won't notice it, or would be okay with it, which was definitely a wrong assumption.
It was definitely a very stressful launch, but it happened with some methodology tuning and some model tuning.
We had some brilliant data scientists and statisticians on the team, but it was definitely an artifact of trying to rush something to hit some contractual obligations.
B2B is a whole different beast.
Stefania: Yeah, exactly. So this is a case where you were working with an external partner on delivering data.
Elena: Mm-hmm (affirmative).
Stefania: Yeah, that's really interesting.
And it has actually an interesting input into a later discussion on the podcast on, how does the data team within Peloton or within organizations that you've seen work with the stakeholders?
Because you do definitely often see, or I've seen it tons of times, and so many people talk about that, that data almost is a silo.
So what you were describing right there is something that could so easily happen when the collaboration and the partnership doesn't really feel tight and the relationships haven't been built.
Elena: Yeah.
Stefania: Thank you for sharing that. That's great.
So on that frustration note, building data cultures is a lot around building data trust, data literacy, data accessibility, and things like that.
And we will touch on a little bit later, what does it mean when people say, "I don't trust this data."
But before we go into that, what would you say is the most common way that analytics break?
Elena: I don't mean to deflect that question, but I think my team has been pretty good about doing thorough testing before something goes into production.
So I would say in production, our data is pretty good.
I wouldn't say it's broken much, but we definitely do catch a lot of small inconsistencies during the testing process.
And a lot of it is as simple as double spacing instead of single spacing.
Or casing is off, or data type is float instead of an integer.
So a lot of that which is, definitely very annoying.
It doesn't make the data completely broken. It's still workable, right?
But that definitely causes a lot of discomfort if it were to go into production, it causes a lot of discomfort and self-service tools, a lot of having to have additional data cleaning scripts.
So it's creating a lot of busy work and similarly, and even in the development process, it creates a lot of back and forth testing, edit, back to testing that can be avoided, right?
So it's annoying, but ultimately not the end of the world.
So yeah, I would say that those are the things that I see the most often, just those little inconsistencies.
Stefania: Yeah, exactly.
I mean, so you now have these thorough processes for queuing the data and I've often heard people talk about the classic UAT, User Acceptance Testing for analytics events.
And that's something that I don't think people start building unless they see the need to do it.
And obviously it sounds like you were already catching some of these issues in QA, but there was a reason why you started doing it.
Can you talk a little bit about how that happened? How did you develop those QA processes and why?
Elena: Yeah, definitely.
I think it's really hard to rely on engineers and rightfully hard.
I wouldn't say it's the, no negative connotation just because engineers aren't the end users of the data to the same extent as a product analyst or a product manager would be.
So with just having more platforms, where when we had just the bike, right?
It was one engineering team implementing everything.
So it was very consistent but as we started growing bigger and we added mobile apps and tread and TV apps and the web app.
And we tried to implement everything in a way that cross platform analysis are possible because a lot of the features live in multiple apps at the same time so we have to be able to look at the user's entire journey.
So that's what spurred the notion of, hey, we need to have some tighter taxonomy principles and tighter quality checks in place so that we're not in a situation where, the same exact interaction on different platforms is captured with an event that has slightly different naming.
One, maybe some event properties are lowercase or uppercase.
Maybe here is float, and here's integer because I send data in the self-service tool, you would very easily see that this just looks like a mess.
And it's going to create a lot of annoyances for folks down the line.
So we established that as a principal early on that, hey, we're going to redo our taxonomy in a way that's establishes the core principles for how we want to name events, how we want to deal with casing and appropriate value data types and things like that, put it on paper and we're going to establish a process around, who creates the design?
Who signs off on it? Who writes the tickets?
And then who tests the tickets?
And I think we've tried having a QA team be a final sign-off PMs in some cases, engineers in some cases but we found that the analytics team is the best equipped to do so, because we'll be the ones who would be doing the analysis, right?
So we already have in mind how we're going to use that data so we have a vision of how it should look.
And then similarly, while doing it, we accumulate the experience for each cases where we can already foresee that, hey, if I test that interaction from this specific menu in the past, maybe it wasn't ideal.
So let me try that again. I just feel like where we're doing the most thorough job and I think it's by design, I don't necessarily think it's wrong.
That said, I do think that there is definitely some space in analytics industry to figure out a more automated protocols for testing as well because one thing is, you're still relying on a human call of how to test and what to test, but we can't know everything that could break.
There can be regressions where you're texting your UAT the new feature, but then you don't notice that it affected some existing features tracking implementation.
So I think there's definitely a space for having that another layer of QA that is more similar to general software QA, unit tests and stuff like that.
So yeah, it would be nice to have all of that in place.
Stefania: Exactly. I mean, it's really interesting to see how just the product development industry has evolved.
It's been, maybe 20 years since it became fairly normal to not have waterfall product development, right?
Where the developers would receive design specs and they were supposed to implement it.
And moving that into being more of a cross-functional collaboration and to shipping a product.
And that's become normal and mainstream now in the last five years.
I think most product and development teams, they won't accept anything other than that.
And I feel like in the last five years, analytics has more and more become also a part of this.
Maybe five to 10 years ago, analytics used to be a complete waterfall thing and the design of the analytics would happen in that data silo team and just the developers would receive specs.
And now more and more we're seeing where analytics has also become more a part of the entire product release journey.
Is that similar to what you've seen also?
Elena: Yeah, definitely.
I do see a lot of value in that embedded model where, product analyst has multiple touch points.
That's one of the reasons why our team sits on their product management vertical as opposed to in the central data team because we feel like we need that visibility and we need that connection being embedded throughout the product development cycle.
From mediation, like I said, "Hey, let's validate some of the hypothesis around user problems with the data."
"Let's validate some of the solutions that analysts would also work on and provide an input for our KPI setting before even the development starts than analysts needs visibility into the design, I guess, at least at the design handoff stage so they understand that designers thinking around, how that specific design answers the user problem."
"Are there any hypotheses around some user flows that might be questionable or that are risky so that we can incorporate that into tracking?"
Making sure we can measure that, or maybe we can suggest an approach, a release approach, maybe a specific test experiment as opposed to a wide rollout.
Then similarly, coming back at the release measurement stage and then just remaining on that ongoing track and so we can also notice where trend changes or maybe a bug or something like that.
So we can catch that proactively and bring ideas to the table on how to refine something or how to fix something. That's how we try to work at least.
Stefania: That's amazing and super inspiring.
I definitely want to ask you a little bit more about that as well later but a little bit more on the broken analytics and your drive for designing the QA process.
I mean, it's a really big statement for any company to say that, your data isn't broken in production that much.
And it speaks so much to the quality of processes and tools that you've built internally to solve this.
So I think most people will be very interested in hearing a little bit more about it.
And you talked about the things that you would typically see in your data before you develop the QA processes.
And you talked about, those are the same things that you are currently catching in your QA process.
And you mentioned the discomfort of broken data.
The busy work, the testing, the edits of the tracking, the back to the testing, all those things.
Can you talk a little bit more about the discomfort when you actually manage to not catch things or before you managed to catch everything in QA?
You mentioned social analytics tools wouldn't function properly.
What else would have been an issue?
What was slowing down the team enough for you to build these processes?
Elena: I think it's mostly having cases where something wasn't implemented cleanly enough at launch, or maybe not implemented at all in time for launch where, we just try to avoid those things and it's better to track more than we need than not to track something really crucial.
So we've just started to be more diligent about it and having a specific set of requirements that can be launch blockers potentially that if something isn't clean enough to go out because it doesn't make sense to release something if you can't tell how it's performing, right?
Stefania: Right. Exactly. Yeah.
Elena: So that's probably a part of it.
Another part of it is just, all of that happened where the team was still in the very early stages.
So to be honest with you, there is still a lot about establishing our value proposition, making sure we get enough resources to grow the team as well.
So it was just really important to not drop the balls and get ahead of any potential problems.
Another thing is that because we're embedded, right? So there are other data teams in the company.
So another thing is also being able to officially collaborate with those teams.
We don't want someone coming to us and say, "Hey, this is wrong. You're not doing your job right."
And similarly, we'll probably do the same if another team that touches some other data was lagging behind and collecting something, right?
So we also try to be mindful of the dependencies and making sure that, all the crucial data points that we know other teams might want, that everything is implemented cleanly with the release and communicate accordingly.
Stefania: Yes. You're touching on a point that I am very excited to talk about.
I meant to go in a different direction right now and stop by on the way back, but I think this is a really good segue into it right now.
So you're touching on what is involved in releasing analytics for every feature release.
Who is involved in planning it, who is involved in implementing it, who is involved in queuing it, analyzing it and prioritizing features.
Can you talk about what that entire process looks like for you today?
Elena: Yeah. It's pretty much 90% owned by my team, by product analysts in this case.
So product analysts would normally, look at the design, figure out what's happening, look at the KPIs that they've planned as well and add design hypothesis to that list.
And that's what goes into the design, the instrumentation design that an analyst prepares based on balancing the desire to track everything and also knowing what are the KPIs?
Everything that needs to be tracked for the key feature KPIs, that's what we call P-zero.
So it's something that could be a launch blocker if it's not clean and then prioritizing the rest of the desired events in the descending order.
Something to validate key design hypothesis or something that's just nice to have in the spirit of, let's just track everything in case we want to revisit that later.
Obviously when they can, when we have resources, we try to track every new interaction, everything, every new screen.
So an analyst prepares that design, very detailed. We do it in a spreadsheet fashion where we lay out all the requirements and the priority status there. Then we have a peer review stage where another analyst on the team would be required to have some eyes on them from perspective that another analyst might see something that the first analyst didn't think about based on their previous experiences. So it's always good to have two pairs of eyes or make a suggestion on taxonomy or how to make it more concise, which properties to add stuff like that.
So that's the stage that we always go through.
And then after that, the analyst writes the ticket for developers, hands them off, developers implement the tracking, it goes back to an analyst, the analyst does the UAT and then the next stage would be for the analyst to build the launch dashboard.
In some features, depending on the depth of the feature and whether it's creating a new production data model, not tracking-wise but more so user experience-wise, we would involve sometimes other data teams if we expect that to affect.
Let's say an example would be, we recently launched a new scenic rides and runs.
Initially we only had the time-based ones where you say, "It's a 10-minute class, 20-minute class."
And then a month ago we launched distance based ones where it's not a 10-minute class but it's a 5K class.
So however long it takes you to complete the 5K, that's your class length.
So in that case, hey, this might affect some of the official KPIs or the way that maybe a content consumption reports look for the content team or the data science team.
So in that case, we would also include those teams and the considerations around design and making sure that we capture all that they want.
And we also get the visibility into how they think about modifying their official reporting.
So that's a little bit ad hoc.
It really depends on the project for something that's, I don't know, adding a new tool tip would probably wouldn't involve other teams, but for something big like that where it's a new class type for example, then definitely we would take a more cross-functional approach.
Stefania: This is so thorough and a really great description.
Thank you for sharing it. I think it's very actionable for a lot of the people that are listening to this.
It definitely sparks up the question about what your org structure looks like, particularly, maybe data-wise and it also sparks the question from me on, what does it mean to have a defined piece hero feature KPIs?
And how much do they tie to your global Peloton KPIs?
Do you have those as well?
And then always for the KPIs for the feature, who designed that and decides that?
I'm guessing the product manager, but would love to hear your thoughts on that.
Then definitely another question is, how do you strike that balance between tracking everything and what makes sense?
But let's start with that first question about the KPIs.
Elena: With the KPI, it's definitely a collaboration between a project manager and an analyst where a product manager would mostly speak to the very clear definition of the target user persona and the user problem and a desired outcome of how that feature would play into a specific, either strategic or a business initiative.
And then the product analysts would be the person who would actually translate it into metrics, like measurable definitional formulas if you will.
Stefania: Can you give us examples of those?
What is an example of how a product manager might frame a KPI and then how a product analyst would translate it?
I know I'm putting you on the spot. If you can think of one.
Elena: I will go pretty general, just not to disclose anything I shouldn't disclose.
But let's say, the sessions feature, right?
We probably don't think that that feature is going to be compelling for everyone because we know that some users don't even look at the leaderboard, right?
So a product manager would speak to that like, "Hey, we want these features for that specific type of user."
Maybe a user who'd already engaged with something similar or a user who, I don't know, looks at the leaderboard or a user who likes to set PIs or something like that.
And then the product manager would also say a hypothesis that, "Hey, some features are really more depth-focused, where we just want a user to engage with more apps maybe, or engage with more class types or some features are more consistency focused where, this is not really about engagement with a new thing, but more so continuously engaging with the existing thing at a higher rate, maybe."
So the product manager would help formulate all of that.
And the product analyst would then say, "Hey, this is going to go into the depth bucket."
The depth bucket normally, a lot of the metrics are around average number of X that the user does per month or something like that.
Or if it's more of a consistency bucket, then there could be some sort of a stickiness metric or some retention metric.
So they will just help formulate that and build-in the right audience definition into that metric.
So that's how it goes, but of course with features, it's a bit more complicated because expecting that every little feature that you launch is going to improve retention or improve conversion, it's a little silly.
Product is not one feature, especially if it's something really small, it doesn't really do anything for the user.
In many cases, it's more so how it plays with the rest of the product and how it fits to it, right?
So not all the time, we would say that, "Hey, this feature is going to improve retention necessarily."
But more so, "Hey, this is for this target user."
We do think that that's something that they're going to do every week or every month.
So then the KPIs for that specific feature might be more tactical, more adoption focused, right?
Adoption satisfaction focused.
Well, for something bigger, it might be something that really ties a bit more clearly into a specific business KPI or a specific strategy.
Stefania: Amazing. And you're touching on the address thing, right?
The next question, the strike a balance.
Because you also mentioned earlier when you were talking about data stories, it's such a great point that sometimes when you are preparing a feature release or a design and you want to make decisions on what should be built next.
Sometimes the right data exists and sometimes it doesn't.
And I am personally a big fan of defaulting rather to less is more just because it can become so overwhelming.
And if you create too big of a slice of things to track, just random pieces of that slice will get tracked.
It sounds like you're solving that of course, with priorities.
This is P-zero, P-one and all that stuff, but can you talk a little bit more about how you strike that balance, particularly when you said, obviously you want to track more and have those insights later rather than not be tracking something crucial, but how do you think about that?
Elena: I'm definitely a fan of tracking everything if possible because sometimes when you're just launching on a journey of, in measuring the success of something, you can't necessarily think of every little thing that's going to actually matter.
So I'm a big fan of creating that opportunity for analysts or data scientists to have as many data points as they would need.
But as you mentioned, it creates a risk of, implementing something that's maybe easier to implement but not super important versus not focusing on something that's really crucial.
As well as just, the constraints about how much data we can store, how a lot of tracking can also mean longer latency for users if you're piling up a bunch of as the case in something.
So it's always that sort of an exercise where prioritization is really important, right?
So we do want for all the P-zero stuff that's related to the key KPIs or the stuff that's related to the most risky paths where we have a hunch that maybe, "Hey, this path might be confusing for a user."
Or, there could be some more quality component to it that, "Hey, maybe we think that there's going to be some performance risk for the feature or whatever."
Making sure that is flag that's major or P-zero, P-one and that goes out with the release, no matter what.
But we still strive to implement all the rest of the stuff.
It's just sometimes doesn't come at the release time but it comes as fast follows depending on the resources.
And then another layer on top of it is also, checking in with engineering on feasibility, with something which seems to be a nice to have but it's actually super convoluted to implement.
It's going to take a week to work on, where an engineer would probably say, "Hey, let's scrap it. It's just not worth it."
So layering on the feasibility piece to it, and keeping an eye on what the engineer feedback is in terms of the user impact as well.
Like if something is going to make it slower, the app slower for a user or something, then we'll definitely scrap it.
Stefania: This is really a good point. The prioritization.
You're prioritizing the P-zero and then you build hypothesis around what are the risks of the feature?
That's a really point. Prioritizing those things instead of just tracking everything.
Prioritizing things that are really important to understand.
And those typically take some thinking.
You need to think a little bit to figure these things out.
So that's really good. I love that.
And then you mentioned the things that happen if you track too much is a really good point.
The easy things get implemented, could cost latency with the data and the data for the end user.
And then the third thing I think is often one side effect of tracking "Everything".
It maybe can slow down data discoverability and data literacy in the company if there is a lot of data that you don't really need, what do you think about that?
Elena: That's something that we're working on, on how to make our self-service tools a bit clear for the user.
I think that's actually the biggest pain point for someone who is not on the product team.
For example, if they're trying to do some analysis in our self-service tools as to figuring out which events to even use to look at what they're trying to look at.
One thing, we try to solve it pretty well with our taxonomy, where we actually go more descriptive routes.
So we don't do tracking such as button clicks, page views, right? That's not helpful.
That's maybe easier on data engineering side, but not on the data consumer side.
So we go fairly descriptive where we try to have some reference to the feature name or something that's fairly straightforward for an end user and they can figure it out.
They can try to search and they would most likely find what they're searching for.
And then adding more annotations, categorizing events a bit better, so users can navigate it a bit more.
But I do think we still have quite a big amount of work to do in that area to improve discoverability in self-service tools.
Stefania: Well, this is a really good point and I love your point about the descriptive event names.
Using what the intention of the user is rather than using button click.
And it's really insightful what you said, which is the button click event might be easier for the data engineering side, but it's definitely not an issue for the data consumer side.
Elena: Yeah, for sure.
I'm sure data engineers, they don't like doing unions of tables or something.
For them it's easier if it's all in the same shape and leaves in the same table.
But unfortunately, that doesn't quite work the same way in self-service and SaaS tools.
Stefania: Exactly. I mean, you talked about doing unions of tables.
The other thing that you have to do, if you have an important user intention, it might not always be represented with the same generic event, like button clicks.
It could be a different event in another case.
So you still have to do the union except you don't know what the event means.
Elena: Yeah. Exactly.
Stefania: That's super insightful.
Before I dive a little bit into your tool stack, and you talked about your self-serve analytics tools.
I know we won't specifically dive maybe into your specific tools, but your type of stack I think is really insightful.
Before we dive into that, can you share with us what your org structure looks like?
That's something that I am always passionate about hearing from, especially exceptional product teams like yours.
How does data work with product and engineering? I mean, you've already covered it a little bit.
Are they integrated with your product teams?
Do they sit in a separate team? Who do they report to? Et cetera.
Elena: In general, Peloton has a hybrid model, where all departments or verticals I'd say who need day-to-day data support, they are encouraged to create their own embedded analytics teams, which is the case for us.
Our product analytics team sits into a broader product development teams, a team that also has hardware engineering, software engineering, product design, and product management.
And we specifically report into the review of product management.
It's mostly, if your team really needs that heavy day-to-day data support, that they just makes sense to create an embedded pod so that you can prioritize your resources independently so that the team can be looked into your roadmap discussion and understand what's going on there.
For product, I feel like it's extremely important, especially as we're trying to be more agile and move faster and experiment more.
I think that becomes absolutely crucial.
And then we also do have a centralized data platform layer that sits, I think, within the CTO org.
So the broader tech org that is responsible for architecture, infrastructure tools, data warehousing tools, data lakes and stuff like that.
That way, all teams operated within the same architecture, right?
But then each team deals with their own data that's more relevant for their function.
Each team creates the data to some extent that is more relevant for their function and they can be more independent when it comes to resourcing and things like that.
Stefania: That's super helpful. That sparks two questions for me.
One is, how does the product analytics team then work directly with the individual product teams?
Do you have a product analyst allocated to different product teams?
And my other question would be, do all the different data teams in the company, the data parts within different sectors of the company, do you have any knowledge sharing or a discipline sessions and things like that?
So let's dive into the first one. Directly product analysts with product teams.
Elena: I mean, we have only two product teams, my team, product development team and then the e-comm team.
So it's really just two product teams. And each of us has a separate product analytics team.
Within our team, we have more work streams, which is not like a team, but that's more or so an initiative, right?
Some of them are very short lived. Some of them are really long-term, right?
And then each analyst would have a couple of those that they support.
So they would have a go-to product manager, go-to development pod, go-to design pod.
But those aren't permanent in the org structure. Still, all analysts report to me via their managers.
And we all report to the VP of product management.
So it's more just an operating model rather than org structure.
Stefania: Nice.
And so when those product analysts are working with the product teams, I mean, you've already covered that, they get pulled in when designs are ready to think about the KPIs and all that stuff.
Whose initiative is that?
Does that happen organically because the analysts sit in on a feature kickoff planning meeting?
Or are the product managers proactive and pulling them in?
Are the product analysts proactive and fetching those information? Which way does this tilt?
Elena: Right now, so they just work as a team.
They meet weekly, so product analyst knows what's going on at any point in time.
And it can be either way, sometimes the product manager would suggest some things.
Sometimes product analyst would suggest that, "Hey, it's time for me to do something."
Stefania: Nice.
Elena: Yeah. So we try to move more and more in that direction, where product analysts are own their scope and they can make a call for when it's time for them to work on something.
Stefania: That's amazing.
That sounds like there is a really strong mutual respect between these two groups, between the product managers and the product analyst.
Elena: Yeah, which I had to step away from a service center notion where, everyone needs to submit the ticket, but more so working in a fully embedded way where product analysts are really assuming some sort of a leadership role within the scope of work that they're supporting.
Stefania: Yeah, exactly.
And that brings me to a follow-up question of that, because you mentioned earlier, when you were talking about your journey for building up the product analytics division and what really helped there was that they were already data-driven and that was one of the things that helped drive your authority to not just have the product analyst teams just be the team that supports the tooling, but also are proactive.
Can you talk a little bit about those two options? What was the dystopian world?
Elena: Yeah.
I think the alternative is that sort of a service center situation where there is a Jira board and the product manager, when they need something, they would submit the ticket and then the analyst would work on it.
I find that it makes sense for some smaller things that there's very specific numbers that need to be pulled or very specific chart that needs to be built.
But I feel like that didn't give the space for analysts to explore the data. I feel like the most useful insights actually come from that generative research, as opposed to just checking off boxes and building some dashboard, right?
So I wanted to empower my analysts with providing everyone the capacity and the incentive to be proactive in their thinking, in their assessment of the ecosystem, how the user experience works, what the holistic platform experience is depending on their focus area.
And with that, they also need to build the subject matter expertise so that they're not just thinking narrowly about what they see in the data, but they also ingest the context, the business context.
They ingest the qualitative research. And really the only way to do it is for them to be continuously embedded on with a group of people that are constantly thinking about a specific initiative or user experience area. And that gives an analyst a bit more space to generate ideas, a bit more ownership that is also important, depending on the person.
Some people are motivated by it, some not, but I think for those who are, it's really important to give that option as well.
And then also let them, sometimes just sit there in meetings where they would build some subject matter expertise because relying on product managers to always make a call to invite a product analyst somewhere, or to always know how to formulate the requirements, it produces results, but I feel like they're still suboptimal.
And we've definitely found that, depending on the product manager, depending on the situation, sometimes we would get a ticket before the embedded times, we'll get the ticket that would say, "Hey, I need these 20 things."
And we're like, "Why? Are you sure that this very specific metric is really the right metric for this? Can we take a step back and talk about what is the actual question you're trying to answer?"
And then let me as a person who had been looking at that data, figure out what is the right metric.
So it's like figuring that gentle balance between not necessarily saying that, "Hey, I know everything. I know better than you."
But more so working collaboratively with, "Hey, let me try to just use my past experience and you teach me about the problem."
Right? "And about your thinking."
And I will use my data specific expertise to figure out, what's the best way to answer it because as a product manager, you probably don't have all that background than the past day, the past trend.
So sometimes you might just not think about a specific thing that would be helpful or a specific way to answer that question.
So yeah, I just find that that works a bit more efficiently.
There's definitely still something to figure out just more from a process perspective because obviously going to too many meetings for analysts is also not good because we need a lot of focus time.
So figuring out how to balance being in the important meetings and reading important documents, but also not being overloaded with just a lot of meetings for the sake of attending everything that happens at their work stream.
So it's still figuring out.
Stefania: This is really good insight.
The gentle balance between you teach me about the problem and I will help you with my past data expertise.
I really love that. That's a good framing. How do you find the right people for that? How do you hire for these roles?
Elena: Yeah, definitely. That's a really good question.
I think we try to balance in our hiring process assessing both high technical skills because obviously it's important to be able to code, you knowing the statistics really well, making sure you can define the right metrics, you know how to assess experiments and have really good baseline but something that we are really trying to look at during the hiring process is, the problem formulation and synthesis, those two buckets of technical skills that are a bit less clear cut as just, "Hey, your query is right or your query is wrong."
But one thing that we find useful is doing a take home assignment, which maybe I would love to figure out the better way, because I know that take-homes can be annoying.
They take up your time if you have kids or something, it's so hard to find it.
I'm very, very well aware of all the implications of it.
We're trying to give folks some space instead of grilling them throughout multiple code pairs and white boarding sessions, we try to give some space to breakdown a problem, where we give them a prompt or the dataset around some hypothetical feature launch and have them think through, how would they think about defining KPIs?
How would they think about the user experience, which is super important product as well?
You can't just treat numbers as data points, right?
Always when you're suggesting implementing stuff, you had to think about how that would affect user experience.
And then also being able to put that together in a story that you would present to a product manager because one thing is presenting to your analytics peers, where you can present pieces of code and this and that and everyone's going to understand you, but you would not be providing the full value as a product analyst, if you could synthesize it in the way that the non-technical person would be able to understand the whole story from the problem to your recommendations, I guess, or thought starter.
So that's how we try to approach that.
We also do some behavioral stuff around just being able to collaborate effectively with your teammates and with our product managers.
But I find that the take home really helps to distinguish someone who would be a good fit or someone who is a bit more maybe focused on statistics or coding.
It's totally fine. Different people are motivated differently.
For someone, a better place would be where we really get to go very narrow and the specific technical skills.
Or really deep, I guess I should say, on the specific technical skills.
And as a product analyst, I find that you have to be a bit more T-shaped.
So you need to know a lot of approaches for simple dashboarding, to some ML models, to some statistical approaches and be able to assess for a specific problem what to pick based on the outcome that you're trying to provide and be able to absorb the business in the user experience context.
Stefania: Amazing. It sounds like we probably will need a follow-up episode on just the hiring because this is so interesting because it's such a new role.
There's the product analyst role and all of a sudden now I feel like just in this year, we have this role called analytics engineer, which is also now sort of trending.
Elena: I'm hiring. Please apply. I have a really manager level position for analytics engineering. It's open. Please, everyone apply.
Stefania: I will recommend to anyone listening that you should work with Elena.
It's been inspiring to talk to her throughout everything that you're doing, Elena really.
So it's a huge opportunity.
Anyone who's looking to become an analytics engineer, I would say, even if you haven't been done an analytics engineer before, because this is just such a new role, you probably have not had that title before.
Elena: Yeah, definitely. It's definitely not about the title, but more about the combination of skills.
Stefania: Exactly. So this is super helpful and I know it's going to be super helpful for anyone who's listening.
So go ahead, check out the Peloton job description for analytics engineer and join this amazing person on a journey for better product analytics.
We are running out of time here but I definitely do want to hear you talk a little bit about your types of tools that you have in your data stack.
Elena: Yeah, definitely. So we do have a CDP or what have you, the data collection platform.
A third party that enables the event tracking in the first place.
And that tool funnels that data both into our product analytics SaaS tool, as well as other destinations, such as data warehouse, data lakes and things like that.
So we do rely on the product analytics SaaS vendor for the trend assessments and feature launches, some experiment assessments that are pretty straightforward and can be out of box T-test, Z-test or whatever.
So that's definitely saves us a ton of time and helps us pretty easily track feature adoption, easily share those numbers with cross-functional stakeholders and empower them to also do their own trend analysis.
And then the data goes into data warehouse and data lake. So we use that both for official reporting given that, would want raw data sometimes just not clean enough.
So there's definitely some additional scripts that are required to bring the data into official reporting shape.
And we also use that raw data from data lake to do a deep dive analysis where a SaaS tool might not just be powerful enough.
So for that, we just use Jupyter Notebooks. Pretty vanilla there. Yeah, Jupyter Notebooks.
So do various types of deep dive, some ML, some of the stuff a bit more exploratory.
We do have an-- decision on the team, who's a PhD in economics and he does a bit more methodology refinements and ingesting a lot of the research literature and definitely relies a lot on that ability to do more complex analysis that would not be available out the books anywhere.
Stefania: Yeah, exactly. So I think I want to wrap this up by some knowledge sharing.
What would you say is the first thing teams should do to get their analytics right?
Elena: Oh man. It's hard to just say one thing.
I think establishing a culture around key data capture is really important.
But that's not a one thing, right? There are multiple things involved to get that right.
So I would say it is important to have someone on the team who would be able to own that and champion that, might not be a traditional maybe data scientist in a sense but there could be someone who has a bit of a functional experience maybe in product or in a specific business area that's relevant, but also has a pretty good hands-on experience in analytics.
So I would say find a champion.
One of the first tasks for them would be to get that data capture part right because without having it, it's just going to be ad hoc here, ad hoc there and it's better to get ahead of it when you're still small and have some sort of principles established around it.
And once, there is some clean data capture happening, figure out the main things that you need to be measuring based on your business model, your product model.
There are a lot of books, really good books around that such as Lean Analytics, for example, a ton of materials on re-foraged, a ton of materials on Amplitude.
Amplitude has some really good handbooks that are available to everyone to have to be their client.
So that would help you to refine your strategy around the main metrics that you should be capturing and establishing all of that in a good concise way and start looking at it weekly to see the movements.
And I think all of that combined would give folks enough inspiration and understanding around the importance of incorporating the data and the decision making.
Stefania: I love that. Thanks for sharing.
Any one thing that you wish more people knew about data and product?
Elena: Yeah. I think part of it, I kind of touched upon it a little bit is that, there is a lot of desire to say, "Hey, this one specific thing that I'm launching is going to increase the revenue or something."
But I think there's more to it than that.
There's more about figuring out how things work in combination and making sure that you understand the user personas that you have on your platform and figuring out the why behind the things that you're building and it's not always going to be that it's going to increase revenue or it's going to reduce churn or something.
So figuring out that chain of events that leads to user engagement, user satisfaction and that leads to better engagement retention, that leads to better paid retention.
Similarly for e-comm flows, there could be a similar thing that drives repeat purchases or intent that's not necessarily about being able to affect the revenue directly right away with each and every release.
Stefania: I love it.
Thank you so much Elena for sharing all of this amazing knowledge with us on The Right Track.
I look forward to one day having you on chapter two, but for now thank you.
Elena: Thank you for having me. It was definitely super fun to geek out about product analytics things.
Subscribe to Heavybit Updates
You don’t have to build on your own. We help you stay ahead with the hottest resources, latest product updates, and top job opportunities from the community. Don’t miss out—subscribe now.
Content from the Library
O11ycast Ep. #78, Exploring OTTL with Tyler Helmuth and Evan Bradley
Episode 78 of o11ycast examines the world of OpenTelemetry Transformation Language (OTTL) with Tyler Helmuth and Evan Bradley,...
O11ycast Ep. #76, Managing 200-Armed Agents with Andrew Keller
In episode 76 of o11ycast, Jessica Kerr and Martin Thwaites speak with Andrew Keller, Principal Engineer at ObservIQ, about the...
Machine Learning Model Monitoring: What to Do In Production
Machine learning model monitoring is the process of continuously tracking and evaluating the performance of a machine learning...